The Atlantic - Technology
The Atlantic's technology section provides deep insights into the tech giants, social media trends, and the digital culture shaping our world.
It’s a rare thing to shoot yourself in the foot and win a marathon. For years, Elon Musk has managed to do something like that with Tesla, achieving monumental success in spite of a series of self-inflicted disasters. There was the time he heavily promoted the company’s automated factory, only to later admit that its “crazy, complex network of conveyor belts” had thrown production of the Model 3 off track; and the time a tweet led him to be sued for fraud by the Securities and Exchange Commission; and the time he said that the Tesla team had “dug our own grave” with the massively delayed and overhyped Cybertruck. Tesla is nonetheless the most valuable car company in the world by a wide margin.
But luck runs out. Yesterday evening, Tesla reported first-quarter earnings for 2025, and they were abysmal: Profits dropped 71 percent from the same time last year. Musk sounded bitter on the call with investors that followed, blaming the company’s misfortune on protesters who have raged at Tesla dealerships around the world over his role running DOGE and his ardent support of far-right politicians. “The protests that you’ll see out there, they’re very organized. They’re paid for,” he said, without evidence.
Then he pivoted. Although Musk described DOGE as “critical work,” he said that his “time allocation” there “will drop significantly” next month, down to just one or two days a week. He’s taking a big step back from politics and returning the bulk of his attention to Tesla, as even his most enthusiastic supporters have begged him to do. (Tesla did not immediately return a request for comment.)
One bad quarter won’t doom Tesla, but it’s unclear how, exactly, the company can move forward from here. Arguably, its biggest and most immediate problem is that electric-vehicle fans in America, who tend to lean left politically, do not want to buy Musk’s cars anymore. The so-called Tesla Takedown protests have given people who feel helpless and angry about President Donald Trump’s policies a tangible place to direct their anger. Because Musk was also the Trump campaign’s biggest financier, those protesters saw a Tesla boycott as one of the best ways to hit back. The fact that these demonstrations were the first thing Musk brought up on the earnings call speaks volumes about how rattled he must be; Tesla purchases have been down considerably this year in the U.S., even as EV sales keep rising.
And while some people in Europe may believe they do not have much cause to care about DOGE, they do care that Musk has been promoting far-right political actors, most notably Germany’s Alternative for Germany party. That seems to be having a palpable impact on Tesla’s sales; they’ve been tanking by double-digits across Europe.
Buyers are turning to other car brands for their electric-powered driving needs, and those brands are happy to take their business. Tesla may have effectively created the modern EV sector, but the competition is catching up. In the U.S. alone, several car companies now offer electric options with more range, better features, and lower prices than Tesla. A long-awaited cheaper new Tesla could bring in more buyers, but there’s been little fanfare around it, perhaps because Musk is preoccupied with autonomous taxis and self-driving cars; a new “robotaxi” service is supposedly launching in June, in Austin. Yet any self-driving-technology investment depends on Tesla’s ability to sell cars right now to finance those dreams, and that’s where Tesla is likely to continue to have trouble.
[Read: The great Tesla sell-off]
Finally, there’s the bigger problem of China. Musk’s company effectively showed that country how to make modern EVs, and although Teslas still sell well enough there, Musk is up against dozens of new Tesla-like companies that have taken his ideas and run wild. Electric cars in China can be had with more advanced features than what Tesla offers, faster charging times, and more advanced approaches to automated driving. (Case in point: I am writing this story in Shanghai, from the passenger’s seat of an EV that can swap its depleted battery for a fresh one in mere minutes.)
At most companies, it’d be long past time to show the CEO the door. But Tesla’s stock price is inextricably linked to Musk and his onetime image as Silicon Valley’s greatest living genius. Even if Musk were to move on, it’s unclear whether Tesla as a brand could recover, Robby DeGraff, an analyst at the research firm AutoPacific, told me. “I’m genuinely not convinced removing him would be enough,” DeGraff said. “I do believe the potential is there for the brand to steer itself around with exciting, quality, innovative products. But there’s a colossal amount of repair work that needs to be done behind the scenes first.”
Unfortunately for Tesla, the great disruptor of the automotive industry is beginning to feel a lot like a “legacy” car company, struggling to figure out what’s next and getting lapped by newcomers. The competition has the advantage of not being inextricably tied to a boss who’s made the brand so toxic that people would rather go to his dealerships to wave angry signs than to buy cars. If Tesla’s future rests on left-leaning EV fans forgiving Musk for backing Trump, boosting the AfD party in Germany, and gleefully putting hundreds of thousands of federal employees out of work, then Musk may find himself longing for the days when his biggest problem was building a wild-looking stainless-steel truck.
Updated at 10:26 a.m. ET on April 23, 2025
Everywhere I look on social media, disembodied heads float in front of legal documents, narrating them line by line. Sometimes they linger on a specific sentence. Mostly they just read and read.
One content creator, who posts videos under the username I’m Not a Lawyer But, recently made a seven-minute TikTok in which she highlighted the important sentences from Drake’s 81-page defamation complaint against Universal Music Group. Another described herself in a recent video as “literally reading through the receipts of Justin Baldoni’s 179-page lawsuit,” referring to one stage of a complicated legal battle between Baldoni and his It Ends With Us co-star, Blake Lively, which is the hot legal case of the moment. The threads of this conflict are too knotted for me to fully untangle here, but the dispute began in December with Lively accusing Baldoni of inappropriate on-set behavior and of a secret social-media campaign against her. It became chaotic—and ripe for play-by-play commentary—in February when Baldoni, who has denied Lively’s allegations, launched a website with the URL thelawsuit.info to tell his side of the story.
The creators I’m seeing have loyal, long-term audiences and sell T-shirts and water bottles emblazoned with obscure references. They go by names such as Lawyer You Know and Legal Bytes (“Explaining the law one bite at a time!”) and sometimes appeal to expertise, usually by proving that they are actual attorneys. For some, though, their bona fides are looser: “I’m not an attorney, but I was raised by attorneys,” one creator said in a recent video.
The popularity of this material—a kind of lawyerly ASMR—has surprised even some of the people who make it. “It seems odd to us,” Stewart Albertson, one half of the podcast Ask 2 Lawyers, told me. He and his co-host, Keith Davidson, in fact are lawyers, and sometimes get 100,000 views on lengthy videos in which they go through a legal motion line by line. They’ve asked the audience if they should go faster and skip over some things. The commenters say no. They love monotony and minutiae. “People talk about, ‘Oh, I could go to sleep to these guys,’” Albertson told me. These are words of affection. He and Davidson know that because the commenters have also asked them to make Ask 2 Lawyers merch (specifically, they would like coffee mugs that say “12(b)(6)” on them, in reference to a type of legal motion filed by Blake Lively).
Albertson and Davidson spent more than a decade making marketing videos explaining trust and estate law, their firm’s speciality. Now they mostly make what they call educational content in which they explain high-profile legal disputes. They started with a series on a dramatic saga involving Tom Girardi, the ex of one of the women on Bravo’s Real Housewives of Beverly Hills. (Girardi was famous for his heroism in the Erin Brockovich case; he is now infamous for having been convicted of embezzlement and wire fraud, as well as for the way his criminal activity affected the fascinating women of RHOBH.) Although the topics are salacious, the two lawyers’ videos are, with all due respect, breathtakingly boring. “You know, we’re kind of bringing calm to chaos,” Albertson said. “Maybe that’s what speaks to people.”
[Read: What the JFK file dump actually revealed]
That’s part of it, but it’s also the simple allure of stacks of papers. Markos Bitsakakis, a 25-year-old TikTok creator from Toronto who also runs an herbal-honey company, sometimes gets 1 million likes on a video in which he flips through huge dossiers the entire time he is talking. (He has so far published 12 installments of a series titled “The Downfall of Blake Lively & Ryan Reynolds.”) He’ll explain to the viewers that he’s just spent nine hours with one document, or that he’s recording at two in the morning because he has been reading for so long. His followers joke that a printer must, as the saying goes, hate to see him coming. “I have like a million files,” he told me. “Mentally, I’m 45 years old,” he added, to explain why he prefers hard copies to PDFs. The way that he dramatizes his work situates him in an online tradition of romanticizing studying and research (especially when they’re done alone). “Lucky for you guys I never travel anywhere without my files,” he said at the start of a video he recorded while on a trip.
Celebrity lawsuits have always been followed in detail by tabloids and gossip bloggers, and our reality-TV culture has been fascinated for some time with the idea of “receipts”—proof of malfeasance, often in the form of text messages or screenshots. But this is newer. Amateur legal analysis is now a whole category of content creation, and thick, formal documents are the influencers’ bread and butter.
These creators often present a very internet-age populist message alongside their analysis—many of the videos allow for the possibility that anyone can become an expert simply by having the commitment to read and keep reading the things that they are able to access freely online. Another of their stated commitments is to the notion of transparency, which helps explain why many of the same creators have expressed an interest in the National Archives’ recent dump of files pertaining to the John F. Kennedy assassination. Of course, some of the draw is gossip. But to a significant degree, I think the draw really is files.
Files are never-ending stories—or at least they can feel that way when a case drags on, providing a new flurry of paperwork week after week. Katy Hoffman, a 32-year-old in Kansas City, follows CourtListener and PacerMonitor for updates on the Baldoni-Lively case and told me that this is effectively her unwinding ritual. Instead of watching TV or scrolling Instagram at night, she’ll read whatever is new. “I try to maintain a good balance,” she said. That last hour of the day that everyone spends doing something pointless is the one she spends on this, she told me. (She also makes her own videos sometimes, though her audience is quite small.)
Similarly, Julie Urquhart, a 49-year-old teacher from New Brunswick, Canada, told me that she spends much of her free time reading court documents and then making short TikTok videos about them. “I’ve read everything you can on this case,” she told me. “All the lawsuits, multiple times.” She loved working at a radio station in college, so this is a hobby that can satisfy the same impulse to research and then broadcast, even if to a tiny audience. As with many other creators, Urquhart has recently focused on the Baldoni lawsuit, and it has caused her some grief: She takes Lively’s side, which she says has made her videos less popular and led people to be furious with her in the comments.
Here is where content about files becomes less fun. Particularly if you look at the comment sections, you’ll see a lot of vitriol against Lively—visible in much the same way as was vitriol against Amber Heard during the Johnny Depp trial or Evan Rachel Wood during her dispute with Marilyn Manson. Most of the creators I spoke with insisted that misogyny is not a factor in the success of their videos or in their own presentation of the facts, but this is not totally convincing. In 2020, I wrote about the rise of conspiracy theories about celebrities allegedly faking their pregnancies, which were transparently the product of resentment toward famous people and other elites, women especially, and I see quite a bit of that here as well. Commenters often express that they are tired of being “manipulated” by such people.
[Read: How a fake baby is born]
This is not to say that content about the Baldoni-Lively case is inherently toxic. In fact, it’s likely that these lawsuit influencers have had success with it because it’s fairly middle-of-the-road: mysterious, but not acutely morbid or upsetting like true crime can be. As Bitsakakis put it, the topic is “dramatic and exciting and salacious, but it isn’t necessarily as serious as nuclear war.” He thinks that he’s been rewarded by the TikTok algorithm for hitting that sweet spot. There are other legal topics he’d like to “investigate,” such as the Luigi Mangione case and the Sean “Diddy” Combs allegations, but those things may be just a little too dark to be pushed out into the main feed by the powerful recommendation engine. (For the same reason, many TikTok creators reference Blake Lively’s claims by saying “SH” rather than “sexual harassment.”)
The broad interest in this case—and its many files—has made for some strange bedfellows. Recently, New York magazine published a story on self-described liberals winding up on the YouTube page of the right-wing influencer Candace Owens, who dabbles in conspiracy theories and is currently working on a YouTube series trying to prove that Brigitte Macron was born a man, because they’re impressed by her ample Baldoni-Lively coverage. Owens is explicitly anti-#MeToo and sells Anti-Feminist baseball caps in her merch store, but viewers who don’t share her politics reportedly still enjoy watching her go through lots of legal documents and show her work. “I read them myself,” she told me. “I sit down with a pen, mark things up, use stickies, little different-colored stickies if I have questions, like for my lawyer, and he’ll explain things to me.”
These videos, as well as ones in which Owens speculates about whether Ryan Reynolds is gay, get millions of views. When I told her that I found it odd that so many people were interested in what amounted to a workplace dispute, she rejected the characterization. It was bigger than that, because it represented a shift in the way that people consume information, she told me. They’re more trusting now of online content creators who will present everything—all of the documents—than they are of traditional journalists, whom they perceive as being inappropriately possessive and aloof. “I’m very excited to see that both the left and the right are agreeing, finally, that we should really be removing a lot of the authority that we gave to the mainstream media to tell us what to think about other people,” she said. “I think it’s great. I think it’s brilliant.”
This was a sentiment I heard frequently from creators and saw often in the comments on their videos—people expressed a vaguely paranoid feeling that raw information is being deliberately kept away from them by reporters who hoard or hide it so that they can maintain their own power. It’s not an accurate understanding of the current state of journalism, but it is a popular one, and it helps explain the allure of reams of court documents. Davidson told me that the audience for Ask 2 Lawyers appreciates the granular level of detail that he and Albertson provide because it indicates that they are intelligent and curious enough to understand.
“We don’t talk down to them,” he said. “We don’t try to make them feel like, We know and you don’t. We’re here to give you the information, and you make up your own mind on it.” Clearly, people are really, really into that.
This article previously referred to Tom Girardi as the ex-husband of a cast member on The Real Housewives of Beverly Hills. The two are separated but not divorced.
There are really two OpenAIs. One is the creator of world-bending machines—the start-up that unleashed ChatGPT and in turn the generative-AI boom, surging toward an unrecognizable future with the rest of the tech industry in tow. This is the OpenAI that promises to eventually bring about “superintelligent” programs that exceed humanity’s capabilities.
The other OpenAI is simply a business. This is the company that is reportedly working on a social network and considering an expansion into hardware; it is the company that offers user-experience updates to ChatGPT, such as an “image library” feature announced last week and the new ability to “reference” past chats to provide personalized responses. You could think of this OpenAI as yet another tech company following in the footsteps of Meta, Apple, and Google—eager not just to inspire users with new discoveries, but to keep them locked into a lineup of endlessly iterating products.
The most powerful tech companies succeed not simply by the virtues of their individual software and gadgets, but by building ecosystems of connected services. Having an iPhone and a MacBook makes it very convenient to use iCloud storage and iMessage and Apple Pay, and very annoying if a family member has a Samsung smartphone or if you ever decide to switch to a Windows PC. Google Search, Drive, Chrome, and Android devices form a similar walled garden, so much so that federal attorneys have asked a court to force the company to sell Chrome as a remedy to an antitrust violation. But compared with computers or even web browsers, chatbots are very easy to switch among—just open a new tab and type in a different URL. That makes the challenge somewhat greater for AI start-ups. Google and Apple already have product ecosystems to slide AI into; OpenAI does not.
OpenAI CEO Sam Altman recently claimed that his company’s products have some 800 million weekly users—approximately a tenth of the world’s population. But even if OpenAI had only half that number of users, that would be a lot of people to risk losing to Anthropic, Google, and the unending torrent of new AI start-ups. As other tech companies have demonstrated, collecting data from users—images, conversations, purchases, friendships—and building products around that information is a good way to keep them locked in. Even if a competing chatbot is “smarter,” the ability to draw on previous conversations could make parting ways with ChatGPT much harder. This also helps explain why OpenAI is giving college students two months of free access to a premium tier of ChatGPT, seeding the ground for long-term loyalty. (This follows a familiar pattern for tech companies: Hulu used to be free, Gmail used to regularly increase its free storage, and eons ago, YouTube didn’t serve ads.) Notably, OpenAI has recently hired executives from Meta, Twitter, Uber, and NextDoor to advance its commercial operations.
OpenAI’s two identities—groundbreaking AI lab and archetypal tech firm—do not necessarily conflict. The company has said that commercialization benefits AI development, and that offering AI models as consumer products is an important way to get people accustomed to the technology, test its limitations in the real world, and encourage deliberation over how it should and shouldn’t be used. Presenting AI in an intuitive, conversational form, rather than promoting a major leap in an algorithm’s “intelligence” or capabilities, is precisely what made ChatGPT a hit. If the idea is to make AI that “benefits all of humanity,” as OpenAI professes in its charter, then sharing these purported benefits now both makes sense and creates an economic incentive to train better and more reliable AI models. Increased revenue, in turn, can sustain the development of those future, improved models.
Then again, OpenAI has gradually transitioned from a nonprofit to a more and more profit-oriented corporate structure: Using generative-AI technology to magically discover new drugs is a nice idea, but eventually the company will need to start making money from everyday users to keep the lights on. (OpenAI lost well over $1 billion last year.) A spokesperson for OpenAI, which has a corporate partnership with The Atlantic, wrote over email that “competition is good for users and US innovation. Anyone can use ChatGPT from any browser,” and that “developers remain free to switch to competing models whenever they choose.”
[Read: The Gen Z lifestyle subsidy]
Anthropic and Meta have both taken alternative approaches to bringing their models to internet users. The former recently offered the ability to integrate its chatbot Claude into Gmail, Google Docs, and Google Calendar—gaining a foothold in an existing tech ecosystem rather than building anew. (OpenAI seemed to be testing this strategy last year by partnering with Apple to incorporate ChatGPT directly into Apple Intelligence, but this requires a bit of setup on the user’s part—and Apple’s AI efforts have been broadly perceived as disappointing.) Meta, meanwhile, has made its Llama AI models free to download and modify—angling to make Llama a standard for software engineers. Altman has said OpenAI will release a similarly open model later this year; apparently the start-up wants to both wall off its garden and make its AI models the foundation for everyone else, too.
From this vantage, generative AI appears less revolutionary and more like all the previous websites, platforms, and gadgets fighting to grab your attention and never let it go. The mountains of data collected through chatbot interactions may fuel more personalized and precisely targeted services and advertisements. Dependence on smartphones and smartwatches could breed dependence on AI, and vice versa. And there is other shared DNA. Social-media platforms relied on poorly compensated content-moderation work to screen out harmful and abusive posts, exposing workers to horrendous media in order for the products to be palatable to the widest audience possible. OpenAI and other AI companies have relied on the same type of labor to develop their training data sets. Should OpenAI really launch a social-media website or hardware device, this lineage will become explicit. That there are two OpenAIs is now clear. But it remains uncertain which is the alter ego.
Finals season looks different this year. Across college campuses, students are slogging their way through exams with all-nighters and lots of caffeine, just as they always have. But they’re also getting more help from AI than ever before. Through the end of May, OpenAI is offering students two months of free access to ChatGPT Plus, which normally costs $20 a month. It’s a compelling deal for students who want help cramming—or cheating—their way through finals: Rather than firing up the free version of ChatGPT to outsource essay writing or work through a practice chemistry exam, students are now able to access the company’s most advanced models, as well as its “deep research” tool, which can quickly synthesize hundreds of digital sources into analytical reports.
The OpenAI deal is just one of many such AI promotions going around campuses. In recent months, Anthropic, xAI, Google, and Perplexity have also offered students free or significantly discounted versions of their paid chatbots. Some of the campaigns aren’t exactly subtle: “Good luck with finals,” an xAI employee recently wrote alongside details about the company’s deal. Even before the current wave of promotions, college students had established themselves as AI’s power users. “More than any other use case, more than any other kind of user, college-aged young adults in the US are embracing ChatGPT,” the vice president of education at OpenAI noted in a February report. Gen Z is using the technology to help with more than schoolwork; some people are integrating AI into their lives in more fundamental ways: creating personalized workout plans, generating grocery lists, and asking chatbots for romantic advice.
AI companies’ giveaways are helping further woo these young users, who are unlikely to shell out hundreds of dollars a year to test out the most advanced AI products. Maybe all of this sounds familiar. It’s reminiscent of the 2010s, when a generation of start-ups fought to win users over by offering cheap access to their services. These companies especially targeted young, well-to-do, urban Millennials. For suspiciously low prices, you could start your day with pilates booked via ClassPass, order lunch with DoorDash, and Lyft to meet your friend for happy hour across town. (On Uber, for instance, prices nearly doubled from 2018 to 2021, according to one analysis). These companies, alongside countless others, created what came to be known as the “Millennial lifestyle subsidy.” Now something similar is playing out with AI. Call it the Gen Z lifestyle subsidy. Instead of cheap Ubers and subsidized pizza delivery, today’s college students get free SuperGrok.
AI companies are going to great lengths to chase students. Anthropic, for example, recently started a “campus ambassadors” program to help boost interest; an early promotion offered students at select schools a year’s worth of access to a premium version of Claude, Anthropic’s AI assistant, for only $1 a month. One ambassador, Josefina Albert, a current senior at the University of Washington, told me that she shared the deal with her classmates, and even reached out to professors to see if they might be willing to promote the offer in their classes. “Most were pretty hesitant,” she told me, “which is understandable.”
The current discounts come at a cost. There are roughly 20 million postsecondary students in the U.S. Say just 1 percent of them take advantage of free ChatGPT Plus for the next two months. The start-up would effectively be giving a handout to students that is worth some $8 million. In Silicon Valley, $8 million is a rounding error. But many students are likely taking advantage of multiple such deals all at once. And, more to the point, AI companies are footing the bill for more than just college students. All of the major AI companies offer free versions of their products despite the fact that the technology itself isn’t free. Every time you type a message into a chatbot, someone somewhere is paying for the cost of processing and generating a response. These costs add up: OpenAI has more than half a billion weekly users, and only a fraction of them are paid subscribers. Just last week, Sam Altman, the start-up’s CEO, suggested that his company spends tens of millions of dollars processing “please” and “thank you” messages from users. Tack on the cost of training these models, which could be as much as $1 billion for the most advanced versions, and the price tag becomes even more substantial. (The Atlantic recently entered into a corporate partnership with OpenAI.)
These costs matter because, despite AI start-ups’ enormous valuations (OpenAI was just valued at $300 billion), they are wildly unprofitable. In January, Altman said that OpenAI was actually losing money on its $200-a-month “Pro” subscription. This year, the company is reportedly projected to burn nearly $7 billion; in a few years, that number could grow to as much as $20 billion. Normally, losing so much money is not a good business model. But OpenAI and its competitors are able to focus on acquiring new users because they have raised unprecedented sums from investors. As my colleague Matteo Wong explained last summer, Silicon Valley has undertaken a trillion-dollar leap of faith, on track to spend more on AI than what NASA spent on the Apollo space missions, with the hope that eventually the investments will pay off.
The Millennial lifestyle subsidy was also fueled by extreme amounts of cash. Ride-hailing businesses such as Uber and Lyft scooped up customers even as they famously bled money for years. At one point in 2015, Uber was offering carpool rides anywhere in San Francisco for just $5 while simultaneously burning $1 million a week. At times, the economics were shockingly flimsy. In 2019, the owner of a Kansas-based pizzeria noticed that his restaurant had been added to DoorDash without his doing. Stranger still, a pizza he sold for $24 was priced at $16 on DoorDash, yet the company was paying him the full price. In its quest for growth, the food-delivery start-up had reportedly scraped his restaurant’s menu, slapped it on their app, and was offering his pie at heavy discount. (Naturally, the pizzeria owner started ordering his own pizzas through DoorDash—at a profit.)
These deals didn’t last forever, and neither can free AI. The Millennial lifestyle subsidy eventually came crashing down as the cheap money dried up. Investors that had for so long allowed these start-ups to offer services at artificially deflated prices wanted returns. So companies were forced to raise prices, and not all of them survived.
If they want to succeed, AI companies will also eventually have to deliver profits to their investors. Over time, the underlying technology will get cheaper: Despite companies’ growing bills, technical improvements are already increasing efficiency and driving down certain expenses. Start-ups could also raise revenue through ultra-premium enterprise offerings. OpenAI is reportedly considering selling “PhD-level research agents” at $20,000 a month. But it’s unlikely that companies such as OpenAI will allow hundreds of millions of free users to coast along indefinitely. Perhaps that’s why the start-up is currently working on both search and social media; Silicon Valley has spent the past two decades essentially perfecting the business models for both.
Today’s giveaways put OpenAI and companies like it only further in the red for now, but maybe not in the long run. After all, Millennials became accustomed to Uber and Lyft, and have stuck with ride-hailing apps even as prices have increased since the start of the pandemic. As students learn to write essays and program computers with the help of AI, they are becoming dependent on the technology. If AI companies can hook young people on their tools now, they may be able to rely on these users to pay up in the future.
Some young people are already hooked. In OpenAI’s recent report on college students’ ChatGPT adoption, the most popular category of non-education or career-related usage was “relationship advice.” In conversations with several younger users, I heard about people who are using AI for color-matching cosmetics, generating customized grocery lists based on budget and dietary preferences, creating personalized audio meditations and half-marathon training routines, and seeking advice on their plant care. When I spoke with Jaidyn-Marie Gambrell, a 22-year-old based in Atlanta, she was in the parking lot at McDonald’s and had just consulted ChatGPT on her order. “I went on ChatGPT and I’m like, ‘Hey girl,’” she said. “‘Do you think it’d be smart for me to get a McChicken?’” The chatbot, which she has programmed to remember her dietary and fitness goals, advised against it. But if she really wanted a sandwich, ChatGPT suggested, she should order the McChicken with no mayo, extra lettuce, tomatoes, and no fries. So that’s what she got.
The Gen Z lifestyle subsidy isn’t entirely like its Millennial predecessor. Uber was appealing because using an app to instantly summon a car is much easier than chasing down a cab. Ride-hailing apps were destructive for the taxi business, but for most users, they were just convenient. Today’s chatbots also sell convenience by expediting essay writing and meal planning, but the technology’s impact could be even more destabilizing. College students currently signing up for free ChatGPT Plus ahead of finals season might be taking exams intended to prepare them for jobs that the very same AI companies suggest will soon evaporate. Even the most active young users I spoke with had mixed feelings about the technology. Some people “are skating through college because of ChatGPT,” Gambrell told me. “That level of convenience, I think it can be abused.” When companies offer handouts, people tend to take them. Eventually, though, someone has to pay up.
Like countless others who have left their hometown to live a sinful, secular life in a fantastic American city, I no longer actively practice Christianity. But a few times a year, my upbringing whispers to me across space and time, and I have to listen. The sound is loudest at Easter, which, aside from being the most important Christian holiday, is also the most fun.
I could talk about Easter all day. The daffodils, the brunch. The color scheme, the smell of grass, the annual screening of VeggieTales: An Easter Carol, which is the same story as Charles Dickens’s A Christmas Carol, except that it’s set at Easter and all the characters are vegetables who work in a factory (the Scrooge character is a zucchini). And most of all, the Easter eggs! Of all the seasonal crafts, this one is the easiest (no carving) and the most satisfying (edible).
This year, because of shocking egg prices, people with online lifestyle brands—or people who aspire to have online lifestyle brands—have suggested numerous ways to keep the dyeing tradition alive without shelling out for eggs. For instance, you can dye jumbo-size marshmallows, or you can make peanut-butter eggs that you then coat in colored white chocolate. You can paint rocks. The story has been widely covered, by local TV and radio stations and even The New York Times. “Easter Eggs Are So Expensive Americans Are Dyeing Potatoes,” the Times reported (though most of the story was about one dairy farmer who’d replaced real eggs with plastic replicas for an annual Easter-egg hunt).
I don’t think many people are actually making Easter spuds. Like baking Goldfish or making breakfast cereal from scratch, dyeing potatoes seems mostly like a good idea for a video to post online. Many Instagram commenters reacted to the Easter potatoes by saying things such as “What in the great depression is this” and “These potatoes make me sad.” And yet, because I love Easter and am curious about the world, I decided to try it myself—just to see if it was somehow any fun.
[Read: I really can’t tell if you’re serious]
My local Brooklyn grocery store didn’t have the classic Paas egg-dyeing tablets, so I bought an “organic” kit that cost three times as much ($6.99) and expensed it to The Atlantic. I bought a dozen eggs ($6.49) and a bag of Yukon Gold potatoes that were light-colored enough to dye and small enough to display in a carton ($5.99), and expensed those to The Atlantic too. Then I looked online for advice on how to proceed; mostly, I wanted to know whether I should cook the potatoes before or after dyeing them. A popular homemaking blog called The Kitchn gave detailed instructions on how to dye Easter potatoes and “save some cash while flexing your creativity for the Easter Bunny this year.” The suggestions—which included soaking the potatoes in ground turmeric, shredded beets, or three cups of mashed blueberries—were not as cost-effective as promised. (Such a volume of fruit could cost north of $15.) But I did find out that I should decorate the potatoes and then cook them. Thank you!
Alone in my kitchen on a Saturday morning, I dyed six boiled eggs and six raw potatoes and used a teensy paintbrush to add squiggly lines, daisies, and other doodles, returning me to my youth as an observant Methodist who really knew her resurrection-specific hymns. The eggs came out in stunning shades of marigold, magenta, and cornflower blue. The potatoes came out sort of yellow, or sort of pink, or sort of purple, all of which you may recognize as colors that potatoes already have when you buy them at the store. I hated them.
When I painted HAPPY EASTER on one of the potatoes, it looked like a threat. When I baked them in my oven, their skins (naturally) crinkled and came somewhat unstuck from their insides. This had the effect of making them look shriveled and even more sinister. When I put them in the egg carton next to my beautiful half-dozen Easter eggs, I thought: Only a person who was lying would do this and say it was good. Without being too overwrought about it, the whole project felt like a symbol not of renewal but of the wan stupidity of our cultural moment.
[Read: The case for brain rot]
The average price for a carton of eggs last month was $6.23, which is, we all agree, a lot for eggs. But it’s not really a lot for a craft project that also serves as a cultural ritual and can also serve as breakfast, so long as you put your craft project and cultural ritual in the refrigerator. (Until recently, I’d assumed that all families eat their Easter eggs, but apparently some people put them on display in their house, after which you certainly can’t eat them.) Sure, if an egg is really too expensive, replacing it with a potato could be called ingenious. But the many deficiencies of this replacement are immediately obvious. For instance, dye doesn’t work as well on a brown potato as it does on a white egg. Potatoes are uninspiring objects—people evoke them when they want to suggest that something is lumpy, dumb, or useless. Eggs are lovely, smooth, elegant, and the subject of fine art. Eggs are revered. You can’t just swap one thing out for another because they are a similar size and weight.
I know I am being judgmental—decidedly not the point of Easter. But this insincere hack rudely assumes that children can’t tell the difference between a simple, nice thing and a more complicated, far inferior thing. I will concede only one point to the potato-dyers. As The Atlantic put it rather grotesquely in 1890, eggs symbolize “the bursting into life of a buried germ.” I have to admit that this is a pretty good way to describe tubers as well. It made me briefly consider burying my Easter potatoes in the backyard and waiting to see if they would grow into more Easter potatoes. Season of hope and all that.
[From the May 1890 issue: The Easter hare]
Instead, I ate all of them and then they were gone, which felt a lot better.
Josh Shapiro is very lucky to be alive. The Pennsylvania governor and his family escaped an arson attack in the early hours of this morning. Parts of the governor’s mansion were badly charred, including an opulent room with a piano and a chandelier where Shapiro had hosted a Passover Seder just hours earlier. Things could have been much worse. The suspect, Cody Balmer, who turned himself in, would have beaten Shapiro with a hammer if he had found him in his home, he reportedly said in an affidavit.
Balmer admitted to “harboring hatred” of Shapiro, authorities said, but his precise motives are still unclear. He reportedly expressed anti-government views and made allusions to violence on social media. He reposted an image of a Molotov cocktail with the caption “Be the light you want to see in the world.” Balmer’s mother told CBS that he has a history of mental illness. But no matter how you square it, the attack is just the latest example of political violence in the United States. Last month, a Wisconsin teenager was charged with murdering his mother and stepfather as part of a plot to try to assassinate President Donald Trump—this, of course, follows two assassination attempts targeting Trump last year. Other prominent instances of ideological violence include the murder of UnitedHealthcare CEO Brian Thompson late last year, and the time when a man broke into Nancy Pelosi’s home in 2022 and attacked her husband, Paul Pelosi, with a hammer, fracturing his skull. (He was badly hurt but survived.)
Shapiro is a Democrat, but in a rare moment of bipartisan agreement, Republicans joined Democrats in condemning the attack. President Trump said in the Oval Office today that the suspect “was probably just a wack job and certainly a thing like that cannot be allowed to happen.” Vice President J. D. Vance called the violence “disgusting,” and Attorney General Pam Bondi posted on X that she was “relieved” that Shapiro and his family are safe.
These kinds of condemnations of political violence are good. They’re also meaningless—especially when taken in the broader context of Trump’s governing style. Perhaps it’s no coincidence that since Trump first ran for office, political violence has been on the rise. When it’s useful to Trump, he praises violence and makes leveraging the threat of it endemic to his style of politics. When Montana’s then–congressional candidate (and now-governor) Greg Gianforte assaulted a reporter in 2017, Trump later said, “Any guy that can do a body slam, he is my type!” After Kyle Rittenhouse shot and killed a protester in Kenosha, Wisconsin, in the summer of 2020, he had a friendly meeting with Trump at Mar-a-Lago the next year. And during a presidential debate against Joe Biden that fall, when Trump was asked if he would rebuke the Proud Boys, a far-right organization with a history of inciting violence, he told the group to “stand back and stand by,” as though he were giving it orders. (This is also how the Proud Boys interpreted it.)
Read: A brief history of Trump’s violent remarks
Trump made his willingness to engage in political violence especially clear during the Capitol insurrection on January 6, 2021. Instead of immediately attempting to call off his rabid supporters, Trump sat on his hands as his supporters stormed the Capitol—even as members of his own party urged him to help. Despite having lost the election, Trump appeared okay with violence if it helped him maintain the presidency.
Since retaking office, Trump has appeared to continue this tradition. When Pete Hegseth, the president’s pick for secretary of defense, faced a sexual-assault accusation ahead of his confirmation vote, violence may have been the ingredient that ensured that Trump got his way. Republican Senator Thom Tillis seemed concerned that some of the allegations against Hegseth could be credible and was on track to tank his nomination. According to Vanity Fair, the FBI warned Tillis of “credible death threats” against him, which could have played a role in his decision to back down. Tillis has not said whether the death threats influenced his Hegseth vote, but his office released recordings of the threats he has received.
Other Republicans in Congress are afraid of opposing Trump because of similar potential concerns for their safety. Many have gone on the record in recent years and said as much. Mitt Romney told my colleague McKay Coppins that a fellow congressman confessed to him that he had wanted to vote for Trump’s second impeachment in 2021 but ultimately chose not to out of fear for his family’s safety. That same year, Republican Representative Peter Meijer told my colleague Tim Alberta that he witnessed a fellow member of Congress have a near breakdown over fear that Trump supporters would come for his family if he voted to certify the 2020 election results.
All of this is to say that when Trump condemns acts of political violence, it’s impossible to take him seriously. In this specific case, the attack on Shapiro served no clear benefit to Trump, which is why he was able to so quickly speak out against it. Compare that with how he’s talked about the Pelosi hammer attack, which he has used as fodder to mock the Pelosis. Trump’s relationship to political violence is the same as his relationship to anything and anyone else in his orbit: If something benefits him, it’s welcome. If not, he may dismiss it.
Nearly three months into President Donald Trump’s term, the future of American AI leadership is in jeopardy. Basically any generative-AI product you have used or heard of—ChatGPT, Claude, AlphaFold, Sora—depends on academic work or was built by university-trained researchers in the industry, and frequently both. Today’s AI boom is fueled by the use of specialized computer-graphics chips to run AI models—a technique pioneered by researchers at Stanford who received funding from the Department of Defense. All of those chatbots? They rely on a training method called “reinforcement learning,” the foundations of which were developed with National Science Foundation (NSF) grants.
“I don’t think anybody would seriously claim that these [AI breakthroughs] could have been done if the research universities in the U.S. didn’t exist at the same scale,” Rayid Ghani, a machine-learning researcher at Carnegie Mellon University, told me. But Trump and the Department of Government Efficiency have frozen, canceled, or otherwise slowed billions of dollars in grants and fired hundreds of staff from the federal agencies that have funded the nation’s pioneering academic research for decades, including the National Institutes of Health and the NSF. The administration has halted or threatened to withhold billions of dollars from premier research universities that it has accused of anti-Semitism or unwanted DEI initiatives. Graduate students are being detained by immigration agents. Universities, in turn, are issuing hiring freezes, reducing offers to graduate students, and canceling research projects.
Outwardly, Trump has positioned himself as a champion of AI. During his first week in office, he signed an executive order intended to “sustain and enhance America’s dominance in AI” and proudly announced the Stargate Project, a private venture he called “the largest AI infrastructure project, by far, in history.” He has been clear that he wants to make it as easy as possible for companies to build and deploy AI models as they wish. Trump has consulted and associated himself with leaders in the tech industry, including Elon Musk, Sam Altman, and Larry Ellison, who have in turn showered the president with praise. But generative AI is not just an industry—it is a technology dependent on progressive innovations. Despite his bravado, Trump is rapidly eroding the engine of scientific innovation in America, and thus the capacity for AI to continue to advance.
In a statement, White House Assistant Press Secretary Taylor Rogers wrote that the administration’s actions are in service of building up the economy, fighting China, and combatting “divisive DEI programs” at the nation’s universities. “While Joe Biden sat back and let China make gains in the AI space, President Trump is restoring America’s global dominance by imposing tariffs on China—which has ripped us off for far too long,” Rogers wrote. (As my colleague Damon Beres wrote earlier this week, tariffs may only hurt American technology businesses.)
Despite Trump’s aims, the United States now risks losing ground to Canada, Europe, and, indeed, China in the race for AI and other technological innovation. In a Nature poll of American scientists last month, 75 percent of respondents—some 1,200 researchers—said they were considering leaving the country. New scientific and technological developments may occur elsewhere, slow down, or simply stop altogether.
Silicon Valley, despite frequently operating at odds with federal oversight, could not have come up with some of its most valuable ideas, or trained the research scientists who did, without the government’s assistance. Federally supported research and researchers, conducted and trained at American universities, helped make possible the internet, Google Search, ChatGPT, AlphaFold, and the entire AI boom (to say nothing of vaccines, electric vehicles, and weather forecasting). This fact is not lost on two of the “godfathers” of AI, Yann LeCun and Geoffrey Hinton, both of whom have lambasted the administration’s assault on science funding.
[Read: Throw Elon Musk out of the Royal Society]
“Curiosity-driven research is what allows us to explore directions that venture capital or research labs in industry would not, and should not, explore,” Alex Dimakis, a computer scientist at UC Berkeley and a co-founder of the AI start-up Bespoke Labs, told me. For example, AlphaFold—a series of AI models that predict the 3-D structure of proteins—was designed at Google but trained on an enormous collection of protein data that, for decades, has been maintained with funding from the NIH, the NSF, and other federal agencies, as well as similar government support in Europe and Japan; AlphaFold’s creators recently won a Nobel Prize. “All of these innovations, whether it’s the transformer or GPT or something else like that, were built on top of smaller little breakthroughs that happened earlier on,” Mark Riedl, a computer scientist at the Georgia Institute of Technology, told me. Needing to show investors progress each fiscal quarter, then a source of revenue within a few years, limits what topics scientists can pursue; meanwhile, federal grants allow them to explore high-risk, long-term ideas and hypotheses that may not present obvious paths to commercialization. The largest tech companies, such as Google, can fund exploratory research but without the same breadth of subjects or tolerance for failure—and these giants are the exception, not the norm.
The AI industry has turned previous, foundational research into impressive AI breakthroughs, pushing language- and image-generating models to impressive heights. But these companies wish to stretch beyond chatbots, and their AI labs can’t run without graduate students. “In the U.S., we don’t make Ph.D.s without federal funding,” Riedl said. From 2018 to 2022, the government supported nearly $50 billion in university projects related to AI, which at the same time received roughly $14 billion in non-federal awards, according to research led by Julia Lane, a labor economist at NYU. A substantial chunk of grant money goes toward paying faculty, graduate students, and postdoctoral researchers, who themselves are likely teaching undergraduates—who then work at or start private companies, bringing expertise and fresh ideas. As much as 49 percent of the cost of building advanced AI models, such as Gemini and GPT-4, goes to research staff.
“The way in which innovation has occurred as a result of federal investment is investments in people,” Lane told me. And perhaps as important as federal investment is federal immigration policy: The majority of top AI companies in the U.S. have at least one immigrant founder, and the majority of full-time graduate students in key AI-related fields are international, according to a 2023 analysis. Trump’s detainment and deportation of a number of immigrants, including students, have cast doubt on the ability—and desire—of foreign-born or -trained researchers to work in the United States.
If AI companies hope to bring their models to bear on scientific problems—say, in oncology or particle physics—or build “superintelligent” machines, they will need staff with bespoke scientific training that a private company simply cannot provide. Slashing funding from the NIH, the NSF, and elsewhere, or directly withdrawing money from universities, may lead to less innovation, fewer U.S.-trained AI researchers, and, ultimately, a less successful American industry. Meanwhile, multiple Chinese AI companies—notably DeepSeek, Alibaba, and Manus AI—are rapidly catching up, and Canada and Europe have sizable AI-research operations (and healthier government science funding) as well. They will simply race ahead, and other companies could even relocate some of their American operations elsewhere, as many financial institutions did after Brexit.
If the pool of talented AI researchers shrinks, only the true AI behemoths will be able to pay them; as the pool of federal science grants dwindles, those same firms will likely further steer research in the directions that are most profitable to them. Without open academic research, the AI oligopoly will only further cement itself.
That may not be good for consumers, nor for AI as a scientific endeavor. “Part of what has built the United States into a real juggernaut of research and innovation is the fact that people have shared research,” Alondra Nelson, a professor at the Institute for Advanced Study who previously served as the acting director of the White House Office of Science and Technology Policy, told me. OpenAI, Anthropic, and Google share limited research, code, or training data sets, and almost nothing about their most advanced models—making it difficult to check products against executives’ grandiose claims. More troublingly, progress in AI—and really any technology or science—depends on collaboration among people and pollination of ideas. These firms could plow ahead with the same massive, expensive, and energy-intensive models that may not be able to do what they promise. Fewer and fewer start-ups and academics will be able to challenge them or propose alternative approaches; these firms will benefit from fewer and fewer graduate students with outside perspectives and expertise to spark new breakthroughs.
President Trump may not care much for these scientists. But there is one he holds in high esteem who might have had something to say about all this. The president’s late uncle, John G. Trump, was a physicist at MIT who did pioneering work in clinical and military uses of radiation. The president has called Uncle John a “super genius.” John Trump received a national medal of science from the NSF, and his work was supported by at least hundreds of thousands of dollars in grants from the agency—more than $4 million today—in addition to funding from the NIH, according to his papers in the MIT archives and government reports. Those NSF grants supported at least six doctoral, 20 master’s, and 13 undergraduate theses in Trump’s lab—and that was one 14-year period in the elder Trump’s decades-long career.
As I did research for this article, I found the scientist’s final research report to the NSF upon the conclusion of those 14 years, written in 1966.
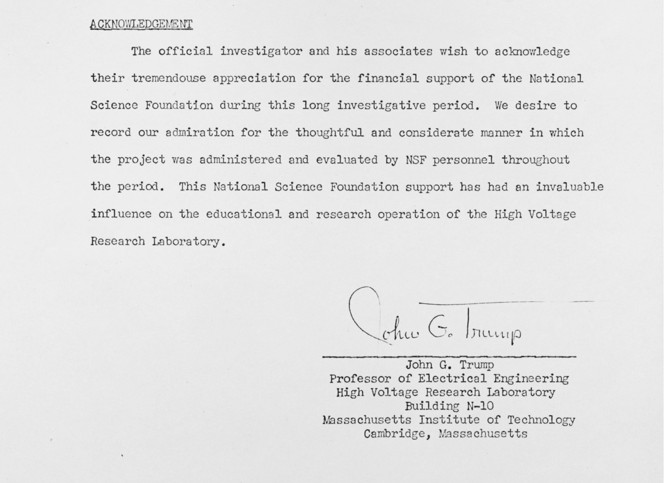
John G. Trump took care to note his team’s “tremendouse [sic] appreciation for the financial support of the National Science Foundation” and its “admiration for the thoughtful and considerate manner in which the project was administered and evaluated by NSF personnel.” The foundation’s support, Trump said, had been an “invaluable influence on the educational and research operation” of his lab. Almost 60 years later, education and research no longer seem to be among the nation’s priorities.
The madness started, as baseball madness tends to start, with the New York Yankees: At the end of March, during the opening weekend of the new season, the team’s first three batters hit home runs on the first three pitches thrown their way. The final score, 20–9, was almost too good to be true. And then, everybody noticed the bats.
A handful of Yankees had used unconventional instruments to hit their home runs: Their bats bulged out a little near the end, such that they were shaped more like bowling pins than clubs. It turned out they’d been designed by an MIT-trained physicist and were tailored to each player’s swing, with the bulge positioned at the place on the bat where that player tends to hit the ball. Yes, after at least a century’s worth of baseball bats that all looked more or less the same—“it must be made of wood, and may be of any length to suit the striker,” reads a set of rules from 1861—the art of making striker’s wood had at last produced a major innovation. After the Yankees hit a franchise-record nine home runs in that one game, media coverage of torpedo bats exploded, and manufacturers are struggling to meet demand from other teams. Even fantasy baseball leagues have cottoned to the trend. “This is torpedomania,” said the CEO of a major bat maker.
At first glance, the craze appears to be the culmination of the data-driven tweaks that have overhauled the modern game. A pursuit of minute statistical advantages characterizes nearly every aspect of baseball today: Pitchers maximize effectiveness by throwing the ball as hard as possible, and rarely spend more than five innings in a game; managers eschew traditional—and suboptimal—strategies such as bunting and stealing bases; fans obsess over esoteric performance metrics with names such as “wRC” and “xFIP.” Now the data revolution is reimagining one of the game’s most fundamental tools: the bat.
The idea of the bowling-pin shape is actually a few years old and has been explored by multiple teams. Aaron Leanhardt, the aforementioned MIT physicist, began designing the Yankees’ torpedo bats in 2022 as a minor-league hitting coach for the team, and some major leaguers were using them last year. His premise was straightforward: Standard bats are widest and heaviest at the tip, but players prefer to make contact with a pitch closer to the midpoint. That’s in part because a bat’s “sweet spot”—the portion of the wood that transfers the most energy on contact—is also a few inches down the barrel from the end. To address this inefficiency, torpedo bats are made with more wood in the sweet spot and less wood elsewhere—thus, the bulge. The idea was to “put it where you’re trying to hit the ball,” Leanhardt told The Athletic.
But that premise may be suspect. Despite their Moneyball makeover, torpedo bats remain, for now, a blunt instrument, largely superstition with a patina of data. Though it seems like common sense that adding heft to the part of the bat where a player hits the ball would be advantageous, several physicists who study baseball bats told me that’s not necessarily true. Because a bat has a thick barrel and rotates when swung, its motion and power depend on the distribution of weight across the entire shaft, not just in one spot. In other words, the physics aren’t cut-and-dried: A bulging sweet spot may provide more space for making contact with the ball, but it likely won’t provide more power. (The Miami Marlins, for whom Leanhardt now works as a field coordinator, declined a request for comment.)
[Read: Why aren’t women allowed to play baseball?]
All of the mass along a bat’s barrel, not just at the point of contact, contributes to the impact. As a result, shifting some wood from the end of the barrel to the sweet spot will not make the bat more powerful, Lloyd Smith, a mechanical engineer who studies ball-bat collisions at Washington State University, told me. Brian Hillerich, the director of professional bat production at Hillerich & Bradsby Co., which makes bats for Louisville Slugger, said that even if torpedo bats are not more powerful, they still promote more consistent contact at the sweet spot, which would tend to help a player’s performance. Smith and other physicists said this is possible, but remains unproved.
In any case, by redistributing some mass closer to the handle, the bowling-pin design could actually make a bat feel lighter when swung—it could lower the “moment of inertia,” in physics parlance. That will allow a player to increase his bat speed, but it also shrinks the force he can apply upon contact. These two factors may well cancel out, Dan Russell, a physicist at Penn State who studies baseball-bat vibrations, told me. (Imagine swinging a hammer while gripping its head instead of its handle: It might move faster, but it wouldn’t do a better job of pounding nails.) A torpedo bat could also be constructed by adding extra wood to make the bulge instead of merely shifting it from other places on the barrel. This would keep the “moment of inertia” constant—the bat would be heavier on a scale but feel the same when swung. Baseball bats used to have more heft as a rule; Babe Ruth swung clubs perhaps 50 percent heavier than today’s. But the net effects remain unclear, and would depend on each particular player’s strength and swing.
A faster swing could still be useful even if it doesn’t give a hitter greater power: “You simply have better bat control, can wait a little longer on the pitch before deciding to swing, make adjustments once you’ve started,” Alan Nathan, who studies the physics of baseball at the University of Illinois, told me. That won’t be the case for everyone—athletes who have spent years honing their swings and timing could be thrown off by the new shape, and several players using torpedo bats have had terrible starts to this season. Hillerich told me that his company designs torpedo bats with this in mind, trying to make them feel as similar to a player’s original bat as possible. It might all be a matter of preference and confidence—and others may not care that much either way. The new shape feels the same in his hands, Jazz Chisholm, a torpedo-wielding Yankee, recently said. “I don’t know the science of it. I’m just playing baseball.”
That the Yankees had a historically great game, and that some players were using funny-looking bats, “is more coincidence than destiny or science,” Smith told me. After all, nobody noticed the new shape last season, and for good reason—there’s simply not enough information, either from MLB games or physics labs, to definitively say what these bats offer, and to which players. Smith said he suspects that “the number of athletes this torpedo bat benefits is going to be fairly narrow.”
Indeed, the current buzz about the bats is pretty much the opposite of being data-driven. In an interview last week with The Athletic, Brett Laxton, the lead bat maker at Marucci Sports, pointed to the fact that Giancarlo Stanton, the Yankees’ designated hitter, had hit three home runs in his first game using a torpedo bat last year. That was “a good eye test” of the technology, he said, invoking just the kind of baseball intuition that statistics-driven analysts would sneer at. Yes, the bat felt and looked good in Stanton’s hands; no, this is not sabermetrics. Meanwhile, other “eye tests” have yielded more ambiguous results. Elly de la Cruz, of the Cincinnati Reds, hit two home runs in his first game using the torpedo bat, for instance, then went 0–4 the next day. Max Muncy, of the Los Angeles Dodgers, tried using a torpedo bat and recorded three outs in a row, then switched back to his old wood and hit a game-tying double.
If anything, the torpedo bats harken to an era before Moneyball, computers, or even the official formation of Major League Baseball. The late 1800s were a time of “great experimentation” in bat design, John Thorn, MLB’s official historian, told me: four-sided bats and flat bats, bats with slits for springs and sliding weights. All of that tinkering has long been left behind, however, and the modern, non-torpedo bat now seems like a simple fact of the game. Perhaps the biggest change to bat manufacturing in recent decades happened in the 1990s, when Barry Bonds started swinging bats made from maple instead of ash, and the rest of the league followed. That, too, had an element of superstition: As it turns out, a bat made from maple wood transfers a little bit less energy to a ball than one made from ash. Bonds, who hit more home runs than any MLB player in history, “could have hit the ball just a bit further if he had stayed with ash,” Smith told me.
Thorn takes issue with the whole discussion. “The whole idea that the magic is in the bat rather than in the batter is fraud,” Thorn said. “It’s calumny.” Of course, baseball players and fans have always been in pursuit of magic. They once used less pretentious tricks—eating chicken before each game, wearing a gold thong to emerge from a funk—but these have now been funneled through the optimization craze; instead of mismatched socks, there are “literal genius”–designed bats. In an era when baseball teams will squeeze any source of data for tiny statistical advantages, torpedomania pretends to be yet another nerd-ish secret weapon. Perhaps, for some subset of players, the new design really is miraculous. More likely, though, when the stats have all been counted and compared, we’ll discover that the torpedo bat is no different from any other talisman in baseball: a ridiculous distraction; a delightful waste of time.
Sign up for Trump’s Return, a newsletter featuring coverage of the second Trump presidency.
To plainly state what is going on right now would make you sound delusional.
The president of the United States has essentially been holding the global financial system hostage. He’s done so by threatening massive tariffs on nearly 100 countries and territories, including one that is unpopulated. These tariffs, which were set to go into effect today but have now been put on a 90-day pause for every country except for China, which will see a 125 percent tax on exports, seem to have been calculated using a blanket formula that some economists and trade experts find almost nonsensical. (Many have speculated that the math was done by a chatbot.) The administration’s supporters do not have a coherent message about why any of this is happening; before President Donald Trump announced the pause, they’d argued simultaneously that the tariffs were a temporary negotiating tactic but also that they might be durable enough to bring manufacturing jobs back to the United States.
As the world braced for Trump’s tariffs to go into effect, stocks plummeted, wiping out trillions of dollars in value. The price of government bonds also dropped precipitously, triggering genuine fear of a dangerous debt crisis. Wall Street analysts likened the tariffs to a U.S. tax increase of roughly $660 billion; one economist called it a set of “punishing sanctions against the American people.” The situation is volatile: 10 percent universal tariffs are still in place, inflation remains a concern, America is still in a trade war with China, and it’s not at all clear that Trump will not just repeat this act as we approach the 90-day deadline.
For many, the second Trump administration has felt like a constant tearing of the fabric of our reality. The country is facing a deadly measles outbreak after Congress confirmed Robert F. Kennedy Jr., a man with a profound skepticism of modern medicine, to run the Department of Health and Human Services. For a time, the businessman Elon Musk seemed to be acting as a shadow president, and he’s participated in the gutting of the federal government (when he’s not streaming himself playing video games on social media). ICE has apprehended visa-holding students off the street—sometimes for no clear reason at all.
While many of these acts have been ignored or rationalized by the president’s supporters, the tariffs may be different. Can the culture war—largely powered by memes, ideologies, vibes, and extremely online radicalization—survive the harsh reality of a trade war? The pause is only temporary; if Trump does not relent, he might still cause a financial meltdown. Trump has also destroyed a lot of trust—just the possibility of future tariffs may cause enough uncertainty to hurt businesses and investors. This would be the ultimate stress test of people’s otherwise unshakable devotion to the president—a final gantlet for this MAGA delusion.
Tech leaders and venture capitalists who have cozied up to Trump were already harmed by the threat of tariffs. Their stock prices are down, and the broad duties planned by the administration will severely affect supply chains, making core products such as smartphones more expensive. (The 125 percent tax on Chinese exports that remains might still have this effect.) There’s genuine concern that the entire industry could be set back years as the uncertainty caused by Trump’s erratic decision making hinders the building of supercomputers as well as strategic investments by VCs. One analyst called it an “economic armageddon” for the industry.
[Read: Buy that new phone now]
Although none of the big technology companies have issued a public statement against the tariffs, some cracks have begun to show. Musk, an administration loyalist, has made personal appeals to Trump to reverse course and used his platform on X to repeatedly insult Peter Navarro, one of the architects of the tariffs, calling him “dumber than a sack of bricks” and giving him the nickname “Peter Retarrdo.” A few other pro-Trump investors and entrepreneurs have issued their own frustrations: The Palantir co-founder Joe Lonsdale tepidly critiqued the rollout, suggesting that “the tariffs could be done better.” Others have indicated that they were misled by enthusiasm for Trump’s culture warring.
Bill Ackman, the billionaire hedge-fund manager and ardent Trump supporter, might have offered the best example of buyer’s remorse on Sunday, when he posted on X that the tariffs were the equivalent of an “economic nuclear war.” He posited that the United States “will severely damage our reputation with the rest of the world that will take years and potentially decades to rehabilitate.” Later, he admitted that he’d underestimated Trump’s penchant for chaos. “I don’t think this was foreseeable,” he posted, referring to the tariffs Trump touted throughout his campaign. “I assumed economic rationality would be paramount. My bad.” The contrition didn’t last: After the pause was announced, Ackman posted on X, “This was brilliantly executed by @realDonaldTrump. Textbook, Art of the Deal.” As of this writing, there is no evidence from the White House that Trump received any specific concessions from the tariffed nations; he merely created a crisis and then paused it because the world (and markets) got spooked. “People were getting a little bit yippy,” Trump told reporters this afternoon.
[Jonathan Chait: Trumpworld makes the case against Trump]
Just as notable are the justifications from the far-right influencers who have served as a de facto propaganda arm for the White House. As markets tanked last week, they swiftly argued in favor of broad austerity measures as a necessary hardship, suggesting that Americans do “not need” consumer electronics such as cellphones and video-game consoles and that American lives have not been changed by the tariffs. Benny Johnson, a Trump loyalist, social-media personality, and podcast host, went further: “Losing money costs you nothing,” he said on his podcast. “In fact, you learn a lot of lessons actually by losing money. Losing your character costs you everything. Losing your country loses you everything.” Other MAGA devotees and some Fox News hosts have conscripted tariffs into the culture wars, justifying them as helping to fix the country’s “crisis in masculinity.”
It’s not just the shock jocks. On X, entrepreneurs, venture capitalists, and a faction best described as an anti-woke tech commentariat posted long threads, proclaiming the tariffs a Trumpian masterstroke. Chamath Palihapitiya, a venture capitalist and one of the hosts of the All-In podcast, recently hypothesized that Trump would bring world leaders to Mar-a-Lago to remake the world economy in what Palihapitiya referred to as “Bretton Woods 2.0.” Keith Rabois, a well-known tech investor, declared that “Trump Derangement Syndrome has morphed into Tariff Derangement Syndrome,” suggesting that the slew of economists and trade experts horrified by the administration’s proposal are all wrong. “I would believe in any macro trader w sustained returns for decades over any academic,” he wrote.
This combination of blind faith, reactionary ideology, and endless justification have less in common with any kind of politics and policy than they do with genuine conspiratorial thinking—a kind of QAnon 2.0 for finance. As in QAnon, a sprawling network of conspiracy theories about a nefarious cabal referred to as the “deep state,” Trump is made out to be a hero, pulling the strings and playing multidimensional chess to deliver political salvation to his devotees. QAnon’s earliest prediction, back in 2017, revolved around Trump “removing criminal rogue elements,” including arresting Hillary Clinton. But the broader goal was a dismantling of the New World Order. The arrests never came, but the elaborate predictions continued. QAnon became a cult in which followers were given “crumbs” and told to “trust the plan.” The group’s slogan was an admission of total surrender to the conspiracy: “Where we go one, we go all.”
It’s not a perfect one-to-one comparison, but many of the dynamics are quite similar. Both QAnon and the legion of tariff true believers share an unabiding faith in Trump, ascribing his erratic governing style to a master plan. As my colleague Adrienne LaFrance wrote in 2020, in QAnon, “every contradiction can be explained away; no form of argument can prevail against it.” Similarly, ardent supporters of Trump’s tariffs have refused to see any of the plan’s glaring flaws: Fluctuations in the stock market are dismissed as not real or, in some cases, a good thing, because they hurt the “bastards” on Wall Street. Either the sell-off is part of a necessary cleansing of the American economy or it’s a blip, the result of Trump playing chicken with world leaders. In some cases, supporters argue that the stock market has almost nothing to do with the long-term health of the economy at all. To these people, the notions that the tariffs will hurt the working-class families they’re supposed to liberate and that they could halt the domestic manufacturing they’re supposed to energize are distractions seeded by an enemy who doesn’t want you to see the end game.
But above all, the prime directive for both QAnon and the tariff truthers is the same: Trust the process. The tech investor and Trump supporter Shaun Maguire wrote on X last week that the tariffs are better than maintaining the status quo: “Stay the course and have a 100% chance you lose in 20 years…Or do the hard things and have a 70% chance you win.” These numbers, it’s worth noting, appear to be random, but they represent a kind of unflinching devotion to a plan that doesn’t seem to exist. Trump, taking a page from Q’s book, issued his own version of this message on Monday, telling supporters on social media, “Don’t be Weak! Don’t be Stupid! Don’t be a PANICAN (A new party based on Weak and Stupid people!).” In response, Fox News ran a lower-third banner with the phrase Good Things Come To Those Who Wait. On X, far-right influencers began using Trump’s term—Panican—as a way to dismiss earnest criticism and concerns about the tariffs. Just as QAnon offered promises of a Great Awakening, in which the deep state was vanquished and the truth of the evils of the global elite cabal was revealed, the tariffs also offer the promise of an imagined utopia. The far-right activist Jack Posobiec summed up the attitude best on X on Monday: “My own retirement account is down too … Don’t care … All-in on the Great Deal … The Golden Age is on the other side.”
The TariffAnon crowd is right about one thing: Even the near future is deeply uncertain. As I wrote these words, the market rallied (the Panicans were fools all along!), only to lose all gains a few hours later (the Panicans are making too big a deal out of market fluctuations). After announcing his pause, Trump confusingly also said that the U.S. would apply additional 10 percent tariffs to Canada and Mexico. It remains possible that Trump could successfully negotiate a bunch of trade deals, placating the markets and further cementing him as a hero among his base. Or it could all go disastrously.
Some political observers hope that Trump single-handedly inflicting deep financial pain on the world might break the spell among his most ardent supporters. In this way, the tariffs are a reality test. But if the recent past is any guide, what many dismiss as bad-faith rhetoric can quickly harden into a core ideology for a set of true believers; the anti-vax movement and the subsequent measles outbreaks are a sobering analogue. This is how breaks with reality occur now, aided, in part, by the internet’s justification machine, which is an efficient mechanism for dispelling any trace of cognitive dissonance. In some cases, falling down the conspiratorial rabbit hole is motivated by grievance—my enemies hate this, therefore it must be good. And any market bounce-back after Trump’s announcement of a 90-day pause will be taken by true believers as evidence of the president’s genius (see: Bill Ackman).
Some Trump supporters are also worried about their reputations. For many outspoken VCs, backing Trump was a bet, much like investing in a portfolio company—one that came with substantial risk. Should Trump’s tariff scheme backfire completely by causing a recession or worse, their reputations as risk managers and complex-systems thinkers would naturally be tarnished (see: Bill Ackman). Unless, of course, they chose to double down and create a fictional narrative in which they cannot be wrong. The truthers are, in essence, talking up their reputational investment —as if the Trump administration was a stock they were holding. As Trump himself has proved throughout his political career, it is often easier to double down and build a fantasy world around your mistake than to admit you were wrong.
That sufficiently motivated people might break with reality is by now somewhat predictable in American politics. But what’s far more uncertain is what happens when reality punctures the protective bubble of cognitive dissonance. QAnon devotees and MAGA die-hards were certain that Trump won the 2020 presidential election. When reality became impossible to ignore, we got January 6. Few events have the power to pierce the veil, but a global financial crisis could be one of them. What happens if the true believers are confronted with a truth they can’t look away from? History suggests it won’t go well.
The tariff apocalypse is upon us. Should you buy an iPad?
Some people, it seems, have answered with a resounding yes. Bloomberg reported yesterday that at some Apple stores, “the atmosphere was like the busy holiday season.” Fearing that the price of electronics will increase as a result of President Donald Trump’s tariffs, people are rushing to purchase stuff. If the economy must collapse, at least let it do so after you have obtained a new tablet for $599 plus tax.
The panic-buying is a little funny. First, because if we are on the eve of a global recession, we have bigger things to worry about than new gizmos. Second, because we are still in the haze of a Trump pseudo-reality. The major tariffs will not hit until tomorrow, assuming there isn’t some unexpected reversal in the next several hours, and no one knows for certain how any of this will shake out.
Apple, in particular, is a good case study for the moment. Consumer electronics—and especially smartphones—illustrate perfectly the gulf between the president’s America-first agenda and the inescapable realities of a globalized supply chain. Many articles have suggested that electronics will become much more expensive if the tariffs stick—perhaps by hundreds of dollars. They certainly will become more expensive for companies like Apple to make. Although the company may have clever ways of reducing its costs—by shipping devices from India rather than China, for example—it’s not hard to imagine some of the expense being passed down to consumers. (Apple did not respond to a request for comment.)
This is, however, far from certain. If America enters a recession, depressed demand might lead to some nice markdowns, which you might take advantage of if you’re not financially preoccupied with keeping a roof over your head or food in your kitchen. The Trump administration could also produce some kind of carve-out for Apple and other American tech firms to do business without paying the tax. (Apple, like other major tech companies, has cozied up to Trump; the company’s CEO, Tim Cook, was present for Trump’s inauguration in January.) When the previous Trump administration announced tariffs on consumer goods imported from China, Apple products were exempt. This was in the interest of the president’s America-first agenda. As Neil Cybart, an Apple analyst, explained in a recent edition of his Above Avalon newsletter, “Any action that stands to hurt the iPhone may benefit non-U.S. smartphone manufacturers.” At a certain point, phones are phones, and people will turn elsewhere: Huawei’s latest is covered in vegan leather, has three folding screens, and makes my iPhone 15 look like meemaw’s clunky old Mr. Coffee.
Trump apparently wants the tariffs to revitalize American manufacturing. He is excited, for instance, that Wyoming hamburgers will once again compete with Australian beef. His commerce secretary, Howard Lutnick, said in an interview over the weekend that “the army of millions and millions of human beings screwing in little screws to make iPhones—that kind of thing is going to come to America.” (Never mind that Lutnick immediately said that the work would be “automated.”)
But global supply chains are a tangle that no presidential administration could easily unwind. In 2016, the journalist Konstantin Kakaes explored the possibility of an “All-American iPhone” for MIT Technology Review and determined that such a thing is not really possible: “The iPhone is a symbol of American ingenuity,” he wrote, “but it’s also a testament to the inescapable realities of the global economy.” As things stand, no one country is capable of producing all of the rare-earth elements that go into a single device, and components such as screen glass and processors are also sourced from all over the world. China refines 90 percent of the world’s rare earths; the tantalum in your phone is probably sourced from a mine in the Democratic Republic of the Congo; its processor likely came from Taiwan. If you want to understand globalization, pay attention to the iPhone.
It would not take an act of God to change this situation, but it might take more than Stephen Miller. America would need an iron resolve, lots of money, and lots of time. Rare-earth deposits exist in the United States—but finding, extracting, and refining them would be tremendously costly and dangerous work. Toxic by-products and environmental devastation would ensue. Americans have been happy to ignore these realities when the work is done elsewhere. Would they be so happy if it were done here? Add to that the need to build factories and fabrication plants for microchips. The effort would demand billions upon billions of dollars of investment over many years, still may not accommodate demand for these products, and would make them drastically more expensive.
In theory, moving some greater amount of electronics manufacturing to U.S. soil could be a beneficial strategy. American technology firms are highly reliant on chips imported from Asia, for instance, which means the supply is vulnerable to disruption. “It would be hard to survive a total cutoff of chips from Taiwan,” Duane Boning, an electrical-engineering and computer-science professor at MIT, told me. The CHIPS Act, signed into law by Joe Biden, has already funded some of this manufacturing in the United States. But tariffs are unlikely to help these efforts, Boning said. (If anything, the costs they would impose on various components might only hurt.)
[Read: Just how badly does Apple need China?]
Consumer electronics are only one way to look at Trump’s tariffs. But keep in mind that Apple, Microsoft, Nvidia, Amazon, and Alphabet are the five most valuable companies in the world—all American, all with products that will be caught in this mess. If the plan doesn’t make sense for them—if the plan will actually hurt their business—then what sense does it make at all?
That said, sense-making is a fool’s errand in this era. What we can do is deal with what’s in front of us. So, sure, buy the new phone or tablet if you have to. But also ask yourself: Do you really have to? If Apple’s prices do rise, the company’s sales could be an early indicator of whether Americans are at all willing to renegotiate their unhealthy relationship with consumerism. For the past decade, technology companies have conditioned buyers to make purchasing expensive gadgets an annual affair through services such as Apple’s iPhone Upgrade Program. (There is a reason that each iPhone model ends in a number: People intuitively understand that a 17 is better than a 16 is better than a 15, and so on.) Hundreds of millions of new devices are shipped every year. Not as many old ones are recycled. This is not wonderful: Consumers are taking their cues from the most moneyed corporations to have ever existed, cycling through handheld computers at a rapid clip because they come in new colors and with slightly reconfigured screens.
I’m not innocent of the vanity gadget upgrade. (I recently sacrificed a mostly fine old Kindle for a mere $5 trade-in credit on a newer model.) But I also know that those toxic rare-earth pools exist in great part because of the endless production of phones, computers, tablets, game consoles, and televisions. Meanwhile, many of these gadgets last longer than they might have even a few years ago, thanks to higher-quality materials, and are easier to fix due to the growing popularity of “right to repair” laws. The device in your hand would be a miracle in any other age. Nothing is certain right now. Chaos is everywhere. At least you can hold on to this.
White House staffers, it seems, had better hope that they stay in Laura Loomer’s good graces. This week, Loomer—a far-right provocateur who has described herself as “pro–white nationalism” and Islam as a “cancer on humanity”—met with Donald Trump in the Oval Office. After she reportedly railed against National Security Council officials she believed were disloyal to the president, the White House fired six NSC staff members the next day. More firings could be on their way: Yesterday, a person close to the administration told my colleague Michael Scherer that “Loomer has been asked to put together a list of people at State who are not MAGA loyalists.”
Loomer doesn’t have an official job in the Trump administration, and the president has denied that she had anything to do with the NSC firings. But she is one of the president’s confidantes, and she has come to exercise a significant amount of influence over the White House. Lots of people in Trump’s inner circle have unlikely backgrounds (defense secretaries are not usually hired straight from Fox News), but Loomer’s is probably the least likely of them all. Over the past decade, she has earned a reputation as an unapologetically racist troll. In 2018, after she was banned from Twitter for criticizing then-incoming Representative Ilhan Omar and her Muslim faith, she famously handcuffed herself to the door of Twitter’s New York City headquarters. She was reinstated after Elon Musk bought the platform, and has continued to post racist things on X: In September, she suggested that a Kamala Harris election win would mean that “the White House will smell like curry.” (Loomer did not respond to a request for comment.)
Loomer’s power marks how little Trump now seems to care about being around people who have expressed racist and extremist ideas and kept racist and extremist company. She is a bit like the Forrest Gump of Trumpworld—an unlikely but persistent character who just keeps popping up during some of the right’s biggest moments. When Trump got off his private plane on his way to the presidential debate in September, Loomer appeared with him. The next day, when Trump traveled to New York for a 9/11-anniversary memorial, Loomer was again there with him. (She has called 9/11 an “inside job.”) As I wrote at the time, prominent Republicans did not like that their presidential candidate was associating himself with Loomer, and publicly challenged Trump over it. He seemingly has not listened.
[Read: Laura Loomer is where Republicans draw the line]
Loomer has also played a role in directing discourse on the right. She was integral to drumming up the hoax that Haitian immigrants in Springfield, Ohio, were eating people’s pets, which became one of the most prominent right-wing causes du jour during the presidential campaign. In December, she instigated a war on the right over high-skilled immigration after she criticized the administration’s hiring of Sriram Krishnan as an AI-policy adviser and called attention to his past comments advocating for expanding H-1B visas. Most workers on H-1B visas are from India, and Loomer posted that Indian immigrants are “third world invaders.”
There is perhaps no one with direct access to the president who has been as outwardly and vociferously racist and bigoted. For all of the arguments that critics of Musk make about how he has boosted white supremacy on X, he has maintained a level of plausible deniability by never disparaging minority groups in a manner as direct as Loomer has. Someone like Loomer likely would not have had access to Trump in his first term. Richard Spencer never made it into Trump’s administration, and the president went out of his way to disavow the white nationalist. In 2018, when CNN reported that Darren Beattie, then a White House speechwriter, had spoken at an event that also featured a white nationalist, he was fired. But now Beattie has been hired to a senior role in this administration.
The president has already clearly established that his second term will be more extreme. Instead of the limited Muslim travel ban, he is pushing for mass deportations and has effectively shut down USAID, an agency largely devoted to helping poorer countries. But by welcoming Loomer into his inner circle, Trump is offering an even starker glimpse into how this administration is different. She is a testament to how much further to the right Trump has moved since his first term, and how much further he may be open to going.
Déjà vu: TikTok’s time was nearly up, and then President Donald Trump stepped in to save it. This happened in January, and also earlier today, when Trump said that he will sign another executive order to delay a possible ban on the social-media app.
Out of concern about the app’s possible weaponization by the Chinese government, Congress passed a law in April 2024 that required TikTok to spin off from its Chinese owner, ByteDance, or else stop operating in the United States. When the deadline for such a sale initially arrived earlier this year, American TikTok users had the hallucinatory experience of losing access to the app … for 14 hours. Trump intervened for the first time then by pushing the deadline for a sale back for 75 days—an extension he is now doubling—and the app soon came back. Months of news coverage, analysis, discussion, viral memes, and even some widely covered “Keep TikTok” protests outside the U.S. Capitol culminated in a brief shutout and a punted deadline. The app reappeared with a pop-up message thanking Trump for the reprieve: “As a result of President Trump’s efforts, TikTok is back in the U.S.!”
TikTok went back to business, mostly as usual. Many American companies and rich people have been rumored to have an interest in acquiring the app. These have ranged from the interesting but unserious (YouTube personality MrBeast or one of the Shark Tank guys) to the serious but uninteresting (current TikTok U.S. data-center operator Oracle) to the obvious names that would pop up no matter what big tech acquisition we were talking about (Elon Musk, Amazon, Microsoft). In his post today, Trump alluded to a pending deal without specifics. “My Administration has been working very hard on a Deal to SAVE TIKTOK, and we have made tremendous progress,” he said. “The Deal requires more work to ensure all necessary approvals are signed.”
This drama is getting tiresome. It’s just an app, and many Americans—at least those who are old enough to vote—don’t actually care that much about it. A 2023 Pew Research Center survey found that TikTok’s popularity was second only to YouTube among teenagers, but it’s far from the country’s most popular social-media app overall, despite its salience as a conversational stand-in for “internet culture” or “annoying thing that young people like.” “It’s a lot of fanfare and suspense over an app that, well, just isn’t all that important,” Kate Lindsay wrote in The Atlantic in January, pointing out that only a third of U.S. adults interviewed for another Pew survey said they’d ever used it. (More of these people say they use Pinterest!) Among young adults, she added, Snapchat and Instagram are more popular.
More recent polling from Pew shows a country with negative but not-very-strong feelings about the ban. Fifty percent of adults supported the idea in March 2023, and that number has now dropped to 34 percent. The percentage of Americans who oppose it has grown from 22 percent to 32 percent. But the percentage of Americans who say they’re not sure about it has risen as well, from 28 to 33 percent. We have approximately a three-way tie on the issue, with opposition and support about as prevalent as shoulder shrugs. Pew didn’t see a big partisan divide on this, either—39 percent of Republicans support the ban, compared with 30 percent of Democrats. People who oppose the ban tend to do so on free-speech grounds, whether they are Democrats or Republicans. They also tend to say they’re unconvinced by arguments about TikTok’s threat to national security.
Perhaps the problem is that these arguments were abstract in the way that data protection is always kind of abstract. They also seemed hypothetical, because as far as the public knows, the U.S. currently has no evidence that Chinese intelligence has ever accessed Americans’ data en masse via TikTok. That’s not to say there’s no chance that TikTok could be used for some nefarious purpose, but the app is not exactly an outlier in this regard; ByteDance employees have been caught using it to track journalists, for example, similar to the accusations more than a decade ago that employees at Uber were using their app’s “God View” to track individual users. The government has also made it seem like we have bigger problems on our hands when it comes to China (and not just a trade war). For instance, the Biden administration accused the Chinese of intelligence efforts to gain control of American power grids and water pipelines, which sounds acutely terrifying; the vague notion of China pushing propaganda on social media falls more into the bucket of “not ideal.” Trump acknowledged this disconnect in January with his signature wild directness: “Is it that important for China to be spying on young people? On young kids watching crazy videos?”
Of course, he was also the one to have first proposed the ban, back in 2020. At that time, some of his more important allies were anti–social media, arguably anti-tech Republicans such as Senator Josh Hawley of Missouri, who argued strenuously that TikTok was an urgent threat to national security. This was a time-limited approach—years passed and nightmare scenarios remained hypothetical. And Trump’s commitment to the ban disappeared when he was out of office. While campaigning in 2024, Trump made a TikTok account that now has more than 15 million followers; in his second term, his administration is tightly knit with the tech industry.
During President Joe Biden’s term, Congress followed through on the ban seemingly because it represented a quick and dramatic intervention to ease anxieties about social media in general. (This is the logic behind the billionaire Frank McCourt’s bid to acquire the app and strip it of its famous recommendation algorithm.) But Americans don’t currently appear to care very much about the narrative that TikTok is addictive, either. Fewer than half of the Pew respondents who said they support a ban cited people spending too much time on TikTok as a major reason. It wasn’t among the top three reasons overall.
The law on the books now reads as the semipermanent result of a political moment that has ended. The whole years-long debate may soon feel like a hallucination, too.
Deep down, Sam Altman and François Chollet share the same dream. They want to build AI models that achieve “artificial general intelligence,” or AGI—matching or exceeding the capabilities of the human mind. The difference between these two men is that Altman has suggested that his company, OpenAI, has practically built the technology already. Chollet, a French computer scientist and one of the industry’s sharpest skeptics, has said that notion is “absolutely clown shoes.”
When I spoke with him earlier this year, Chollet told me that AI companies have long been “intellectually lazy” in suggesting that their machines are on the path to a kind of supreme knowledge. At this point, those claims are based largely on the programs’ ability to pass specific tests (such as the LSAT, Advanced Placement Biology, and even an introductory sommelier exam). Chatbots may be impressive. But in Chollet’s reckoning, they’re not genuinely intelligent.
Chollet, like Altman and other tech barons, envisions AI models that can solve any problem imaginable: disease, climate change, poverty, interstellar travel. A bot needn’t be remotely “intelligent” to do your job. But for the technology to fulfill even a fraction of the industry’s aspirations—to become a researcher “akin to Einstein,” as Chollet put it to me—AI models must move beyond imitating basic tasks, or even assembling complex research reports, and display some ingenuity.
Chollet isn’t just a critic, nor is he an uncompromising one. He has substantial experience with AI development and created a now-prominent test to gauge whether machines can do this type of thinking. For years, he has contributed major research to the field of deep learning, including at Google, where he worked as a software engineer from 2015 until this past November; he wants generative AI to be revolutionary, but worries that the industry has strayed. In 2019, Chollet created the Abstraction and Reasoning Corpus for Artificial General Intelligence, or ARC-AGI—an exam designed to show the gulf between AI models’ memorized answers and the “fluid intelligence” that people have. Drawing from cognitive science, Chollet described such intelligence as the ability to quickly acquire skills and solve unfamiliar problems from first principles, rather than just memorizing enormous amounts of training data and regurgitating information. (Last year, he launched the ARC Prize, a competition to beat his benchmark with a $1 million prize fund.)
You, a human, would likely pass this exam. But for years, chatbots had a miserable time with it. Most people, despite having never encountered ARC-AGI before, get scores of roughly 60 to 70 percent. GPT-3, the program that became ChatGPT, the legendary, reality-distorting bot, scored a zero. Only recently have the bots started to catch up.
How could such powerful tools fail the test so spectacularly for so long? This is where Chollet’s definition of intelligence comes in. To him, a chatbot that has analyzed zillions of SAT-style questions, legal briefs, and lines of code is not smart so much as well prepared—for the SAT, a law-school exam, advanced coding problems, whatever. A child figuring out tricky word problems after just learning how to multiply and divide, meanwhile, is smart.
ARC-AGI is simple, but it demands a keen sense of perception and, in some sense, judgment. It consists of a series of incomplete grids that the test-taker must color in based on the rules they deduce from a few examples; one might, for instance, see a sequence of images and observe that a blue tile is always surrounded by orange tiles, then complete the next picture accordingly. It’s not so different from paint by numbers.
The test has long seemed intractable to major AI companies. GPT-4, which OpenAI boasted in 2023 had “advanced reasoning capabilities,” didn’t do much better than the zero percent earned by its predecessor. A year later, GPT-4o, which the start-up marketed as displaying “text, reasoning, and coding intelligence,” achieved only 5 percent. Gemini 1.5 and Claude 3.7, flagship models from Google and Anthropic, achieved 5 and 14 percent, respectively. These models may have gotten lucky on a few puzzles, but to Chollet they hadn’t evinced a shred of abstract reasoning. “If you were not intelligent, like the entire GPT series,” he told me, “you would score basically zero.” In his view, the tech barons were not even on the right path to building their artificial Einstein.
[Read: The GPT era is already ending]
Chollet designed the grids to be highly distinctive, so that similar puzzles or relevant information couldn’t inadvertently be included in a model’s training data—a common problem with AI benchmarks. A test taker must start anew with each puzzle, applying basic notions of counting and geometry. Most other AI evaluations and standardized tests are crude by comparison—they aren’t designed to evaluate a distinct, qualitative aspect of thinking. But ARC-AGI checks for the ability to “take concepts you know and apply them to new situations very efficiently,” Melanie Mitchell, an AI researcher at the Santa Fe Institute, told me.
To improve their performance, Silicon Valley needed to change its approach. Scaling AI—building bigger models with more computing power and more training data—clearly wasn’t helping. OpenAI was first to market with a model that even came close to the right kind of problem-solving. The firm announced a so-called reasoning model, o1, this past fall that Altman later called “the smartest model in the world.” Mark Chen, OpenAI’s chief research officer, told me the program represented a “new paradigm.” The model was designed to check and revise its approach to any question and to spend more time on harder ones, as a human might. An early version of o1 scored 18 percent on ARC-AGI—a definite improvement, but still well below human performance. A later iteration of o1 hit 32 percent. OpenAI was still “a long way off” from fluid intelligence, Chollet told me in September.
That was about to change. In late December, OpenAI previewed a more advanced reasoning model, o3, that scored a shocking 87 percent on ARC-AGI—making it the first AI to match human performance on the test and the best-performing model by far. Chollet described the program as a “genuine breakthrough.” o3 appeared able to combine different strategies on the fly, precisely the kind of adaptation and experimentation needed to succeed on ARC-AGI.
Unbeknownst to Chollet, OpenAI had kept track of his test “for quite a while,” Chen told me in January. Chen praised the “genius of ARC,” calling its resistance to memorized answers a good “way to test generalization, which we see as closely linked to reasoning.” And as the start-up’s reasoning models kept improving, ARC-AGI resurfaced as a meaningful challenge—so much so that the ARC Prize team collaborated with OpenAI for o3’s announcement, during which Altman congratulated them on “making such a great benchmark.”
Chollet, for his part, told me he feels “pretty vindicated.” Major AI labs were adopting, even standardizing, his years-old ideas about fluid intelligence. It is not enough for AI models to memorize information: They must reason and adapt. Companies “say they have no interest in the benchmark, because they are bad at it,” Chollet said. “The moment they’re good at it, they will love it.”
Many AI proponents were quick to declare victory when o3 passed Chollet’s test. “AGI has been achieved in 2024,” one start-up founder wrote on X. Altman wrote in a blog post that “we are now confident we know how to build AGI as we have traditionally understood it.” Since then, Google, Anthropic, xAI, and DeepSeek have launched their own “reasoning” models, and the CEO of Anthropic, Dario Amodei, has said that artificial general intelligence could arrive within a couple of years.
But Chollet, ever the skeptic, wasn’t sold. Sure, AGI might be getting closer, he told me—but only in the sense that it had previously been “infinitely” far away. And just as this hurdle was cleared, he decided to raise another.
Last week, the ARC Prize team released an updated test, called ARC-AGI-2, and it appears to have sent the AIs back to the drawing board. The full o3 model has not yet been tested, but a version of o1 dropped from 32 percent on the original puzzles to just 3 percent on the new version, and a “mini” version of o3 currently available to the public dropped from roughly 30 percent to below 2 percent. (An OpenAI spokesperson declined to say whether the company plans to run the benchmark with o3.) Other flagship models from OpenAI, Anthropic, and Google have achieved roughly 1 percent, if not lower. Human testers average about 60 percent.
If ARC-AGI-1 was a binary test for whether a model had any fluid intelligence, Chollet told me last month, the second version aims to measure just how savvy an AI is. Chollet has been designing these new puzzles since 2022; they are, in essence, much harder versions of the originals. Many of the answers to ARC-AGI were immediately recognizable to humans, while on ARC-AGI-2, people took an average of five minutes to find the solution. Chollet believes the way to get better on ARC-AGI-2 is to be smarter, not to study harder—a challenge that may help push the AI industry to new breakthroughs. He is turning the ARC Prize into a nonprofit dedicated to designing new benchmarks to guide the technology’s progress, and is already working on ARC-AGI-3.
[Read: DOGE’s plans to replace humans with AI are already under way]
Reasoning models take bizarre and inhuman approaches to solving these grids, and increased “thinking” time will come at substantial cost. To hit 87 percent on the original ARC-AGI test, o3 spent roughly 14 minutes per puzzle and, by my calculations, may have required hundreds of thousands of dollars in computing and electricity; the bot came up with more than 1,000 possible answers per grid before selecting a final submission. Mitchell, the AI researcher, said this approach suggests some degree of trial and error rather than efficient, abstract reasoning. Chollet views this inefficiency as a fatal flaw, but corporate AI labs do not. If chatbots achieve fluid intelligence in this way, it will not be because the technology approximates the human mind: You can’t just stuff more brain cells into a person’s skull, but you can give a chatbot more computer chips.
In the meantime, OpenAI is “shifting towards evaluations that reflect utility as well,” Chen told me, such as tests of an AI model’s ability to navigate and take actions on the web—which will help the company make better, although not necessarily smarter, products. OpenAI itself, not some third-party test, will ultimately decide when its products are useful, how to price them (perhaps $20,000 a year for a “Phd-level” bot, according to one report), and whether they’ve achieved AGI. Indeed, the company may already have its own key AGI metric, of a sort: As The Information reported late last year, Microsoft and OpenAI have come to an agreement defining AGI as software capable of generating roughly $100 billion in profits. According to documents OpenAI distributed to investors, that determination “is in the ‘reasonable discretion’ of the board of OpenAI.”
And there’s the problem: Nobody agrees on what’s being measured, or why. If AI programs are bad at Chollet’s test, maybe it just means that they have a hard time visualizing colorful grids rather than anything deeper. And bots that never solve ARC-AGI-2 could generate $100 billion in profits some day. Any specific test—the LSAT or ARC-AGI or a coding puzzle—will inherently contradict the notion of general intelligence; the term’s defining trait may be its undefinability.
The deeper issue, perhaps, is that human intelligence is poorly understood, and gauging it is an infamously hard and prejudiced task. People have knacks for different things, or might arrive at the same result—the answer to a math problem, the solution to an ARC-AGI grid—via very different routes. A person who scores 30 percent on ARC-AGI-2 is in no sense inferior to someone who scores 90 percent. The collision of those differing routes and minds is what sparks debate, creativity, and beauty. Intentions, emotions, and lived experiences drive people as much as any logical reasoning.
Human cognitive diversity, in other words, is a glorious jumble. How do you even begin to construct an artificial version of that? And when that diversity is already so abundant, do you really want to?
Last night, X’s “For You” algorithm offered me up what felt like a dispatch from an alternate universe. It was a post from Elon Musk, originally published hours earlier. “This is the first time humans have been in orbit around the poles of the Earth!” he wrote. Underneath his post was a video shared by SpaceX—footage of craggy ice caps, taken by the company’s Dragon spacecraft during a private mission. Taken on its own, the video is genuinely captivating. Coming from Musk at that moment, it was also somewhat depressing.
X fed me that video just moments after it became clear that Susan Crawford, the Democratic judge Musk spent $25 million campaigning against, would handily win election to the Wisconsin Supreme Court. Given Musk’s heavy involvement—the centibillionaire not only campaigned in the state but also brazenly attempted to buy the election by offering to pay voters $100 for signing a petition from his America PAC opposing “activist judges”—the election was billed as a referendum of sorts on Musk’s own popularity. In that sense, it was a resounding defeat. Musk, normally a frenetic poster, had very little to say about politics last night, pecking out just a handful of terse messages to his 218.5 million followers. “The long con of the left is corruption of the judiciary,” he posted at 1:23 a.m. eastern time.
In the light of defeat, the SpaceX post feels like a glimpse into what could have been for Musk—a timeline where the world’s richest man wasn’t algorithmically radicalized by his own social-media platform. It’s possible that Musk’s temperament and personal politics would have always led him down this path. But it’s also easy to imagine a version where he mostly stayed out of politics, instead leaning into his companies and continuing to bolster his carefully cultivated brand of Elon Musk, King of Nerd Geniuses.
[Read: The “rapid unscheduled disassembly” of the United States government]
Unfortunately, he surrendered fully to grievance politics. Like so many other prolific posters, he became the person his most vocal followers wanted him to be and, in the process, appears to have committed reputational suicide. Since joining President Donald Trump’s administration as DOGE’s figurehead—presiding over the quasi-legal gutting of the federal government—Musk has become not just polarizing but also genuinely unpopular in America. Now his political influence is waning, Tesla is the object of mass protest, and sales of his vehicles are cratering. This morning, only hours after his candidate lost, Trump reportedly told his inner circle and Cabinet members that Musk will be “stepping back” from his perch in the administration for a more “supporting role.” In Trumpworld, nothing’s over until it’s over, but Elon Musk seems to have overstayed his welcome. (Musk did not respond to a request for comment. A spokesperson for the White House referred me to Press Secretary Karoline Leavitt’s post calling the report that Musk is stepping back “garbage”; Musk posted on X that the reporting is “fake news.”)
Musk’s appeal to Trump has always been about two things: money and optics. As the richest man in the world, Musk is both a cash cow and a kind of enforcer: His checkbook and closeness to Trump remind Republicans in Congress that they can and will be primaried if they break from the administration. But Musk’s reputation is just as important to Trump, who respects great wealth and clearly enjoys being shadowed and adored by a man of Musk’s perceived stature and technological acumen (although Trump is easily impressed—take, for example, “Everything’s Computer!”) Musk’s image in Silicon Valley was useful to the Trump campaign, bringing in new fanboy voters and sending a message that the administration would transform the government and run it like a lean start-up.
But although his money is still good, the Wisconsin election suggests that Musk himself is an electoral liability. A poll released today, conducted in Wisconsin by Marquette University Law School, showed that 60 percent of respondents view Musk unfavorably, and a recent Harvard/Harris poll shows that his national favorability dropped 10 points from February to March. (He now has a net favorability rating of –10 percent.) An aggregation of national polls shows that the approval rating of his DOGE efforts has also dipped dramatically: Just 39 percent of Americans approve of his work, nearly 10 points lower than in mid-February.
To many observers, it seemed inevitable from the outset that, over time, Musk would clash with, and alienate himself from, Trump, a man who does not like to share the spotlight. But behind Musk’s low favorability rating is a simple notion: Americans (including Trump supporters) are uncomfortable and resentful of an unelected mega-billionaire rooting through the government, dismantling programs and blithely musing about cutting benefit programs such as Social Security. Musk has long behaved in business as though laws and regulations don’t apply to him—a tactic that seems to backfire more easily when applied to politics. His posts, which use captions such as “Easy money in Wisconsin” to offer thinly veiled bribes to state residents for posing outside polling locations, aren’t just questionably legal; they’re blatant reminders that the world’s richest man was attempting to purchase an election.
There is also, perhaps, a creeping sensation that Musk’s efficiency hunt into the government has not yielded the examples of corruption that Trump supporters crave. During a Q&A at Musk’s rally in Wisconsin on Sunday, one attendee asked if DOGE had found any evidence that “radical left” Democrats have received money from USAID, and if so, whether Musk planned to share the evidence. Musk stammered, explaining only that USAID’s money flowed circuitously and that it was suspicious that members of Congress were so wealthy. Throughout the rally, Musk seemed more interested in role-playing as a politician, delivering “extended monologues about immigration policy, alleged fraud in the Social Security system and the future of artificial intelligence,” as The New York Times reported.
[Read: Elon Musk looks desperate]
Trump may be realizing that though tech products and services may be quite popular, their creators are often less appealing. (Two-thirds of Americans have an unfavorable view of Meta CEO Mark Zuckerberg, for example, even as billions of people around the world use his platforms.) To those outside of the techno-optimist bubble, plenty of the obsessions of the tech elite (artificial general intelligence, cryptocurrency) can come off as weird or inscrutable. “As I mentioned several years ago, it increasingly appears that humanity is a biological bootloader for digital superintelligence,” Musk posted on X in the wee hours today, as if to prove the point.
Musk seems, at least outwardly, unable to reckon with his current position. Just days after framing this race as a hinge point for “the entire destiny of humanity,” Musk said on X that “I expected to lose, but there is value to losing a piece for positional gain.” From the outside, though, it’s difficult to see what he’s gained. Last week, protesters demonstrated outside hundreds of Tesla locations; Musk has long been erratic, but his dalliance with DOGE has alienated environmentally conscious liberals, a major demographic for electric vehicles. And by seeming so focused on DOGE, he’s frustrated investors who worry that Tesla is losing its first-mover advantage in the United States. Foreign rivals, such as China’s BYD, are quickly gaining steam. Tesla’s stock price instantly rose 15 points on the reports that Musk would soon leave the administration.
There is a case to be made that Musk’s cozying up to Trump will ultimately benefit Musk’s empire—avoiding regulations that may help with Tesla’s self-driving plans or SpaceX and Starlink contracts, for example. But so many of the signs point to a less desirable outcome. Musk’s outsize support of Trump was always a political risk, but his decision to come aboard the administration and, at one time, position himself as a kind of shadow president is arguably the biggest bet of his career. In the short term, it does not appear to be paying off.
Musk woke up this morning less popular than he’s been in recent memory. He’s alienated himself from an American public that used to widely revere him, and his political capital seems to be fading rapidly. The only question now is whether, after getting a taste of the political spotlight, he’ll be able to give it up without a fight.
If your virtual kart-racing life was missing something, you’re in luck. Nintendo, the Japanese electronics manufacturer, announced its new Mario Kart appliance today. The Switch 2, which can be used handheld or connected to a television, allows players to race go-karts piloted by characters from the company’s entertainment franchises: Mario, Yoshi, Princess Peach.
The karting games that ran on previous appliances allowed racers to compete on only a series of discrete tracks. But the updated hardware allows for something else: Mario Kart World, as the new software is called, presents its users with the tantalizing prospect of a digital commute. Racers may now convey from one track to the next through a large and continuous simulated world. This new capacity will unlock other new ways to kart, among them 1980s-style arcade racing and more contemporary, open-world kart tourism.
Longtime kart racers will surely celebrate the opportunity to kart anew. Someone who might have played Mario Kart 8—the previous fully original home release in the franchise—in 2014, when they were 12, has now graduated college. In the gaps between soul-crushing weeks at an investment bank or a management consultancy, karting sons and daughters who became karting adults might sneak in a nostalgic trip or race with their aging parents or once-baby siblings, now adolescents.
To facilitate the process, Nintendo has finally improved its online kart-racing infrastructure. Its competitors Sony and Microsoft, whose entertainment appliances mostly facilitate simulated sports or ritualistic arena murder, have allowed players to connect by voice or even video while playing, both to coordinate matches and to issue racist or homophobic taunts. The Switch 2 finally adds this capacity to kart racing, deployed via a “C” (“Cart”? No, “Chat”) button on its controllers.
[Read: Video games are better without stories]
All of this kart racing comes at a hefty price: $450 for the appliance itself, or $500 for the device bundled with the Mario Kart software. Those who would choose to forgo the bundle in favor of purchasing inscrutably updated rehashes of previous works, such as embarrassing fantasy-adventure games and insipid party titles, will have to hand over $80 for Mario Kart World if they choose to add it later. That might put kart-based home entertainment out of reach for many Americans. But others will surely see the value in the Switch 2, given the appeal and frequency of these karting delights.
Games such as Mario Kart World will be delivered on cartridges matching the size and shape of those from the previous appliance. Those carts may not contain software, instead acting as dummy keys that will unlock a probably time-consuming download. In exchange for this inconvenience, players will be able to “gameshare” some software titles with up to four friends, allowing the games to trickle down, Reaganomics-style, from the wealthy to the aspirant underclasses (though even these paupers will apparently still have to pay for a separate Nintendo Switch Online subscription to voice- or videochat with their game-giving overlords). But not Mario Kart World, which is ineligible for gameshare. All citizens must purchase their own access to karting.
[Read: The quiet revolution of Animal Crossing]
Nintendo has also updated the guts of the Switch 2 kart appliance. It will finally be capable of using the entire 4K resolution of the televisions that were being sold back when your college graduate was still 12. Note that the appliance itself features an LCD screen rather than the rich OLED displays that have been commonplace in smartphones for the past decade or so.
Nintendo has also failed to heed the lessons from its previous Mario Kart appliance. That device, the Switch, featured finicky, removable Joy-Con kart-racing controllers. Inevitably, kart-racing fanciers elected to pay exorbitant prices for traditional, add-on controllers instead. A new version of those controllers is also on offer for the Switch 2, requiring a new investment of $80 each for a racing tether that features the new “C” button.
Will this new kart chaos be worthwhile? Emphatically yes. I spent $1,600 on a new washer-dryer this year, and I use it only once a week, whereas I kart (or long to kart) far more often. Similarly, a good countertop air fryer might cost hundreds of dollars; why not a karting appliance too? And like an air fryer, which can toast and roast in addition to convection bake, the Switch 2 Mario Kart appliance is also capable of supporting other Nintendo-crafted experiences, such as an ape-oriented romp game announced today and, perhaps eventually, attempts to rehabilitate the non-karting titles from which the Mario character and his kindred had been mercifully liberated.
Steve Bannon seems resigned to sharing power with the “tech bros,” as he calls them. Last week, when I spoke with President Donald Trump’s former chief strategist and continued ally, he was clear about his disagreements with Elon Musk and other Silicon Valley elites on the so-called tech right. “Nationalist populists,” Bannon’s self-identified political clan, “don’t trust these oligarchs,” he told me. But, he added, “we see the usefulness of working together on broad things.”
It was a noticeably different tone for Bannon. In recent months, he has taken every opportunity to bash Musk. In January, Bannon said that the billionaire is a “truly evil guy, a very bad guy,” and that he’d have Musk “run out” of Trump’s inner circle by Inauguration Day. Musk and other “techno-feudalists,” Bannon said later that month, “don’t give a flying fuck about the human being.” In February, he called Musk a “parasitic illegal immigrant.” (Musk seemingly isn’t a fan of Bannon, either: “Bannon is a great talker, but not a great doer,” he posted on X in February. “What did he get done this week? Nothing.”)
The nationalist right and the tech right came together to elect Trump in November, but the MAGA coalition has seemed on the verge of falling apart ever since. The nationalist right sees social conservatism—such as mass deportations, heavy immigration restrictions, and a more explicitly Christian government—as paramount, even if it comes at the expense of free markets. Prominent members of the tech right, including the venture capitalists Marc Andreessen and Peter Thiel, prioritize private-sector technological progress above all else. In December, during a public spat with the nationalists over highly skilled immigrants, Musk posted on X that anyone who disagreed with him should “fuck” themselves “in the face.” Billionaire cosmopolitans who want to hire immigrants don’t mix easily with vehemently nativist populists who want to ratchet up taxes on said billionaires. Last month, in a speech at a tech summit in Washington, D.C., Vice President J. D. Vance said that he would “like to speak to these tensions as a proud member of both tribes.” This is not a thing you would feel the need to say about a relationship that is running smoothly.
[Read: The MAGA honeymoon is over]
But to assume that a fallout is inevitable is a mistake. The two sides don’t love each other, but for now, they are still together. Musk continues to be one of the most consequential people in government, and Bannon and other nationalists continue to hold sway over a White House that is cracking down on immigrants. Rather than split up, this new MAGA coalition might persist for years to come.
What the tech right and nationalist right are going through looks like an update to how conservatism has long worked. Especially before the Trump era, being “conservative” meant a commitment to free markets and traditional social views. This alliance wasn’t always a given. During the mid-20th century, traditionalists were suspicious of the potential harms of unchecked businesses, while libertarians saw excessive government encroachment as constraining the lives of Americans. But in spite of the difference, they still managed to stay together under one coalition. “Fusionism,” as the National Review editor Frank Meyer famously called it, worked because both sides had a clear, binding tie: opposition to communism.
The current moment looks a lot like fusionism 2.0: The uniting through line of opposing communism has been replaced by an opposition to “wokeness,” as the University of Virginia historian David Austin Walsh has observed. The similarities between the political moments provide a rough model for how the new MAGA coalition might persist. In the original fusionism, communism was almost always a bigger deal than whatever libertarians and social conservatives didn’t see eye to eye on. This worked because “communism” never just meant one thing. It could be invoked to oppose the Soviet Union and the literal ideology of communism, to thwart the supposedly communist-infilitrated civil-rights and anti-war movements, or to refer to anything related to left-wing politics.
Wokeness now serves that function on the right. It’s no coincidence that wokeness is a rebranded version of the right’s previous bogeyman: “cultural Marxism.” As Musk has ripped up federal agencies in a supposed effort to reduce government waste, a lot of what DOGE has actually focused on is rooting out perceived wokeness. Musk has enthusiastically helped carry out Trump’s anti-DEI executive order by targeting related programs for cuts, sometimes taking this to an almost-comic level of absurdity: DOGE reportedly placed one government worker whose job involved managing relationships with private-equity firms on administrative leave, seemingly mistakenly believing that the employee’s work was related to diversity, equity, and inclusion. Andreessen, who played an early role in staffing DOGE, has also railed against wokeness. Charlie Kirk, the right-wing podcast host and member of the conservative power elite, loves to talk about how much he hates wokeness, and has applauded DOGE for “hunting down those insane DEI departments.”
[Read: Charlie Kirk is the right’s new kingmaker]
In many cases, the two factions detest wokeness in the same specific ways. Joe Lonsdale, a Palantir co-founder and tech investor who is also a large donor to right-wing causes, recently posted that Columbia University “delenda est,” Latin for “must be destroyed.” Similarly, Bannon told me that he has urged Trump and other administration officials to target public universities such as the University of Michigan and the University of Wisconsin at Madison. “Purge the faculties and purge the administrators,” he said. “The states have no money to back up universities, so hold federal funding until they purge.”
Wokeness is even vaguer and more malleable than communism, which makes it especially useful. DEI hiring practices and critical race theory in schools count as wokeness on the right, but depending on whom you talk to, so is something as simple as a TV show or a movie with gay people. It can be bent into a passionate opposition to anything that might have the faintest traces of being liberal, left-wing, or progressive. “As long as there are common enemies,” Walsh, the UVA historian told me, the new MAGA coalition “will remain stable.”
People within the coalition at least partially agree. To the right, wokeness “is the glue that holds the left together,” Jeremy Carl, a senior fellow at the Claremont Institute, a conservative think tank, told me. Carl, a self-proclaimed nationalist who worked in the first Trump administration, explained that both factions of the right sincerely don’t like the rough assemblage of things they deem to be woke, but they also lean on it because they see it as a unifying point. “My nationalist group chats are not filled with people spewing anger at Elon,” he said. “They like Elon. They think he’s doing a great job."
None of this means that the tech right and nationalist right are destined to stay together. The groups in the original fusionism were often on a similar footing in terms of power. That’s not the case now. The tech elites hold a disproportionate amount of leverage in their ability to influence elections through massive donations. Musk is still throwing his money around, funneling millions of dollars to try to sway a Wisconsin Supreme Court race. His influence does not seem to be waning, even as he has continued to pursue an agenda at DOGE that has reportedly irritated a number of Trump’s other senior administration officials. This power imbalance gives the tech right an advantage. Even if the relationship still benefits the nationalists, they become the political equivalent of a group of suckerfish riding a whale.
When we spoke, Bannon rejected the entire idea of fusionism 2.0. “Let me say it bluntly: Fuck fusionism,” he said. At another point in the conversation, he referred to Silicon Valley as an “apartheid state” in which white-collar tech jobs are filled by immigrants instead of native-born Americans. In Bannon’s view, MAGA is not fusing together so much as begrudgingly forced to stick together—the way that the Democratic Party was once a coalition between northern liberals and southern Dixiecrats.
But even if Bannon’s view is right, it still suggests a MAGA coalition that is far more robust than it seemed just a few months ago. The Silicon Valley elite, Musk especially, has already moved closer to the nationalist right over the past several years. The nationalists, however wary of the tech right, have welcomed them. They understand that Musk and the rest of the tech right are deep-pocketed, powerful allies against wokeness in all its forms. Each faction still has every reason to keep putting its differences aside.
In Los Angeles, where I live, you don’t expect to be heckled while driving an electric car to the grocery store. But on a recent afternoon, a couple of men on bikes saw the Tesla logo on the front of my car and shouted, “Fuck you, Tesla guy” as I rolled by with the windows down.
I bought my Tesla Model 3 in 2019, after my wife and I moved from New York to L.A. and needed a car. Not willing to burn gasoline, we got the most practical EV we could afford. Six years later, that car carries a different connotation.
In the aftermath of Elon Musk’s MAGA embrace and his scorched-earth tactics running DOGE, Tesla has become a primary target for protests. On Saturday, demonstrators marched outside all 277 Tesla showrooms and service centers in the United States; Teslas across the country have been vandalized and even destroyed in recent weeks. Even in Los Angeles, where Teslas are as familiar as Fords and not primarily viewed as right-wing totems, this wasn’t the first time I’d been shouted at since the election. Tesla owners who don’t support Musk are playing defense. Some have begun to slap on bumper stickers such as I bought this before Elon went crazy. Others are simply done. Sheryl Crow sold her Tesla and donated the money to NPR; Senator Mark Kelly of Arizona also got rid of his, saying he couldn’t stand to own a car that is a “rolling billboard for a man dismantling our government and hurting people.” Teslas that are eight years old or newer now account for 1.4 percent of all car trade-ins, up from 0.4 percent a year ago, and EV brands such as Lucid and Polestar offer tantalizing deals to Tesla drivers looking for an out. “You’re definitely seeing a lot of people say, ‘You know what? I don’t want to be associated with the trash that’s going on right now around Elon Musk,’” Robby DeGraff, an analyst at AutoPacific, told me. “‘I’m just going to get rid of my car.’”
The Great Tesla Sell-Off is producing a glut of politically tainted pre-owned cars. Used Teslas are now shockingly cheap: One 2021 Model 3 for sale near me, which would have cost more than $50,000 new, is going for less than $20,000. Even if you resent Musk, you should consider buying one. In this moment of DOGE madness, it’s difficult to see a Tesla as anything other than an avatar of Musk. But Elon doesn’t make any money off the Model Y you get secondhand. Strip away the symbolism, and an old Tesla is just a good, affordable car.
[Read: My day inside America’s most hated car]
No, you shouldn’t buy a new Tesla if you’re enraged at Musk. The boycotters are correct that rejecting these vehicles directly hurts him. It drives down sales numbers, which hurts Tesla’s bottom line and saps the company’s stock price. Tesla’s remarkable valuation, buoyed as much by a cult of personality as by the company’s sales figures, has made Musk the world’s richest person: The company was worth three times more than Toyota in 2024, despite selling six times fewer vehicles. Even so, collapsing sales, not only in the U.S. but also across Europe and Australia, have wiped out billions of dollars from Tesla’s stock.
Buying a pre-owned Tesla might feel just as unseemly. But it’s not. Start with the sustainability question. Anti-Musk liberals would surely agree that more Americans should go electric to cut carbon emissions. Only about one in 10 registered vehicles in America is an EV, so it’s likely that a used-Tesla buyer will be replacing an old gas-burner. The switch might be permanent: More than 90 percent of EV owners say they won’t go back to combustion. For the most part, Tesla refugees aren’t retreating to the polluting purr of the V-6; they’re switching to electric cars from other brands, such as Chevy, Lucid, and Kia.
Used Teslas also help solve the main problem with getting Americans to go electric, which is price. Even with government tax credits, EVs tend to cost a premium compared with gas cars or hybrids. Pre-owned EVs, though, are shockingly affordable. Electric cars in general depreciate faster than gas-powered cars for a number of reasons, including fading battery life and used-car buyers’ unfamiliarity with the technology, Brian Moody, a senior staff editor at Kelley Blue Book, told me. All of this is bad for sellers but good for buyers.
[Read: America finally has the answer to the biggest problem with EVs]
Even before the Great Tesla Sell-Off, the bulk of used EVs were Teslas. The math doesn’t lie: Just five years ago, Tesla sold nearly 80 percent of the electric cars in America. Now that virtually every major car company offers EVs, Tesla’s dominance is waning, but most of those non-Tesla EVs have yet to reach their second owners. Used Teslas were already pretty affordable, but now they are getting even cheaper. Moody said the average transaction price for a used Tesla dipped from nearly $32,000 in November to about $30,400 in early March as more flooded the market. Tesla’s resale value is reportedly falling three times as fast as the rest of the used-car market.
Perhaps most important of all: Unless you purchase a used Tesla directly from the company, Musk isn’t getting your money. It’s possible to buy a pre-owned Tesla and avoid his other revenue streams too. Just like every carmaker, Tesla maintains a network of service centers to repair its vehicle, and because so few car mechanics specialize in electric vehicles, paying Tesla to do the work is much easier (and can feel safer) than taking a gamble on your neighborhood repair guy. However, a used car is likely to be past its four-year basic warranty, so you could take your old Model Y to an independent shop without voiding any coverage.
Then there is the question of charging. Tesla’s Supercharger network is admittedly excellent and convenient. But that is relevant for more than Tesla owners. Over the past couple of years, the rest of the industry has adopted Tesla’s plug standard, and many other brands’ EVs can now visit the company’s fast-charging stations. Musk-hating Rivian owners might still find themselves paying him for kilowatt-hours in a pinch. Still, avoiding them is easier than you might think. EV newbies tend to fret about charging, given that plugging in a car is still not as simple or quick as pulling into the nearest Shell. But the anxiety tends to be exaggerated. Something like 80 percent of EV owners primarily charge at home, which provides enough electricity for daily driving. On road trips, drivers can plan ahead to make a point of visiting charging stations that aren’t owned by Tesla.
Of course, a used Tesla may not spare you from getting shouted at while you’re getting groceries. Buying any kind of Tesla in 2025 can practically feel like an invitation to get graffitied, or at least a tacit endorsement of the brand. But set aside the optics—no simple task—and a pre-owned Tesla is just as climate-virtuous as all the Chevy Bolts and Ford Mustang Mach-Es that aren’t carrying around any MAGA baggage. Refusing an old Model 3 doesn’t hurt Elon or help the planet. But it does stop you from getting a good deal. If you’re still feeling trepidation, consider an apology bumper sticker: I bought it from someone who bought it before Elon was crazy.
Photographs by Kent Nishimura
On the first Sunday of spring, surrounded by row houses and magnolia trees, I came to a horrifying realization: My mom was right. I had been flipped off at least 17 times, called a “motherfucker” (in both English and Spanish), and a “fucking dork.” A woman in a blue sweater stared at me, sighed, and said, “You should be ashamed of yourself.” All of this because I was driving a Tesla Cybertruck.
I had told my mom about my plan to rent this thing and drive it around Washington, D.C., for a day—a journalistic experiment to understand what it’s like behind the wheel of America’s most hated car. “Wow. Be careful,” she texted back right away. Both of us had read the stories of Cybertrucks possibly being set on fire, bombed with a Molotov cocktail, and vandalized in every way imaginable. People have targeted the car—and Tesla as a whole—to protest Elon Musk’s role in Donald Trump’s administration. But out of sheer masochism, or stupidity, I still went ahead and spent a day driving one. As I idled with the windows down on a street in the Mount Pleasant neighborhood, a woman glared at me from her front porch: “Fuck you, and this truck, and Elon,” she yelled. “You drive a Nazi truck.” She slammed her front door shut, and then opened it again. “I hope someone blows your shit up.”
Earlier that day, my first stop was the heart of the resistance: the Dupont Circle farmers’ market. The people there wanted to see the organic asparagus and lion’s-mane mushrooms. What they did not want to see was a stainless-steel, supposedly bulletproof Cybertruck. Every red light created new moments for mockery. “You fucker!” yelled a bicyclist as he pedaled past me on P Street. The diners eating brunch on the sidewalk nearby laughed and cheered. Then came the next stoplight: A woman eating outside at Le Pain Quotidien gave me the middle finger for a solid 20 seconds, all without interrupting her conversation.
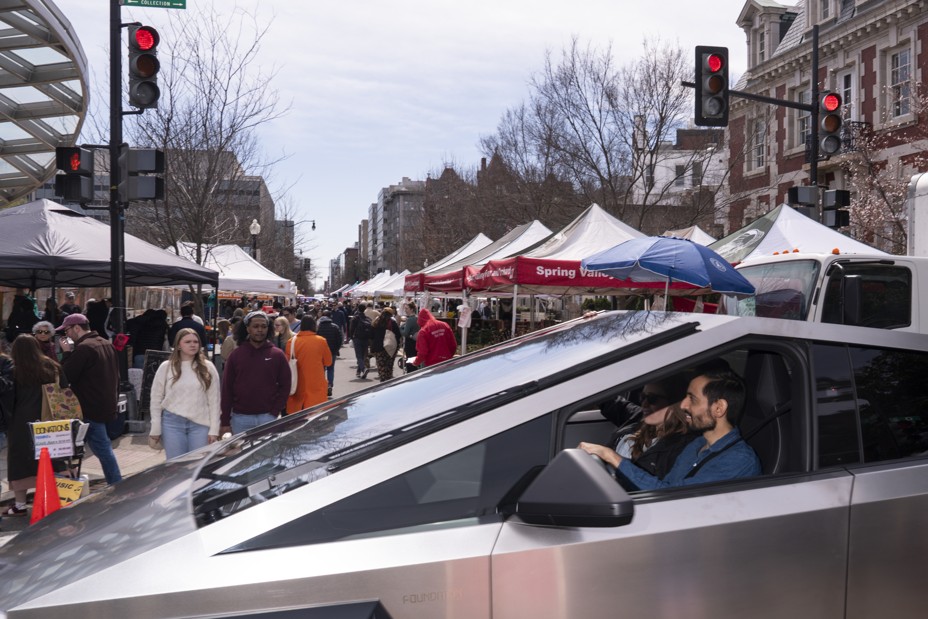
The anger is understandable. This is, after all, the radioactive center of DOGE’s blast radius. On the same block where I was yelled at in Mount Pleasant, I spotted a hand-drawn sign in one window: CFPB, it read, inside of a giant red heart; and at one point, I tailed behind a black Tesla Model Y with the bumper sticker Anti Elon Tesla Club. But the Cybertruck stands out on America’s roads about as much as LeBron James in a kindergarten classroom. No matter where you live, the car is a nearly 7,000-pound Rorschach test: It has become the defining symbol of the second Trump term. If you hate Trump and Musk, it is a giant MAGA hat, Pepe the Frog on wheels, or the “Swasticar.” If you love Trump and Musk, the Cybertruck is, well, a giant MAGA hat. On Monday, FBI Director Kash Patel called Tesla vandalism “domestic terrorism” as he announced a Tesla task force to investigate such acts. Alex Jones has trolled Tesla protesters from the back of his own Cybertruck, bullhorn in hand. Kid Rock has a Cybertruck with a custom Dukes of Hazzard paint job; the far-right podcaster Tim Pool owns one and says he’ll buy another “because it will own the libs”; and Kanye West has three. Trump’s 17-year-old granddaughter was gifted one by the president, and another by Musk.
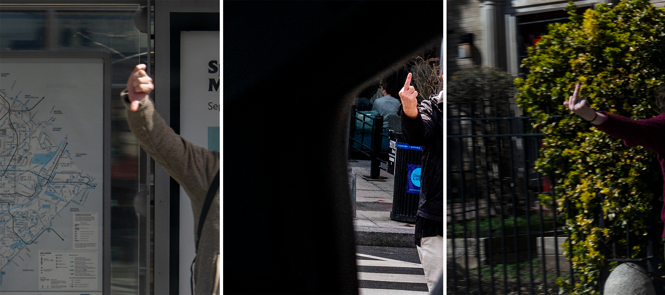
When I parked the car for lunch in Takoma Park, where I support federal workers signs were staked into the grass, I heard two women whispering at a nearby table: “Should we egg it?” (In this economy?) Over and over again, as pedestrians and drivers alike glared at me, I had to remind myself: It’s just a car. And it’s kind of a cool one, too. It can apparently outrace a Porsche 911, while simultaneously towing a Porsche 911. Or it can power a house for up to three days. My day in the Cybertruck wasn’t extremely hard-core, but the eight onboard cameras made city driving more bearable than I was expecting. Regardless of what you do with it, the car is emissions-free. “The underlying technology of the Cybertruck is amazing,” Loren McDonald, an EV analyst at the firm Paren, told me. And the exterior undersells just how ridiculous it is. Just before I returned the car on Monday morning, I took an impromptu Zoom meeting from the giant in-car touchscreen. It has a single windshield wiper that is so long—more than five feet—that Musk has compared it to a “katana.”
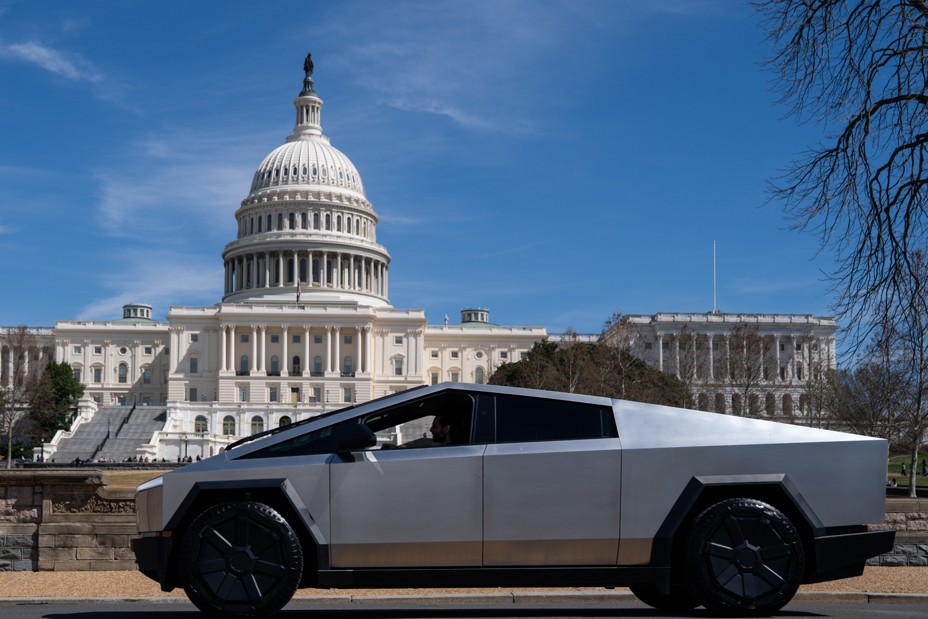
After 10 hours of near-constant hazing, I navigated to an underground parking lot to recharge the truck (and my battered self-image). Someone had placed a sticker just beneath the Tesla logo: Elon Musk is a parasite, it read. Still, even in D.C., I got a fair number of thumbs-ups as my Cybertruck zoomed by the areas most frequented by tourists. Near the National Mall, a man in a red bandanna and shorts yelled, “That’s awesome!” and cheered. Perhaps it was an attempt at MAGA solidarity, or maybe not. Lots of people just seemed to think it looked cool. One guy in his 20s, wearing a make money, not friends hoodie, frantically took out his phone to film me making a left turn. Even in the bluest neighborhoods of D.C.—near a restaurant named Marx Cafe and a Ruth Bader Ginsburg mural—kids could not get enough of the Cybertruck. One girl in Takoma Park saw me and started screaming, “Cybertruck! Cybertruck!” Later, a boy spotted the car and frantically rode his scooter to try to get a better look. Just before sunset, I was struggling to change lanes near George Washington University when two teens stopped to stare at me from the sidewalk. I was anxiously checking directions on my phone and clearly had no idea where to go. “Must be an Uber,” one said to the other.
[Read: Admit it, the Cybertruck is awesome]
By 9 p.m., I’d had enough. I valeted at my hotel, with its “Tibetan Bowl Sound Healing” classes, and got a nervous look from the attendant. I can’t blame anyone who sees the car as the stainless-steel embodiment of the modern right. This week, a county sheriff in Ohio stood in front of a green Cybertruck and derided Tesla vandals as “little fat people that live in their mom’s basement and wear their mom’s pajamas.” But it is also a tragedy that the Cybertruck has become the most partisan car in existence—more so than the Prius, or the Hummer, or any kind of Subaru. The Cybertruck, an instantly meme-able and very weird car, could have helped America fall in love with EVs. Instead, it is doing the opposite. The revolt against Tesla is not slowing down, and in some cases people are outright getting rid of their cars. Is it really a win that Senator Mark Kelly of Arizona exchanged his all-electric Tesla sedan for a gas-guzzling SUV?
Then again, Republicans aren’t buying the Cybertruck en masse. It is too expensive and too weird. Buying any Tesla might be a way to own the libs, but the right has proved maddeningly resistant to going electric. “Your average MAGA Trump supporter isn’t going to go buy a Tesla,” McDonald, the EV analyst, said. Before the car shipped in November 2023, Musk predicted that Tesla would sell 250,000 a year. He hasn’t even sold one-fifth of that in total—and sales are falling. (Neither Tesla nor Musk responded to a request for comment.)
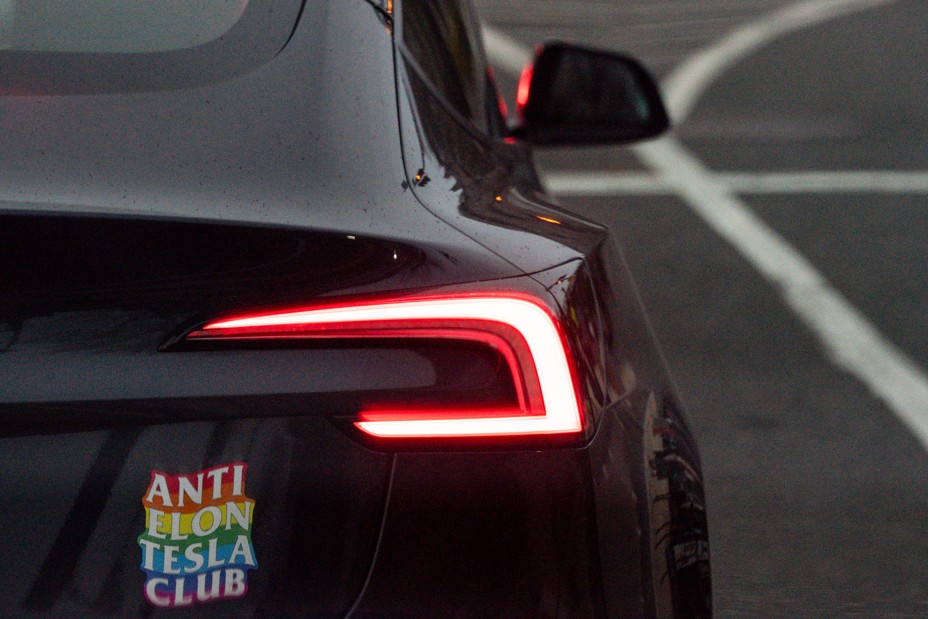
Musk made a lot of other promises that haven’t really panned out: The Cybertruck was supposed to debut at less than $40,000. The cheapest model currently available is double that. The vehicle, Musk said, would be “really tough, not fake tough.” Instead, its stainless-steel side panels have fallen off because Tesla used the wrong glue—and that was just the most recent of the car’s eight recalls. The Cybertruck was supposed to be able to haul “near infinite mass” and “serve briefly as a boat.” Just this month alone, one Cybertruck’s rear end snapped off in a test of its towing power, and another sank off the coast of Los Angeles while trying to offload a Jet Ski from the bed.
The Cybertruck, in that sense, is a perfect metaphor for Musk himself. The world’s richest man has a bad habit of promising one thing and delivering another. X was supposed to be the “everything app”; now it is a cesspool of white supremacy. DOGE was billed as an attempt to make the government more nimble and tech-savvy. Instead, the cuts have resulted in seniors struggling to get their Social Security checks. So far, Musk has only continued to get richer and more powerful while the rest of us have had to deal with the wreckage. Let that sink in, as he likes to say. The disaster of the Cybertruck is not that it’s ugly, or unconventional, or absurdly pointy. It’s that, for most people, the car just isn’t worth driving.
Sign up for Trump’s Return, a newsletter featuring coverage of the second Trump presidency.
On March 18, the official White House account on X posted two photographs of Virginia Basora-Gonzalez, a woman who was arrested earlier this month by U.S. Immigration and Customs Enforcement. The post described her as a “previously deported alien felon convicted of fentanyl trafficking,” and celebrated her capture as a win for the administration. In one photograph, Basora-Gonzalez is shown handcuffed and weeping in a public parking lot.
The White House account posted about Basora-Gonzalez again yesterday—this time, rendering her capture in the animated style of the beloved Japanese filmmaker Hayao Miyazaki, who co-founded the animation company Studio Ghibli. Presumably, whoever runs the account had used ChatGPT, which has been going viral this week for an update to its advanced “4o” model that enables it to transform photographs in the style of popular art, among other things. The White House did not respond directly to a request for comment, instead referring me to a post by Deputy Communications Director Kaelan Dorr that says, in part, “The arrests will continue. The memes will continue.”
It’s worth pausing here: The internet has been flooded with AI-generated images in this exact Studio Ghibli style. Some people have used it for images of pets or family members. Others opted for a trollish register, leading ChatGPT to spit out cutesy renderings of JFK’s assassination, planes hitting the World Trade Center, and the torture at Abu Ghraib. On X, the prevalence of these images became an event unto itself, one in which the White House decided to participate by sharing a cartoon of a woman crying in handcuffs.
This is how the White House account operates now. In previous administrations (including much of Donald Trump’s first term), the account was used to post anodyne updates, highlight press releases, and share information about the administration. It was, to be fair, often painfully dull or written in the stilted language of a brand. Now the account exists to troll its political enemies and delight the MAGA faithful.
[Read: The internet is worse than a brainwashing machine]
On Wednesday, the account posted a picture of Vice President J. D. Vance shooting a tactical rifle, referring to the bullets he fired as “freedom seeds,” a term popular among gun YouTubers. When Google Maps adopted the “Gulf of America” language pushed by the administration, the White House account celebrated by sharing a video in which the words Gulf of Mexico are wiped off the globe. In February, it posted an AI-generated picture of Trump as an American monarch, wearing a crown. The image’s caption reads, “Long live the king.” After the disastrous Oval Office ambush of Ukrainian President Volodymyr Zelensky, the account posted a photo of Vance staring at Zelensky with the caption “Have you said thank you once?” Although the account sometimes shares actual news, it’s frequently preoccupied by rapid-response engagement bait for MAGA diehards. Less information, more content. The intent is not to inform but to go viral.
Beyond the fact that this kind of shitposting is so obviously beneath the office, the posts are genuinely sinister. By adding a photo of an ICE arrest to a light-hearted viral trend, for instance, the White House account manages to perfectly capture the sociopathic, fascistic tone of ironic detachment and glee of the internet’s darkest corners and most malignant trolls. The official X account of the White House isn’t just full of low-rent 4chan musings, it’s an alarming signal of an administration that’s fluent in internet extremism and seemingly dedicated to pursuing its casual cruelty as a chief political export.
To be clear, the actions of the second Trump administration—the dismantling of the federal government via DOGE, the apprehension and detainment of immigrants and green-card holders with seemingly no due process—are of far more consequence than what it posts on social media. But White House posts are not random missives either: They’re official government communications from the executive branch, sent out to 1.4 million followers, to say nothing of whatever additional reach these posts receive via algorithmic recommendation and ad hoc sharing.
The account’s true obsession is immigration: @WhiteHouse has posted dozens of mugshots of immigrants arrested by ICE. Each one lists an offense they’ve been arrested for in big block letters, and usually the catchphrase “MAKE AMERICA SAFE AGAIN” is appended. Earlier this month, after the Department of Homeland Security commented on the deportation of a Lebanese professor at Brown University—a violation of a court order temporarily protecting her from expulsion—the White House account responded by posting a photo of Trump waving goodbye from a McDonald’s drive-through window. (The picture was taken during a 2024 campaign stunt.) On Valentine’s Day, the account wrote, “Roses are red / Violets are blue / Come here illegally / And we’ll deport you.”
And in an infamous example, on February 18, the White House account posted a 41-second video of faceless men being shackled and marched onto planes. The post’s caption read, “ASMR: Illegal Alien Deportation Flight.” (ASMR is short for “autonomous sensory meridian response,” or the titillating sensation caused by certain noises, such as whispering, tapping, or crinkling; videos of people making such noises are enormously popular across social media.) The subtext of the White House post is far from subtle and is reminiscent of something out of 4chan’s notoriously bigoted politics message board: Watching allegedly undocumented immigrants bound in chains is a pleasurable, even sensual experience. Like any trolling post, it’s meant to be simultaneously taken seriously and played off as a dumb online joke. Even those inside the Trump administration seemed taken aback by the audacity of the post. Even some MAGA supporters appeared uncomfortable by @WhiteHouse’s brazenness. “If you guys could stick with the grim shock and awe, and leave the edgy gloating to those of us who don’t work in the White House I think that would probably be better for optics,” one user wrote on X. The “ASMR” deportation video, as of this writing, has been viewed almost 104 million times on X.
Exactly who is running the White House X account is an object of fascination for close observers. Some accounts fantasize that Trump’s college-age son, Barron, is running it. Those outside of Trump fandom have insisted that it is being run by edgelords—one post referred to the operator as an “incel reddit user.” One Bluesky user described the account as “lowkey goebbelsmaxxing,” a reference to the Nazi propagandist. (The White House did not respond to a request to identify who writes the account’s posts.) What all the speculation suggests is that at least someone with access to the account is intimately familiar with far-right internet spaces and culture, specifically Groypers, a term for the loose online movement that has succeeded the alt-right. Earlier this year, the writer John Ganz argued that “Groyperism totally suffuses the cultural environment of the right.” He and others have suggested that the culture is present in the offices of Republican representatives in Washington, D.C., including in the White House. (A Trump staffer was fired in the first administration for associating with white nationalists; he’s now back, in a role at the State Department.) Although the identities of whoever is running the account are, at present, unknown, what’s clear is that their output appears to delight prominent extremists online. The message coming from the account, to borrow language from one far-right X user, is clear: The posters are in control.
[Read: X is a white-supremacist site]
And the posters have goals. The first is to engage and supply their loyal audiences with constant memes and content. The second is perhaps more strategic. The account’s blatant humiliation of immigrants who it alleges have heinous criminal records is intentional. The goal is to goad their opponents into defending people accused of indefensible crimes. The primary accusation from the MAGA faithful toward people who are outraged about the White House’s Studio Ghibli post or the ASMR video is that the left is more concerned with defending fentanyl dealers and immigrants accused of rape and robbery than they are about the safety of the country. “Disappointing that folks are more upset about this meme than they are about the fentanyl crisis,” Dorr said in the same post that the White House pointed me to. But this is a false binary; in all cases, the chief objections are to the dehumanization and glee on display and the worrying lack of due process.
The White House is after something more than just shock value. It’s propaganda, and Trump’s allies are learning the playbook. This week, Department of Homeland Security Secretary Kristi Noem posted a video on X from a prison in El Salvador where deported immigrants are being held. Behind Noem are dozens of men in one jail cell, many shirtless with tattoos; their heads have all been shaved during intake. The prisoners are props, a backdrop for Noem’s message of intimidation to undocumented immigrants: “If you do not leave, we will hunt you down, arrest you, and you could end up in this El Salvadorian prison.” Like the ASMR post or the Ghibli cartoon, the implication is that these deportees are utterly undeserving of any shred of human dignity. There are many other examples, such as FBI Director Kash Patel’s recent posts, one of which features him walking around in camouflage, set to rock music, as FBI agents blow open doors with explosives. Taken together, the posts offer a bracing but useful insight into how the administration sees itself, and the message of casual cruelty and overwhelming force it wants to project to the rest of the world.
That this administration should fully embrace the tactics and aesthetics of online far-right extremists and technological tools like generative AI to further its message makes perfect sense. These are reliable ways to increase engagement, gain attention, and illustrate a precise vision of the future they want to usher in. Even so, the account is chilling. Those who’ve spent enough time in the online spaces that have clearly influenced this administration—or at least whoever runs its social accounts—know how this goes. This is a game of accelerationism and nihilism, using tools and platforms that excel at depersonalizing, thus rendering empathy for others ever more difficult. That this sociopathic posting style is coming out of this administration—that it has been so thoroughly mainstreamed by the right—suggests that the cultural architecture of the internet has changed. There is still a fever swamp, but now the White House sits on top of it.
In 1962, the CIA had a driver’s license made for one of its officers, James P. O’Connell. It gave him an alias: James Paul Olds. We know this because the document containing the information was released to the public in 2017—part of an effort to declassify information related to John F. Kennedy’s assassination. But now, thanks to an executive order from President Donald Trump calling for the release of all the classified information pertaining to the incident, we know a bit more. It was, specifically, a California driver’s license.
This is an irrelevant detail in an irrelevant document. As far as anyone knows, O’Connell had nothing to do with the assassination; the inclusion of his story was probably just a by-product of an overly broad records request. But there it was on Tuesday evening, when the National Archives and Record Administration uploaded to its website about 63,400 pages of “JFK Assassination Records.” Given Trump’s order, the release of all this information sounded dramatic, but much of what has been revealed is about as interesting as that driver’s-license detail. Many of these documents were already public with minor redactions, and many of them have almost nothing to do with the Kennedy assassination and never did. This is why the Assassination Records Review Board, which processed them in the 1990s, labeled so many of them “Not Believed Relevant.”
Hundreds of thousands of such documents have been released since the ’90s, including thousands released during Trump’s first term and the Biden administration. (This is thanks to the President John F. Kennedy Assassination Records Collection Act of 1992, which was passed in response to overwhelming public interest in the case after the release of the Oliver Stone movie JFK.) But one of Trump’s 2024 campaign promises was to release all the rest; he said that it was “time for the American people to know the TRUTH!” His health secretary, Robert F. Kennedy Jr.—John Kennedy’s nephew—has been animated about the issue and framed the secrecy around the last files as evidence to support his conspiratorial view of history.
[Read: RFK Jr. won. Now what?]
There are still some documents that the Archives could not make public, because they are subject to IRS privacy laws or because they come from sealed grand-jury proceedings. These may come out eventually, but they will likely follow the same drip, drip, drip as all the rest. It seems possible that the public’s curiosity will never be fully satisfied, at least in my lifetime. A new batch will always come out, but there will always be something left.
I’m one of the people who cares a lot about the Kennedy assassination. I’m currently finishing a book about the case. On principle, and out of selfish personal interest, I agree that the government should make all of the documents public if it can. Of course I scanned this new batch to see whether there was anything exciting. There wasn’t, but some of it was kind of funny.
In many cases, the removed redactions reveal proper nouns that a reader could have easily inferred before or that seem totally inconsequential. For instance, there is a 1974 memo about the Watergate conspirator E. Howard Hunt’s history with the CIA. A previously released version of the document mentions that the Office of Finance had asked a CIA station whether Hunt had received payments from it while he was living in Madrid. We did not know which station had been asked. Now we know it was the Madrid station. (Wow!) A 1977 document about the New York Times reporter Tad Szulc includes a rumor about Szulc being a Communist; in previous versions of the document, this information was “apparently from a [REDACTED] source.” With the redaction removed, we now know that it was “apparently from a British source.”
Some of it was less funny. The files also contain the unredacted personal information—including Social Security numbers—of dozens of people, seemingly published accidentally, though the National Archives site now suggests this was an inevitable result of the transparency effort. White House Press Secretary Karoline Leavitt acknowledged the problem to The New York Times on Thursday, saying, “At the request of the White House, the National Archives and the Social Security Administration immediately put together an action plan to proactively help individuals whose personal information was released in the files.” The National Archives did not respond to my request for comment.
In my scan, I came across the late-’70s personnel files of dozens of staff members of the House Select Committee on Assassinations, all of which contained Social Security numbers. A good number of those people are likely still alive. The document dump contains the Social Security number of a journalist who was active in the anti-war movement during the ’60s. There are, by my count, 19 documents about his personal life and employment history; none of the documents about him appears to have the faintest relevance to the assassination. Bizarrely, the new release also contains an unredacted arrest record for a Dealey Plaza witness who testified in front of the Warren Commission in 1964. This record—for the alleged theft of a car in 1970—has nothing whatsoever to do with the assassination of President Kennedy. Yet it is reproduced in full and it includes the man’s Social Security number and a full set of his fingerprints.
[From the February 1964 issue: A eulogy for John F. Kennedy]
Relatively few of the documents even mention Kennedy. I saw only one addressed to him: a June 30, 1961, memo from his special assistant, confidant, and eventual biographer, Arthur Schlesinger about the growing power of the CIA. Most of it has been public since 2018, but the version released on Tuesday removed a final redaction about the agency’s extensive use of State Department jobs as cover for its agents. Schlesinger informed Kennedy that about 1,500 CIA agents abroad had State-provided cover stories at the time—too many, in his opinion; he wrote that “the effect is to further the CIA encroachment on the traditional functions of State.” The Paris embassy had 128 CIA people in it at the time, he added as an example. “CIA occupies the top floor of the Paris Embassy, a fact well known locally; and on the night of the Generals’ revolt in Algeria, passersby noted with amusement that the top floor was ablaze with lights.” Again, this is at best “kind of interesting” and at most trivia. It doesn’t meaningfully affect the historical understanding of President Kennedy’s tense relationship with the CIA, which is very well documented elsewhere.
After decades of releases, it may be that these are the only kinds of secrets the Archives still hold about the Kennedy assassination—tiny bits of color on things that are already well understood and boring details about people whose connections to the event are minimal if they even exist. But there’s no way to know until we see everything … if we see everything, if we ever can. Even then, when the count of secret things ticks down to zero, how will we know that was really, really all? We won’t, of course. We never will.
Perhaps the biggest surprise of Careless People, the new tell-all memoir by the former Facebook executive Sarah Wynn-Williams, is that a book chronicling the social network’s missteps and moral bankruptcy can still make news in 2025.
The tech giant—now named Meta—seems determined to make this happen itself. The company filed an emergency motion in court to halt the book’s continued publication, and in numerous statements, Meta’s communications team has derided it as the work of a disgruntled ex-employee. All of this has only generated interest: On Thursday, the book debuted at the top of the New York Times best-seller list for nonfiction and, as of this writing, was the third-best-selling book on Amazon.
A general theme of the pushback is that Wynn-Williams, who worked on global policy at Facebook, is guilty of the same sins she documents in the book. “Not only does she fail to take any responsibility for her role in all of this,” Katie Harbath, a former director of public policy at Facebook wrote on her Substack, “but she is also careless in her account. She also gives no recommendations on how to do things better other than to say they should be done differently.” Andy Stone, a spokesperson for Meta, called the book “a mix of old claims and false accusations about our executives.” He has also shared posts from current and former employees that cast Wynn-Williams as an unreliable narrator. In one post, a former colleague expresses frustration that the book seems to take credit for his efforts at the company.
Given Wynn-Williams’s privileged position as Facebook’s first executive focused specifically on global policy, her perspective might differ from that of employees on other teams. Meta is a huge organization, after all. But the debates over the more gossipy anecdotes obscure the larger trends that surface through the book. I’ve never spoken with Wynn-Williams for a story or otherwise—she is currently under a gag order after Meta pursued legal action against her, claiming that the book violates her nondisclosure agreement—but her descriptions of Facebook taking actions in foreign countries without regard for consequences are similar to anecdotes told to me over the years by current and former employees. These stories are even more relevant in 2025, when tech’s most powerful figures have assumed an outsize role in American politics. All of us are living in a world that’s been warped by the architecture and algorithms of Silicon Valley’s products, but also by the egos of the people who have made fortunes building them.
I tore through Careless People despite being intimately familiar with many of the broad storylines—Facebook’s push into politics and the fallout from the 2016 election, its global efforts to expand in China, the platform’s bungled expansion in Myanmar that contributed to a genocide in the region. Wynn-Williams’s perspective provides crucial dimensionality to a well-trodden story, given her proximity to the company’s leadership.
But although it explores serious subject matter, the book is also not nearly as strident or sanctimonious as some other whistleblower memoirs. Wynn-Williams is comfortable reaching for an absurdist register: She recounts, for example, a scheduling nightmare that brought her to the brink of tears while trying to get Mark Zuckerberg a last-minute spot at the Global Citizen Festival in 2015: In her attempts, Wynn-Williams manages to anger an actor dressed as Big Bird and create logistical “issues for Malala and Beyoncé.” The spectacle culminates with a sweaty Zuckerberg onstage, “looking around desperately, like an animal in a trap.” The book is filled with similar anecdotes that capture the peculiar indignities of those catering to the whims of the most powerful people in the world. (When reached for comment, Dave Arnold, a spokesperson for Meta, referred to past statements the company has made about the book and cast doubt on Wynn-Williams’s status as a whistleblower: “Whistleblower status protects communications to the government, not disgruntled activists trying to sell books.”)
Early in Careless People, Wynn-Williams says Facebook asked her if “Mark should take credit for the Arab Spring.” In passages about Facebook’s expansion in Myanmar, she cites the executive team’s incuriosity about the country’s culture and politics. Later, when viral fake news stories on Facebook lead to riots and killings in Myanmar, Wynn-Williams details that the company had just one moderator who spoke Burmese, never posted its community standards in Burmese, and did not translate core navigation features of the platform into Burmese, including the button you use to report hateful content. News coverage in the aftermath of the genocide supported many of Wynn-Williams’s claims.
Wynn-Williams offers a few explanations for these problems over the course of Careless People. She suggests that executives like Zuckerberg simply don’t care about Facebook users once they’re on the platform. But there is also the company’s relentless pursuit of growth. Facebook’s obsession with gaining access to Myanmar and other Southeast Asian countries came around the same time that Facebook’s growth and stock were flagging post-IPO. Internally, stagnant user growth was referred to as “running out of road.” She describes Facebook’s growth team as willing to do almost anything to extend that road.
Nearly every insight and example provided in Careless People—the allegations that former Facebook COO Sheryl Sandberg and the Irish prime minister secretly schemed on ways to dodge corporate taxes, the documentation of Facebook’s attempts to work with China to collect data on its citizens—traces back in one form or another to a blind obsession with scaling the business. All of it, of course, is meant to achieve Zuckerberg’s vague yet relentless mission to connect the world. When asked about these allegations, Arnold, the Meta spokesperson, told me, “We do not operate our services in China today. It is no secret we were once interested in doing so as part of Facebook’s effort to connect the world.”
[Read: New Mark Zuckerberg dropped]
Wynn-Williams’s assessment of Facebook’s mission aligns with what I know. In 2018, while reporting for BuzzFeed News, my colleagues and I obtained a memo written by Andrew Bosworth, one of Zuckerberg’s most loyal executives, outlining his personal strategy of growth at any cost. In the memo, Bosworth suggests that people could get hurt or killed as a result of Facebook’s expansions. Still, he is unequivocal: “The ugly truth is that we believe in connecting people so deeply that anything that allows us to connect more people more often is *de facto* good.” This same tone of self-assuredness, short-sightedness, and binary thinking are present in most of the conversations with Facebook executives that Wynn-Williams writes about (on Threads, Bosworth called Careless People “full of lies. Literally stories that did not happen”). It’s captured memorably early on in the book, when Wynn-Williams details the company’s young policy team’s struggle to come up with a mission statement of its own:
[For] Mark and Sheryl, it’s obvious. We run a website that connects people. That’s what we believe in. We want more. We want it to be profitable and to grow. What else is there to say? There is no grand ideology here. No theory about what Facebook should be in the world. The company is just responding to stuff as it happens. We’re managers, not world-builders. Marne just wants to get through her inbox, not create a new global constitution.
Reading Careless People, I became fixated on a question: What is left to say about Facebook? The company has been through more than a decade of mega-scandals, congressional hearings, apologies, and Zuckerbergian heel turns. Many people have experienced the ways that the platform has algorithmically warped and influenced our culture, politics, and personal relationships. It is difficult to say something new about the company that has, in large part, succeeded in connecting the world. A book revealing, in 2025, that Facebook has behaved recklessly or in morally reprehensible ways feels akin to arguing at length that oil companies are substantially responsible for climate change—almost too obvious to be very interesting.
And yet, something about Careless People—beyond the court order, the messy PR spectacle, and Wynn-Williams’s formidable storytelling abilities—feels urgent, even necessary right now. It’s not just that Zuckerberg is in the news for cozying up to Donald Trump, though that’s part of it. Paging through the book makes clear that even recent history rhymes with the present. Careless People is a memoir, but even Wynn-Williams’s most personal anecdotes speak to the power and authority that tech executives, their platforms, and their massive fortunes wield over so many.
American politics, in the second Trump administration especially, is as much a tech story as it is a political one. Elon Musk’s dismantling of the federal government via DOGE is a product of the same Silicon Valley ideology that Zuckerberg coined with his infamous “move fast and break things” motto. Similarly, Musk’s self-described obsession with rooting out waste, fraud, and abuse to make the government efficient shares a platitudinal vagueness with Zuckerberg’s long-standing mission to connect the world. Both ideas sound good on paper but are ultimately poorly defined (and executed even worse), leaving the same question unanswered: connection for what? Efficiency at what cost?
[Read: The rise of techno-authoritarianism]
Careless People illustrates how this ideological vacuum is filled by its leaders’ fleeting whims and governed by their fragile egos. Zuckerberg, whose “disregard for politics is a point of pride” at the beginning of the book, is ultimately enamored by the power it brings. Slowly, Wynn-Williams notes, he becomes more involved in global affairs, eventually asking to make complex content-moderation decisions on his own. “In reality it’s just Mark,” she writes. “Facebook is an autocracy of one.”
The chaos of the past two months—the looming constitutional crises, the firings and rehirings—is what it feels like when a government is run, at least in spirit, like a technology company. Wynn-Williams’s book isn’t prescient; much of it is, as Meta notes, older news. What’s most disorienting about Careless People is that it is packaged as a history of sorts, but its real utility to the reader is as a window into our current moment, a field guide to tech autocracy.
In her epilogue, Wynn-Williams notes of the Facebook executives that “the more power they grasp, the less responsible they become.” Does that sound familiar? For now, the careless people have won. They’re in charge. The chaos Wynn-Williams has documented—we’re just living through a different version of it.
Editor’s note: This search tool is part of The Atlantic’s investigation into the Library Genesis data set. You can read an analysis about LibGen and its contents here. Find The Atlantic’s search tool for movie and television writing used to train AI here.
Disclaimer: LibGen contains errors. You may, for example, find books that list incorrect authors. This search tool is meant to reflect material that could be used to train AI programs, and that includes material containing mistakes and inaccuracies.
It’s impossible to know exactly which parts of LibGen Meta used to train its AI, and which parts it might have decided to exclude; this snapshot was taken in January 2025, after Meta is known to have accessed the database, so some titles here would not have been available to download.
Updated at 5:40 p.m. ET on March 21, 2025
Editor’s note: This analysis is part of The Atlantic’s investigation into the Library Genesis data set. You can access the search tool directly here. Find The Atlantic’s search tool for movie and television writing used to train AI here.
When employees at Meta started developing their flagship AI model, Llama 3, they faced a simple ethical question. The program would need to be trained on a huge amount of high-quality writing to be competitive with products such as ChatGPT, and acquiring all of that text legally could take time. Should they just pirate it instead?
Meta employees spoke with multiple companies about licensing books and research papers, but they weren’t thrilled with their options. This “seems unreasonably expensive,” wrote one research scientist on an internal company chat, in reference to one potential deal, according to court records. A Llama-team senior manager added that this would also be an “incredibly slow” process: “They take like 4+ weeks to deliver data.” In a message found in another legal filing, a director of engineering noted another downside to this approach: “The problem is that people don’t realize that if we license one single book, we won’t be able to lean into fair use strategy,” a reference to a possible legal defense for using copyrighted books to train AI.
This article was featured in the One Story to Read Today newsletter. Sign up for it here.
Court documents released last night show that the senior manager felt it was “really important for [Meta] to get books ASAP,” as “books are actually more important than web data.” Meta employees turned their attention to Library Genesis, or LibGen, one of the largest of the pirated libraries that circulate online. It currently contains more than 7.5 million books and 81 million research papers. Eventually, the team at Meta got permission from “MZ”—an apparent reference to Meta CEO Mark Zuckerberg—to download and use the data set.
This act, along with other information outlined and quoted here, recently became a matter of public record when some of Meta’s internal communications were unsealed as part of a copyright-infringement lawsuit brought against the company by Sarah Silverman, Junot Díaz, and other authors of books in LibGen. Also revealed recently, in another lawsuit brought by a similar group of authors, is that OpenAI has used LibGen in the past. (A spokesperson for Meta declined to comment, citing the ongoing litigation against the company. In a response sent after this story was published, a spokesperson for OpenAI said, “The models powering ChatGPT and our API today were not developed using these datasets. These datasets, created by former employees who are no longer with OpenAI, were last used in 2021.”)
Until now, most people have had no window into the contents of this library, even though they have likely been exposed to generative-AI products that use it; according to Zuckerberg, the “Meta AI” assistant has been used by hundreds of millions of people (it’s embedded in Meta products such as Facebook, WhatsApp, and Instagram). To show the kind of work that has been used by Meta and OpenAI, I accessed a snapshot of LibGen’s metadata—revealing the contents of the library without downloading or distributing the books or research papers themselves—and used it to create an interactive database that you can search here:
There are some important caveats to keep in mind. Knowing exactly which parts of LibGen that Meta and OpenAI used to train their models, and which parts they might have decided to exclude, is impossible. Also, the database is constantly growing. My snapshot of LibGen was taken in January 2025, more than a year after it was accessed by Meta, according to the lawsuit, so some titles here wouldn’t have been available to download at that point.
LibGen’s metadata are quite disorganized. There are errors throughout. Although I have cleaned up the data in various ways, LibGen is too large and error-strewn to easily fix everything. Nevertheless, the database offers a sense of the sheer scale of pirated material available to models trained on LibGen. Cujo, The Gulag Archipelago, multiple works by Joan Didion translated into several languages, an academic paper named “Surviving a Cyberapocalypse”—it’s all in here, along with millions of other works that AI companies could feed into their models.
Meta and OpenAI have both argued in court that it’s “fair use” to train their generative-AI models on copyrighted work without a license, because LLMs “transform” the original material into new work. The defense raises thorny questions and is likely a long way from resolution. But the use of LibGen raises another issue. Bulk downloading is often done with BitTorrent, the file-sharing protocol popular with pirates for its anonymity, and downloading with BitTorrent typically involves uploading to other users simultaneously. Internal communications show employees saying that Meta did indeed torrent LibGen, which means that Meta could have not only accessed pirated material but also distributed it to others—well established as illegal under copyright law, regardless of what the courts determine about the use of copyrighted material to train generative AI. (Meta has claimed that it “took precautions not to ‘seed’ any downloaded files” and that there are “no facts to show” that it distributed the books to others.) OpenAI’s download method is not yet known.
Meta employees acknowledged in their internal communications that training Llama on LibGen presented a “medium-high legal risk,” and discussed a variety of “mitigations” to mask their activity. One employee recommended that developers “remove data clearly marked as pirated/stolen” and “do not externally cite the use of any training data including LibGen.” Another discussed removing any line containing ISBN, Copyright, ©, All rights reserved. A Llama-team senior manager suggested fine-tuning Llama to “refuse to answer queries like: ‘reproduce the first three pages of “Harry Potter and the Sorcerer’s Stone.”’” One employee remarked that “torrenting from a corporate laptop doesn’t feel right.”
It is easy to see why LibGen appeals to generative-AI companies, whose products require huge quantities of text. LibGen is enormous, many times larger than Books3, another pirated book collection whose contents I revealed in 2023. Other works in LibGen include recent literature and nonfiction by prominent authors such as Sally Rooney, Percival Everett, Hua Hsu, Jonathan Haidt, and Rachel Khong, and articles from top academic journals such as Nature, Science, and The Lancet. It includes many millions of articles from top academic-journal publishers such as Elsevier and Sage Publications.
[Read: These 183,000 books are fueling the biggest fight in publishing and tech]
LibGen was created around 2008 by scientists in Russia. As one LibGen administrator has written, the collection exists to serve people in “Africa, India, Pakistan, Iran, Iraq, China, Russia and post-USSR etc., and on a separate note, people who do not belong to academia.” Over the years, the collection has ballooned as contributors piled in more and more pirated work. Initially, most of LibGen was in Russian, but English-language work quickly came to dominate the collection. LibGen has grown so quickly and avoided being shut down by authorities thanks in part to its method of dissemination. Whereas some other libraries are hosted in a single location and require a password to access, LibGen is shared in different versions by different people via peer-to-peer networks.
Many in the academic world have argued that publishers have brought this type of piracy on themselves, by making it unnecessarily difficult and expensive to access research. Sci-Hub, a sibling of LibGen, was launched independently in 2011 by a Kazakhstani neuroscience student named Alexandra Elbakyan, whose university didn’t provide access to the big academic databases. In that same year, the hacktivist Aaron Swartz was arrested after taking millions of articles from JSTOR in an attempt to build a similar kind of library.
Publishers have tried to stop the spread of pirated material. In 2015, the academic publisher Elsevier filed a complaint against LibGen, Sci-Hub, other sites, and Elbakyan personally. The court granted an injunction, directed the sites to shut down, and ordered Sci-Hub to pay Elsevier $15 million in damages. Yet the sites remained up, and the fines went unpaid. A similar story played out in 2023, when a group of educational and professional publishers, including Macmillan Learning and McGraw Hill, sued LibGen. This time the court ordered LibGen to pay $30 million in damages, in what TorrentFreak called “one of the broadest anti-piracy injunctions we’ve seen from a U.S. court.” But that fine also went unpaid, and so far authorities have been largely unable to constrain the spread of these libraries online. Seventeen years after its creation, LibGen continues to grow.
[Read: There’s no longer any doubt that Hollywood writing is powering AI]
All of this certainly makes knowledge and literature more accessible, but it relies entirely on the people who create that knowledge and literature in the first place—that labor that takes time, expertise, and often money. Worse, generative-AI chatbots are presented as oracles that have “learned” from their training data and often don’t cite sources (or cite imaginary sources). This decontextualizes knowledge, prevents humans from collaborating, and makes it harder for writers and researchers to build a reputation and engage in healthy intellectual debate. Generative-AI companies say that their chatbots will themselves make scientific advancements, but those claims are purely hypothetical.
One of the biggest questions of the digital age is how to manage the flow of knowledge and creative work in a way that benefits society the most. LibGen and other such pirated libraries make information more accessible, allowing people to read original work without paying for it. Yet generative-AI companies such as Meta have gone a step further: Their goal is to absorb the work into profitable technology products that compete with the originals. Will these be better for society than the human dialogue they are already starting to replace?
This article has been updated to include a comment from OpenAI.
Forever 21 opened in my hometown when I was in middle school, when the opening of a new store at the mall was still a big deal. When the sign first went up, nobody knew what “Forever 21” was. I remember thinking that it would be a store marketed to retirement-age women who felt young at heart—Forever 21! This was wrong, but not so far off: Do Won Chang, one of its founders, has said he chose the name because 21 is “the most enviable age.” And it is, especially if you are 13, 14, 15, 16, 17, 18, 19, or 20, which are the ages at which I bought most of my clothes there. I can still smell the polyester and hear the White Rabbits on the sound system.
The clothes were, in theory, inexpensive versions of the latest trends. In practice, they were usually inexpensive versions of recently passed trends, and sometimes they were items so odd that they seemed to have been imported from a different reality (crop top with suspenders; Cheetos bathing suit). But for a suburban teenager with a little bit of cash, the store still felt like a place of possibilities. Yes, Forever 21 was always a disaster: Its racks and racks of cheap merchandise were “arranged” according to surrealist organizing principles that were impossible for the amateur to comprehend, and much of what was for sale was hideous. But I did not have taste—what I had was an after-school job in the mall food court that paid $7.25 an hour. I received paychecks every other week of about $150 that I could “save for college,” or that I could spend on statement necklaces and bubble skirts.
[Read: Will Americans ever get sick of cheap junk?]
I think of that store fondly, and now it is closing. All of the Forever 21 stores remaining in the U.S. are expected to close as well. The company filed for bankruptcy this week—not for the first time, although this one is truly the end. “We have been unable to find a sustainable path forward, given competition from foreign fast fashion companies,” Brad Sell, the company’s CFO, said in a statement. He also mentioned “rising costs, economic challenges impacting our core customers, and evolving consumer trends.”
Forever 21 grew rapidly in the 2010s, opening hundreds of stores—many of them in enormous spaces—and reporting billions in annual sales. For a solid decade, Forever 21 was a common feature of the American landscape. At that time, finding a Forever 21 seemed as easy as finding a library. The highlighter-yellow shopping bags were an indelible symbol of the accessibly cool Millennial lifestyle. Some Forever 21 locations even became true destinations. For myself and many of my classmates, the four-story Forever 21 in Times Square was the most exciting part of our senior-class trip to New York City—not joking!
Now the 20- and 30-somethings who learned to shop by wading through their local Forever 21 locations are mourning the brand and marking the “end of an era.” You can find many “RIP” comment threads right now in regional Reddit forums, where people often discuss their local malls. Most are lighthearted (“I guess they turned 22”). Nobody is in a state of shock, because there is obviously not a place in the culture anymore for something like Forever 21.
Today’s young people still like in-person shopping, but they like to do it in secondhand and thrift shops or, at the other end of the spectrum, in gorgeous stores that make shopping feel like an Instagrammable excursion. They still like fast fashion too, but they like it faster than Forever 21 does it—online-only brands such as Shein, Fashion Nova, and ASOS can turn out hundreds or even thousands of new styles every week and sell them at unbelievably low prices.
[Read: The mysterious, meteoric rise of Shein]
In the past several years, cultural awareness has grown about the reality of the fast-fashion business model, which relies on paying factory workers shockingly low wages and takes a multifaceted approach to furthering the ruination of the planet. (Barely used clothes pile up in landfills; synthetic fabrics shed microplastics into the water supply; the manufacturing of polyester drives greenhouse-gas emissions up and up.)
Today, young people shop fast fashion in spite of themselves. They know it’s bad, but they’re accustomed to convenience and limitless choice, so they do it anyway. Maybe the difference is that now they can buy it from their phone, which is less uncomfortable than looking at it all piled up around them in one physical place. When Forever 21 declared bankruptcy for the first time, six years ago, a retail consultant suggested something like this to The New York Times. “The emotional and physical aesthetic of it is not something that the current shopper wants as much,” WSL Strategic Retail’s Wendy Liebmann told the paper. You can’t go home again, and it can’t be 2010 again.
As an adult, I now realize that the items I impulse-bought at Forever 21 on my 15-minute breaks were (1) contributing to human misery and (2) not worth even the paltry sums I paid for them. I almost never got substantial use out of anything, either because the items were poorly made and fell apart or because I quickly realized that they were ugly and unflattering. The only purchase I remember clearly is a dress I picked out to wear as a bridesmaid in a high-school friend’s wedding, for which I paid $12. I thought it was genuinely chic; I couldn’t believe my luck, and I was impressed with myself for pulling a needle from a galaxy-size hayfield.
But I wore it only once, and I suppose it is in a landfill now. There, it will last a long, long time. Not forever, but much longer than Forever 21 did.
I need you to watch this 13-second video of ESPN commentator Stephen A. Smith walking to his courtside seat at a Los Angeles Lakers game. I need you to notice how Smith, perhaps the biggest voice in sports—in sheer decibels, if not reach—savors the see-and-be-seen pleasures of the courtside experience. That was two years ago. Now imagine how he might have floated into the Lakers’ home arena the night of March 6. Only hours earlier, it had been reported that ESPN had agreed to a new contract with Smith worth more than $100 million. To celebrate, Smith’s agent, Ari Emanuel, had invited him to the game. Larry David would join them courtside, near the end of the Lakers bench. This should have been the perfect atmosphere for Smith to revel in his ascent to the pinnacle of sports media. Instead, his night took a bad turn.
Early in the game, Smith made eye contact with Bronny James, a rookie reserve guard on the Lakers and, crucially, the son of the Lakers’ star LeBron James. Bronny has had a rough season. He has been dogged by accusations that he would not be playing in the NBA were it not for his last name. On the road, opposing crowds engage in mocking chants, begging the Lakers coach to put him in. In January, he had his worst game of the season; in 15 minutes, he missed all of his shots and turned the ball over three times. The next morning, on First Take, ESPN’s flagship morning show, Smith made Bronny the subject of one of his trademark rants. For more than four uninterrupted minutes, he pleaded with LeBron—as a father—to stop exposing his son. “Stop this,” he said. “Stop this.”
LeBron does not seem to have taken kindly to Smith’s unsolicited counsel. At the March 6 game, near the end of the third quarter, he approached Smith in his courtside seat. Looming over Smith in a manner that does not usually accompany friendly chatter, he barked something. His exact words can’t be heard in a fan video of the encounter, but his menacing tone is legible. According to Smith’s account of the exchange, LeBron said, “Stop fucking with my son. That’s my son.”
Smith did not respond to a request for comment. Neither did LeBron, so the details of what he said that night are unconfirmed. But even going by the body language and by related comments that LeBron later made on a hot mic, it does appear that his goal was to intimidate. By confronting Smith, LeBron sent a message not just to Smith but also to members of the wider NBA press corps, few of whom have Smith’s stature and influence: Criticize my son, and there will be consequences. Like many rich and powerful people, LeBron seems to want the fruits of global media stardom for himself and his kids, but not the corresponding scrutiny.
LeBron has himself to blame for the sad media spectacle of Bronny’s first season. In the months leading up to last year’s NBA draft, Bronny told reporters that it was his life’s great dream to be drafted by a pro team, any team. In off-the-record interviews, league executives made clear that because Bronny does not have his dad’s skill or size, he wasn’t all that draftable. In his short career at the University of Southern California, which was interrupted by a scary incident of cardiac arrest, he averaged only five points a game.
By all appearances, it was LeBron who made sure Bronny got drafted, first by puffing him up, saying publicly that his son was already better than many active NBA players. LeBron also made it known that he himself would play for whichever team drafted Bronny. (No prospect has ever been packaged so attractively, with the NBA’s first or second all-time best player as a throw-in.) On draft day, the Lakers selected Bronny in the second round. That night, Bronny shed tears; his dream had come true. At a press conference a few days later, the team’s coach, JJ Redick, tried to quiet any doubts. Bronny had earned the pick, he said, with hard work.
At the time, some of LeBron’s critics saw this as contemptible. I wasn’t one of them. Why appoint yourself to the meritocracy police just to make LeBron and his son your first arrests? LeBron’s own father was never in his life. He was still sleeping on friends’ couches late into his childhood. Parenthood means something deep to him; he has described playing with his son as the biggest accomplishment of a career that includes four NBA titles and four MVP awards. There was something beautiful about him using the leverage that he’s amassed in the basketball world to make that happen, by dragging his first-born boy into the league. Was it nepotism? Sure, but human life is shot through with all kinds of advantages of birth, and Bronny’s didn’t seem the most important or unfair.
LeBron deserves some empathy, even in the way he dealt with Smith. Nearly all parents experience something akin to his naive desire, a wish to give their kid the future of their dreams while shielding them from pain and disappointment. LeBron may have felt some anguish as he watched his son this season. Few things are as excruciating as watching your kids suffer. No amount of money or fame can insure against it. LeBron may well be haunted by his role in bringing about that suffering.
But even one of the greatest athletes of our age doesn’t—and certainly shouldn’t—have the power to protect his adult son from criticism. Indeed, what power LeBron has derives from the intense public curiosity about him as a basketball player and as a person. He was able to conjure a plus-one for a family member on an NBA roster precisely because he has been the league’s main character for a generation now. The media were always going to be interested in Bronny’s performance on the court, even if he’d made it there according to his own ability—and all the more so because he seemingly did not.
[Read: The secret code of pickup basketball]
LeBron has had more exposure to the NBA’s media ecosystem than any other active player. He knows that it rewards viral rants that lionize or denigrate a player based on their most recent game. And he should have known that Bronny would not be exempt from that dynamic, no matter how fiercely his father was protecting him.
Now, by appearing to threaten Smith, LeBron has not only acted like a petty strongman; he has drawn new attention to his son’s disappointing season, enlarging the very story that he sought to suppress. It’s a rare misstep for someone so media-savvy, who has amassed an enormous personal fortune while staying almost entirely scandal-free across a long career that began when he was still a teenager. The mistake is, perhaps, understandable. The emotions of parenthood are gigantic. They can knock anyone off their game, even the great LeBron James.
Illustration Sources: Tim Heitman / Getty; Gina Ferazzi / Los Angeles Times via Getty; Nathaniel S. Butler / Getty