Github Trending - Weekly
Github weekly trending
Building a modern alternative to Salesforce, powered by the community.
The #1 Open-Source CRM
๐ Website ยท ๐ Documentation ยท Roadmap ยท
Discord ยท
Figma
Installation
See:
๐ Self-hosting
๐ฅ๏ธ Local Setup
Does the world need another CRM?
We built Twenty for three reasons:
CRMs are too expensive, and users are trapped. Companies use locked-in customer data to hike prices. It shouldn't be that way.
A fresh start is required to build a better experience. We can learn from past mistakes and craft a cohesive experience inspired by new UX patterns from tools like Notion, Airtable or Linear.
We believe in Open-source and community. Hundreds of developers are already building Twenty together. Once we have plugin capabilities, a whole ecosystem will grow around it.
What You Can Do With Twenty
Please feel free to flag any specific needs you have by creating an issue.
Below are a few features we have implemented to date:
- Personalize layouts with filters, sort, group by, kanban and table views
- Customize your objects and fields
- Create and manage permissions with custom roles
- Automate workflow with triggers and actions
- Emails, calendar events, files, and more
Personalize layouts with filters, sort, group by, kanban and table views
Customize your objects and fields
Create and manage permissions with custom roles
Automate workflow with triggers and actions
Emails, calendar events, files, and more
Stack
- TypeScript
- Nx
- NestJS, with BullMQ, PostgreSQL, Redis
- React, with Recoil, Emotion and Lingui
Thanks
Thanks to these amazing services that we use and recommend for UI testing (Chromatic), code review (Greptile), catching bugs (Sentry) and translating (Crowdin).
Join the Community
- Star the repo
- Subscribe to releases (watch -> custom -> releases)
- Follow us on Twitter or LinkedIn
- Join our Discord
- Improve translations on Crowdin
- Contributions are, of course, most welcome!
Turso Database is a project to build the next evolution of SQLite.
Turso Database
Turso Database is an in-process SQL database, compatible with SQLite.
Features and Roadmap
Turso Database is a work-in-progress, in-process OLTP database engine library written in Rust that has:
- SQLite compatibility [doc] for SQL dialect, file formats, and the C API
- Language bindings for JavaScript/WebAssembly, Rust, Go, Python, and Java
- Asynchronous I/O support on Linux with
io_uring
- OS support for Linux, macOS, and Windows
In the future, we will be also working on:
BEGIN CONCURRENT
for improved write throughput.- Indexing for vector search.
- Improved schema management including better
ALTER
support and strict column types by default.
Getting Started
Please see the Turso Database Manual for more information.
๐ป Command Line
You can install the latest `turso` release with:
curl --proto '=https' --tlsv1.2 -LsSf \
https://github.com/tursodatabase/turso/releases/latest/download/turso_cli-installer.sh | sh
Then launch the shell to execute SQL statements:
Turso
Enter ".help" for usage hints.
Connected to a transient in-memory database.
Use ".open FILENAME" to reopen on a persistent database
turso> CREATE TABLE users (id INT PRIMARY KEY, username TEXT);
turso> INSERT INTO users VALUES (1, 'alice');
turso> INSERT INTO users VALUES (2, 'bob');
turso> SELECT * FROM users;
1|alice
2|bob
You can also build and run the latest development version with:
cargo run
๐ฆ Rust
cargo add turso
Example usage:
let db = Builder::new_local("sqlite.db").build().await?;
let conn = db.connect()?;
let res = conn.query("SELECT * FROM users", ()).await?;
โจ JavaScript
npm i @tursodatabase/turso
Example usage:
import { Database } from '@tursodatabase/turso';
const db = new Database('sqlite.db');
const stmt = db.prepare('SELECT * FROM users');
const users = stmt.all();
console.log(users);
๐ Python
pip install pyturso
Example usage:
import turso
con = turso.connect("sqlite.db")
cur = con.cursor()
res = cur.execute("SELECT * FROM users")
print(res.fetchone())
๐น Go
- Clone the repository
- Build the library and set your LD_LIBRARY_PATH to include turso's target directory
cargo build --package limbo-go
export LD_LIBRARY_PATH=/path/to/limbo/target/debug:$LD_LIBRARY_PATH
- Use the driver
go get github.com/tursodatabase/turso
go install github.com/tursodatabase/turso
Example usage:
import (
"database/sql"
_ "github.com/tursodatabase/turso"
)
conn, _ = sql.Open("sqlite3", "sqlite.db")
defer conn.Close()
stmt, _ := conn.Prepare("select * from users")
defer stmt.Close()
rows, _ = stmt.Query()
for rows.Next() {
var id int
var username string
_ := rows.Scan(&id, &username)
fmt.Printf("User: ID: %d, Username: %s\n", id, username)
}
โ๏ธ Java
We integrated Turso Database into JDBC. For detailed instructions on how to use Turso Database with java, please refer to the README.md under bindings/java.
Contributing
We'd love to have you contribute to Turso Database! Please check out the contribution guide to get started.
FAQ
Is Turso Database ready for production use?
Turso Database is currently under heavy development and is not ready for production use.
How is Turso Database different from Turso's libSQL?
Turso Database is a project to build the next evolution of SQLite in Rust, with a strong open contribution focus and features like native async support, vector search, and more. The libSQL project is also an attempt to evolve SQLite in a similar direction, but through a fork rather than a rewrite.
Rewriting SQLite in Rust started as an unassuming experiment, and due to its incredible success, replaces libSQL as our intended direction. At this point, libSQL is production ready, Turso Database is not - although it is evolving rapidly. More details here.
Publications
- Pekka Enberg, Sasu Tarkoma, Jon Crowcroft Ashwin Rao (2024). Serverless Runtime / Database Co-Design With Asynchronous I/O. In EdgeSys โ24. [PDF]
- Pekka Enberg, Sasu Tarkoma, and Ashwin Rao (2023). Towards Database and Serverless Runtime Co-Design. In CoNEXT-SW โ23. [PDF] [Slides]
License
This project is licensed under the MIT license.
Contribution
Unless you explicitly state otherwise, any contribution intentionally submitted for inclusion in Turso Database by you, shall be licensed as MIT, without any additional terms or conditions.
Partners
Thanks to all the partners of Turso!
Contributors
Thanks to all the contributors to Turso Database!
An open source graphics editor for 2025: comprehensive 2D content creation tool suite for graphic design, digital art, and interactive real-time motion graphics โ featuring node-based procedural editing
Your procedural toolbox for 2D content creation
Graphite is a free, open source vector and raster graphics engine, available now in alpha. Get creative with a fully nondestructive editing workflow that combines layer-based compositing with node-based generative design.
Having begun life as a vector editor, Graphite continues evolving into a generalized, all-in-one graphics toolbox that's built more like a game engine than a conventional creative app. The editor's tools wrap its node graph core, providing user-friendly workflows for vector, raster, and beyond. Photo editing, motion graphics, digital painting, desktop publishing, and VFX compositing are additional competencies on the planned roadmap making Graphite into a highly versatile content creation tool.
Learn more from the website, subscribe to the newsletter, consider volunteering or donating, and remember to give this repository a โญ!
https://github.com/user-attachments/assets/f4604aea-e8f1-45ce-9218-46ddc666f11d
Support our mission โค๏ธ
Graphite is 100% community built and funded. Please become a part of keeping the project alive and thriving with a donation if you share a belief in our mission:
Graphite strives to unshackle the creativity of every budding artist and seasoned professional by building the best comprehensive art and design tool that's accessible to all.
Mission success will come when Graphite is an industry standard. A cohesive product vision and focus on innovation over imitation is the strategy that will make that possible.
Screenshots
Contributing/building the code
Are you a graphics programmer or Rust developer? Graphite aims to be one of the most approachable projects for putting your engineering skills to use in the world of open source. See instructions here for setting up the project and getting started.
By submitting code for inclusion in the project, you are agreeing to license your changes under the Apache 2.0 license, and that you have the authority to do so. Some directories may have other licenses, like dual-licensed MIT/Apache 2.0, and code submissions to those directories mean you agree to the applicable license(s).
Scira (Formerly MiniPerplx) is a minimalistic AI-powered search engine that helps you find information on the internet and cites it too. Powered by Vercel AI SDK! Search with models like xAI's Grok 3.
Scira
A minimalistic AI-powered search engine that helps you find information on the internet.
Powered By
- Vercel AI SDK - For AI model integration and streaming
- Tavily AI - For search grounding and web search capabilities
Special Thanks
Features
Core Search & Information
- AI-powered search: Get answers to your questions using multiple AI models including xAI's Grok, Anthropic's Claude, Google's Gemini, and OpenAI's GPT models
- Web search: Search the web using Tavily's API with support for multiple queries, search depths, and topics
- URL content retrieval: Extract and analyze content from any URL using Exa AI with live crawling capabilities
- Reddit search: Search Reddit content with time range filtering using Tavily API
- X (Twitter) search: Search X posts with date ranges and specific handle filtering using xAI Live Search
- Extreme search: Advanced multi-step search capability for complex queries
Academic & Research
- Academic search: Search for academic papers and research using Exa AI with abstracts and summaries
- YouTube search: Find YouTube videos with detailed information, captions, and timestamps powered by Exa AI
Entertainment & Media
- Movie & TV show search: Get detailed information about movies and TV shows using TMDB API
- Trending movies: Discover trending movies with cast, ratings, and detailed information
- Trending TV shows: Find popular TV shows with comprehensive metadata
Financial & Data Analysis
- Stock charts: Generate interactive stock charts with news integration using yfinance and Tavily
- Currency converter: Convert between currencies with real-time exchange rates using yfinance
- Code interpreter: Write and execute Python code with chart generation capabilities using Daytona sandbox
Location & Travel
- Weather information: Get current weather and forecasts for any location using OpenWeather API
- Maps & geocoding: Find places and get coordinates using Google Maps API
- Nearby places search: Discover nearby restaurants, attractions, and services with Google Places API
- Flight tracking: Track real-time flight information using Aviation Stack API
Productivity & Utilities
- Text translation: Translate text between languages using AI models
- Date & time: Get current date and time in user's timezone with multiple format options
- Memory management: Add and search personal memories using Mem0 AI
- MCP server search: Search for Model Context Protocol servers using Smithery Registry
Search Groups
- Web: Search across the entire internet powered by Tavily
- Memory: Your personal memory companion (requires authentication)
- Analysis: Code execution, stock charts, and currency conversion
- Chat: Direct conversation with AI models
- X: Search X (Twitter) posts
- Reddit: Search Reddit posts
- Academic: Search academic papers powered by Exa
- YouTube: Search YouTube videos powered by Exa
- Extreme: Deep research with multiple sources and analysis
LLM Models Supported
- xAI: Grok 3, Grok 3 Mini, Grok 2 Vision
- Google: Gemini 2.5 Flash (Preview), Gemini 2.5 Pro (Preview)
- Anthropic: Claude 4 Sonnet, Claude 4 Opus (with thinking capabilities)
- OpenAI: GPT-4o, o4-mini, o3 (with reasoning capabilities)
- Groq: Qwen QwQ 32B, Qwen 3 32B, Meta's Llama 4 Maverick
Built with
- Next.js - React framework
- Tailwind CSS - Styling
- Vercel AI SDK - AI model integration
- Shadcn/UI - UI components
- Exa.AI - Web search and content retrieval
- Tavily - Search grounding
- OpenWeather - Weather data
- Daytona - Code execution sandbox
- Google Maps - Location services
- Aviation Stack - Flight tracking
- TMDB - Movie and TV data
- Mem0 - Memory management
- Better Auth - Authentication
- Drizzle ORM - Database management
Deploy your own
Set Scira as your default search engine
-
Open the Chrome browser settings:
- Click on the three vertical dots in the upper right corner of the browser.
- Select "Settings" from the dropdown menu.
-
Go to the search engine settings:
- In the left sidebar, click on "Search engine."
- Then select "Manage search engines and site search."
-
Add a new search engine:
- Click on "Add" next to "Site search."
-
Set the search engine name:
- Enter
Scira
in the "Search engine" field.
- Enter
-
Set the search engine URL:
- Enter
https://scira.ai?q=%s
in the "URL with %s in place of query" field.
- Enter
-
Set the search engine shortcut:
- Enter
sh
in the "Shortcut" field.
- Enter
-
Set Default:
- Click on the three dots next to the search engine you just added.
- Select "Make default" from the dropdown menu.
After completing these steps, you should be able to use Scira as your default search engine in Chrome.
Local development
Run via Docker
The application can be run using Docker in two ways:
Using Docker Compose (Recommended)
- Make sure you have Docker and Docker Compose installed on your system
- Create a
.env
file based on.env.example
with your API keys - Run the following command in the project root:
docker compose up
- The application will be available at
http://localhost:3000
Using Docker Directly
- Create a
.env
file based on.env.example
with your API keys - Build the Docker image:
docker build -t scira.app .
- Run the container:
docker run --env-file .env -p 3000:3000 scira.app
The application uses a multi-stage build process to minimize the final image size and implements security best practices. The production image runs on Node.js LTS with Alpine Linux for a minimal footprint.
Run with Node.js
To run the application locally without Docker:
- Sign up for accounts with the required AI providers:
- OpenAI (required)
- Anthropic (required)
- Tavily (required for web search feature)
- Copy
.env.example
to.env.local
and fill in your API keys - Install dependencies:
pnpm install
- Start the development server:
pnpm dev
- Open
http://localhost:3000
in your browser
License
This project is licensed under the Apache 2.0 License - see the LICENSE file for details.
Invoicing, Time tracking, File reconciliation, Storage, Financial Overview & your own Assistant made for Freelancers
Midday
Run your business smarter
Discord ยท Website ยท Issues
About Midday
Midday is an all-in-one tool designed to help freelancers, contractors, consultants, and solo entrepreneurs manage their business operations more efficiently. It integrates various functions typically scattered across multiple platforms into a single, cohesive system.
Features
Time Tracking: Allows for live time tracking of projects to boost productivity and collaboration, providing insightful project overviews.
Invoicing: An upcoming feature that will enable users to create web-based invoices, collaborate in real-time, and synchronize projects seamlessly.
Magic Inbox: Automatically matches incoming invoices or receipts to the correct transactions, simplifying financial tracking and organization.
Vault: Secure storage for important files like contracts and agreements, keeping everything in one place for easy accessโ.
Seamless Export: Facilitates easy export of financial data, packaged neatly in CSV files for accountants.
Assistant: Provides tailored insights into financial situations, helping users understand spending patterns, cut costs, and find documents.
Recognition
Get started
We are working on the documentation to get started with Midday for local development: https://docs.midday.ai
App Architecture
- Monorepo
- Bun
- React
- TypeScript
- Nextjs
- Supabase
- Shadcn
- Tauri
- Expo
- TailwindCSS
Hosting
- Supabase (database, storage, realtime, auth)
- Vercel (Website, Dashboard)
- Fly.io (API/tRPC)
Services
- Trigger.dev (background jobs)
- Resend (Transactional & Marketing)
- Novu (notifications)
- Github Actions (CI/CD)
- GoCardLess (Bank connection EU)
- Plaid (Bank connection in Canada and US)
- Teller (Bank connection in the US)
- OpenPanel (Events and Analytics)
- Polar (Payment processing)
- Typesense (Search)
- Mistral
- OpenAI
Repo Activity
License
This project is licensed under the AGPL-3.0 for non-commercial use.
Commercial Use
For commercial use or deployments requiring a setup fee, please contact us for a commercial license at [email protected].
By using this software, you agree to the terms of the license.
A collection of guides and examples for the Gemma open models from Google.
Welcome to the Gemma Cookbook
This is a collection of guides and examples for Google Gemma.
Get started with the Gemma models
Gemma is a family of lightweight, generative artificial intelligence (AI) open models, built from the same research and technology used to create the Gemini models. The Gemma model family includes:
- Gemma
The core models of the Gemma family.- Gemma
For a variety of text generation tasks and can be further tuned for specific use cases - Gemma 2
Higher-performing and more efficient, available in 2B, 9B, 27B parameter sizes - Gemma 3
Longer context window and handling text and image input, available in 1B, 4B, 12B and 27B parameter sizes - Gemma 3n
Designed for efficient execution on low-resource devices. Handling text, image, video, and audio input, available in E2B and E4B parameter sizes
- Gemma
- Gemma variants
- CodeGemma
Fine-tuned for a variety of coding tasks - PaliGemma
Vision Language Model
For a deeper analysis of images and provide useful insights - PaliGemma 2
VLM which incorporates the capabilities of the Gemma 2 models - RecurrentGemma
Based on Griffin architecture
For a variety of text generation tasks - ShieldGemma
Fine-tuned for evaluating the safety of text prompt input and text output responses against a set of defined safety policies - ShieldGemma 2
Fine-tuned on Gemma 3's 4B IT checkpoint for image safety classification - DataGemma
Fine-tuned for using Data Commons to address AI hallucinations
- CodeGemma
You can find the Gemma models on the Hugging Face Hub, Kaggle, Google Cloud Vertex AI Model Garden, and ai.nvidia.com.
Table of Notebooks
- Gemma
- CodeGemma
- PaliGemma
- Workshops and technical talks
- Showcase complex end-to-end use cases
- Gemma on Google Cloud : GCP open models has additional notebooks for using Gemma
Get help
Ask a Gemma cookbook-related question on the developer forum, or open an issue on GitHub.
Wish list
If you want to see additional cookbooks implemented for specific features/integrations, please open a new issue with โFeature Requestโ template.
If you want to make contributions to the Gemma Cookbook project, you are welcome to pick any idea in the โWish Listโ and implement it.
Contributing
Contributions are always welcome. Please read contributing before implementation.
Thank you for developing with Gemma! Weโre excited to see what you create.
Translation of this repository
21 Lessons, Get Started Building with Generative AI ๐ https://microsoft.github.io/generative-ai-for-beginners/
21 Lessons teaching everything you need to know to start building Generative AI applications
๐ Multi-Language Support
Supported via GitHub Action (Automated & Always Up-to-Date)
French | Spanish | German | Russian | Arabic | Persian (Farsi) | Urdu | Chinese (Simplified) | Chinese (Traditional, Macau) | Chinese (Traditional, Hong Kong) | Chinese (Traditional, Taiwan) | Japanese | Korean | Hindi | Bengali | Marathi | Nepali | Punjabi (Gurmukhi) | Portuguese (Portugal) | Portuguese (Brazil) | Italian | Polish | Turkish | Greek | Thai | Swedish | Danish | Norwegian | Finnish | Dutch | Hebrew | Vietnamese | Indonesian | Malay | Tagalog (Filipino) | Swahili | Hungarian | Czech | Slovak | Romanian | Bulgarian | Serbian (Cyrillic) | Croatian | Slovenian
Generative AI for Beginners (Version 3) - A Course
Learn the fundamentals of building Generative AI applications with our 21-lesson comprehensive course by Microsoft Cloud Advocates.
๐ฑ Getting Started
This course has 21 lessons. Each lesson covers its own topic so start wherever you like!
Lessons are labeled either "Learn" lessons explaining a Generative AI concept or "Build" lessons that explain a concept and code examples in both Python and TypeScript when possible.
For .NET Developers checkout Generative AI for Beginners (.NET Edition)!
Each lesson also includes a "Keep Learning" section with additional learning tools.
What You Need
To run the code of this course, you can use either:
-
Azure OpenAI Service - Lessons: "aoai-assignment"
-
GitHub Marketplace Model Catalog - Lessons: "githubmodels"
-
OpenAI API - Lessons: "oai-assignment"
-
Basic knowledge of Python or TypeScript is helpful - *For absolute beginners check out these Python and TypeScript courses
-
A GitHub account to fork this entire repo to your own GitHub account
We have created a Course Setup lesson to help you with setting up your development environment.
Don't forget to star (๐) this repo to find it easier later.
๐ง Ready to Deploy?
If you are looking for more advanced code samples, check out our collection of Generative AI Code Samples in both Python and TypeScript.
๐ฃ๏ธ Meet Other Learners, Get Support
Join our official Azure AI Foundry Discord server to meet and network with other learners taking this course and get support.
Ask questions or share product feedback in our Azure AI Foundry Developer Forum on Github.
๐ Building a Startup?
Sign up for Microsoft for Startups Founders Hub to receive free OpenAI credits and up to $150k towards Azure credits to access OpenAI models through Azure OpenAI Services.
๐ Want to help?
Do you have suggestions or found spelling or code errors? Raise an issue or Create a pull request
๐ Each lesson includes:
- A short video introduction to the topic
- A written lesson located in the README
- Python and TypeScript code samples supporting Azure OpenAI and OpenAI API
- Links to extra resources to continue your learning
๐๏ธ Lessons
# | Lesson Link | Description | Video | Extra Learning |
---|---|---|---|---|
00 | Course Setup | Learn: How to Setup Your Development Environment | Video Coming Soon | Learn More |
01 | Introduction to Generative AI and LLMs | Learn: Understanding what Generative AI is and how Large Language Models (LLMs) work. | Video | Learn More |
02 | Exploring and comparing different LLMs | Learn: How to select the right model for your use case | Video | Learn More |
03 | Using Generative AI Responsibly | Learn: How to build Generative AI Applications responsibly | Video | Learn More |
04 | Understanding Prompt Engineering Fundamentals | Learn: Hands-on Prompt Engineering Best Practices | Video | Learn More |
05 | Creating Advanced Prompts | Learn: How to apply prompt engineering techniques that improve the outcome of your prompts. | Video | Learn More |
06 | Building Text Generation Applications | Build: A text generation app using Azure OpenAI / OpenAI API | Video | Learn More |
07 | Building Chat Applications | Build: Techniques for efficiently building and integrating chat applications. | Video | Learn More |
08 | Building Search Apps Vector Databases | Build: A search application that uses Embeddings to search for data. | Video | Learn More |
09 | Building Image Generation Applications | Build: An image generation application | Video | Learn More |
10 | Building Low Code AI Applications | Build: A Generative AI application using Low Code tools | Video | Learn More |
11 | Integrating External Applications with Function Calling | Build: What is function calling and its use cases for applications | Video | Learn More |
12 | Designing UX for AI Applications | Learn: How to apply UX design principles when developing Generative AI Applications | Video | Learn More |
13 | Securing Your Generative AI Applications | Learn: The threats and risks to AI systems and methods to secure these systems. | Video | Learn More |
14 | The Generative AI Application Lifecycle | Learn: The tools and metrics to manage the LLM Lifecycle and LLMOps | Video | Learn More |
15 | Retrieval Augmented Generation (RAG) and Vector Databases | Build: An application using a RAG Framework to retrieve embeddings from a Vector Databases | Video | Learn More |
16 | Open Source Models and Hugging Face | Build: An application using open source models available on Hugging Face | Video | Learn More |
17 | AI Agents | Build: An application using an AI Agent Framework | Video | Learn More |
18 | Fine-Tuning LLMs | Learn: The what, why and how of fine-tuning LLMs | Video | Learn More |
19 | Building with SLMs | Learn: The benefits of building with Small Language Models | Video Coming Soon | Learn More |
20 | Building with Mistral Models | Learn: The features and differences of the Mistral Family Models | Video Coming Soon | Learn More |
21 | Building with Meta Models | Learn: The features and differences of the Meta Family Models | Video Coming Soon | Learn More |
๐ Special thanks
Special thanks to John Aziz for creating all of the GitHub Actions and workflows
Bernhard Merkle for making key contributions to each lesson to improve the learner and code experience.
๐ Other Courses
Our team produces other courses! Check out:
- NEW Model Context Protocol for Beginners
- AI Agents for Beginners
- Generative AI for Beginners using .NET
- Generative AI for Beginners using JavaScript
- ML for Beginners
- Data Science for Beginners
- AI for Beginners
- Cybersecurity for Beginners
- Web Dev for Beginners
- IoT for Beginners
- XR Development for Beginners
- Mastering GitHub Copilot for AI Paired Programming
- Mastering GitHub Copilot for C#/.NET Developers
- Choose Your Own Copilot Adventure
An LLM-powered knowledge curation system that researches a topic and generates a full-length report with citations.
STORM: Synthesis of Topic Outlines through Retrieval and Multi-perspective Question Asking
| Research preview | STORM Paper| Co-STORM Paper | Website |
**Latest News** ๐ฅ-
[2025/01] We add litellm integration for language models and embedding models in
knowledge-storm
v1.1.0. -
[2024/09] Co-STORM codebase is now released and integrated into
knowledge-storm
python package v1.0.0. Runpip install knowledge-storm --upgrade
to check it out. -
[2024/09] We introduce collaborative STORM (Co-STORM) to support human-AI collaborative knowledge curation! Co-STORM Paper has been accepted to EMNLP 2024 main conference.
-
[2024/07] You can now install our package with
pip install knowledge-storm
! -
[2024/07] We add
VectorRM
to support grounding on user-provided documents, complementing existing support of search engines (YouRM
,BingSearch
). (check out #58) -
[2024/07] We release demo light for developers a minimal user interface built with streamlit framework in Python, handy for local development and demo hosting (checkout #54)
-
[2024/06] We will present STORM at NAACL 2024! Find us at Poster Session 2 on June 17 or check our presentation material.
-
[2024/05] We add Bing Search support in rm.py. Test STORM with
GPT-4o
- we now configure the article generation part in our demo usingGPT-4o
model. -
[2024/04] We release refactored version of STORM codebase! We define interface for STORM pipeline and reimplement STORM-wiki (check out
src/storm_wiki
) to demonstrate how to instantiate the pipeline. We provide API to support customization of different language models and retrieval/search integration.
Overview (Try STORM now!)
While the system cannot produce publication-ready articles that often require a significant number of edits, experienced Wikipedia editors have found it helpful in their pre-writing stage.
More than 70,000 people have tried our live research preview. Try it out to see how STORM can help your knowledge exploration journey and please provide feedback to help us improve the system ๐!
How STORM & Co-STORM works
STORM
STORM breaks down generating long articles with citations into two steps:
- Pre-writing stage: The system conducts Internet-based research to collect references and generates an outline.
- Writing stage: The system uses the outline and references to generate the full-length article with citations.
STORM identifies the core of automating the research process as automatically coming up with good questions to ask. Directly prompting the language model to ask questions does not work well. To improve the depth and breadth of the questions, STORM adopts two strategies:
- Perspective-Guided Question Asking: Given the input topic, STORM discovers different perspectives by surveying existing articles from similar topics and uses them to control the question-asking process.
- Simulated Conversation: STORM simulates a conversation between a Wikipedia writer and a topic expert grounded in Internet sources to enable the language model to update its understanding of the topic and ask follow-up questions.
CO-STORM
Co-STORM proposes a collaborative discourse protocol which implements a turn management policy to support smooth collaboration among
- Co-STORM LLM experts: This type of agent generates answers grounded on external knowledge sources and/or raises follow-up questions based on the discourse history.
- Moderator: This agent generates thought-provoking questions inspired by information discovered by the retriever but not directly used in previous turns. Question generation can also be grounded!
- Human user: The human user will take the initiative to either (1) observe the discourse to gain deeper understanding of the topic, or (2) actively engage in the conversation by injecting utterances to steer the discussion focus.
Co-STORM also maintains a dynamic updated mind map, which organize collected information into a hierarchical concept structure, aiming to build a shared conceptual space between the human user and the system. The mind map has been proven to help reduce the mental load when the discourse goes long and in-depth.
Both STORM and Co-STORM are implemented in a highly modular way using dspy.
Installation
To install the knowledge storm library, use pip install knowledge-storm
.
You could also install the source code which allows you to modify the behavior of STORM engine directly.
-
Clone the git repository.
git clone https://github.com/stanford-oval/storm.git cd storm
-
Install the required packages.
conda create -n storm python=3.11 conda activate storm pip install -r requirements.txt
API
Currently, our package support:
- Language model components: All language models supported by litellm as listed here
- Embedding model components: All embedding models supported by litellm as listed here
- retrieval module components:
YouRM
,BingSearch
,VectorRM
,SerperRM
,BraveRM
,SearXNG
,DuckDuckGoSearchRM
,TavilySearchRM
,GoogleSearch
, andAzureAISearch
as
๐ PRs for integrating more search engines/retrievers into knowledge_storm/rm.py are highly appreciated!
Both STORM and Co-STORM are working in the information curation layer, you need to set up the information retrieval module and language model module to create their Runner
classes respectively.
STORM
The STORM knowledge curation engine is defined as a simple Python STORMWikiRunner
class. Here is an example of using You.com search engine and OpenAI models.
import os
from knowledge_storm import STORMWikiRunnerArguments, STORMWikiRunner, STORMWikiLMConfigs
from knowledge_storm.lm import LitellmModel
from knowledge_storm.rm import YouRM
lm_configs = STORMWikiLMConfigs()
openai_kwargs = {
'api_key': os.getenv("OPENAI_API_KEY"),
'temperature': 1.0,
'top_p': 0.9,
}
# STORM is a LM system so different components can be powered by different models to reach a good balance between cost and quality.
# For a good practice, choose a cheaper/faster model for `conv_simulator_lm` which is used to split queries, synthesize answers in the conversation.
# Choose a more powerful model for `article_gen_lm` to generate verifiable text with citations.
gpt_35 = LitellmModel(model='gpt-3.5-turbo', max_tokens=500, **openai_kwargs)
gpt_4 = LitellmModel(model='gpt-4o', max_tokens=3000, **openai_kwargs)
lm_configs.set_conv_simulator_lm(gpt_35)
lm_configs.set_question_asker_lm(gpt_35)
lm_configs.set_outline_gen_lm(gpt_4)
lm_configs.set_article_gen_lm(gpt_4)
lm_configs.set_article_polish_lm(gpt_4)
# Check out the STORMWikiRunnerArguments class for more configurations.
engine_args = STORMWikiRunnerArguments(...)
rm = YouRM(ydc_api_key=os.getenv('YDC_API_KEY'), k=engine_args.search_top_k)
runner = STORMWikiRunner(engine_args, lm_configs, rm)
The STORMWikiRunner
instance can be evoked with the simple run
method:
topic = input('Topic: ')
runner.run(
topic=topic,
do_research=True,
do_generate_outline=True,
do_generate_article=True,
do_polish_article=True,
)
runner.post_run()
runner.summary()
do_research
: if True, simulate conversations with difference perspectives to collect information about the topic; otherwise, load the results.do_generate_outline
: if True, generate an outline for the topic; otherwise, load the results.do_generate_article
: if True, generate an article for the topic based on the outline and the collected information; otherwise, load the results.do_polish_article
: if True, polish the article by adding a summarization section and (optionally) removing duplicate content; otherwise, load the results.
Co-STORM
The Co-STORM knowledge curation engine is defined as a simple Python CoStormRunner
class. Here is an example of using Bing search engine and OpenAI models.
from knowledge_storm.collaborative_storm.engine import CollaborativeStormLMConfigs, RunnerArgument, CoStormRunner
from knowledge_storm.lm import LitellmModel
from knowledge_storm.logging_wrapper import LoggingWrapper
from knowledge_storm.rm import BingSearch
# Co-STORM adopts the same multi LM system paradigm as STORM
lm_config: CollaborativeStormLMConfigs = CollaborativeStormLMConfigs()
openai_kwargs = {
"api_key": os.getenv("OPENAI_API_KEY"),
"api_provider": "openai",
"temperature": 1.0,
"top_p": 0.9,
"api_base": None,
}
question_answering_lm = LitellmModel(model=gpt_4o_model_name, max_tokens=1000, **openai_kwargs)
discourse_manage_lm = LitellmModel(model=gpt_4o_model_name, max_tokens=500, **openai_kwargs)
utterance_polishing_lm = LitellmModel(model=gpt_4o_model_name, max_tokens=2000, **openai_kwargs)
warmstart_outline_gen_lm = LitellmModel(model=gpt_4o_model_name, max_tokens=500, **openai_kwargs)
question_asking_lm = LitellmModel(model=gpt_4o_model_name, max_tokens=300, **openai_kwargs)
knowledge_base_lm = LitellmModel(model=gpt_4o_model_name, max_tokens=1000, **openai_kwargs)
lm_config.set_question_answering_lm(question_answering_lm)
lm_config.set_discourse_manage_lm(discourse_manage_lm)
lm_config.set_utterance_polishing_lm(utterance_polishing_lm)
lm_config.set_warmstart_outline_gen_lm(warmstart_outline_gen_lm)
lm_config.set_question_asking_lm(question_asking_lm)
lm_config.set_knowledge_base_lm(knowledge_base_lm)
# Check out the Co-STORM's RunnerArguments class for more configurations.
topic = input('Topic: ')
runner_argument = RunnerArgument(topic=topic, ...)
logging_wrapper = LoggingWrapper(lm_config)
bing_rm = BingSearch(bing_search_api_key=os.environ.get("BING_SEARCH_API_KEY"),
k=runner_argument.retrieve_top_k)
costorm_runner = CoStormRunner(lm_config=lm_config,
runner_argument=runner_argument,
logging_wrapper=logging_wrapper,
rm=bing_rm)
The CoStormRunner
instance can be evoked with the warmstart()
and step(...)
methods.
# Warm start the system to build shared conceptual space between Co-STORM and users
costorm_runner.warm_start()
# Step through the collaborative discourse
# Run either of the code snippets below in any order, as many times as you'd like
# To observe the conversation:
conv_turn = costorm_runner.step()
# To inject your utterance to actively steer the conversation:
costorm_runner.step(user_utterance="YOUR UTTERANCE HERE")
# Generate report based on the collaborative discourse
costorm_runner.knowledge_base.reorganize()
article = costorm_runner.generate_report()
print(article)
Quick Start with Example Scripts
We provide scripts in our examples folder as a quick start to run STORM and Co-STORM with different configurations.
We suggest using secrets.toml
to set up the API keys. Create a file secrets.toml
under the root directory and add the following content:
# ============ language model configurations ============
# Set up OpenAI API key.
OPENAI_API_KEY="your_openai_api_key"
# If you are using the API service provided by OpenAI, include the following line:
OPENAI_API_TYPE="openai"
# If you are using the API service provided by Microsoft Azure, include the following lines:
OPENAI_API_TYPE="azure"
AZURE_API_BASE="your_azure_api_base_url"
AZURE_API_VERSION="your_azure_api_version"
# ============ retriever configurations ============
BING_SEARCH_API_KEY="your_bing_search_api_key" # if using bing search
# ============ encoder configurations ============
ENCODER_API_TYPE="openai" # if using openai encoder
STORM examples
To run STORM with gpt
family models with default configurations:
Run the following command.
python examples/storm_examples/run_storm_wiki_gpt.py \
--output-dir $OUTPUT_DIR \
--retriever bing \
--do-research \
--do-generate-outline \
--do-generate-article \
--do-polish-article
To run STORM using your favorite language models or grounding on your own corpus: Check out examples/storm_examples/README.md.
Co-STORM examples
To run Co-STORM with gpt
family models with default configurations,
- Add
BING_SEARCH_API_KEY="xxx"
andENCODER_API_TYPE="xxx"
tosecrets.toml
- Run the following command
python examples/costorm_examples/run_costorm_gpt.py \
--output-dir $OUTPUT_DIR \
--retriever bing
Customization of the Pipeline
STORM
If you have installed the source code, you can customize STORM based on your own use case. STORM engine consists of 4 modules:
- Knowledge Curation Module: Collects a broad coverage of information about the given topic.
- Outline Generation Module: Organizes the collected information by generating a hierarchical outline for the curated knowledge.
- Article Generation Module: Populates the generated outline with the collected information.
- Article Polishing Module: Refines and enhances the written article for better presentation.
The interface for each module is defined in knowledge_storm/interface.py
, while their implementations are instantiated in knowledge_storm/storm_wiki/modules/*
. These modules can be customized according to your specific requirements (e.g., generating sections in bullet point format instead of full paragraphs).
Co-STORM
If you have installed the source code, you can customize Co-STORM based on your own use case
- Co-STORM introduces multiple LLM agent types (i.e. Co-STORM experts and Moderator). LLM agent interface is defined in
knowledge_storm/interface.py
, while its implementation is instantiated inknowledge_storm/collaborative_storm/modules/co_storm_agents.py
. Different LLM agent policies can be customized. - Co-STORM introduces a collaborative discourse protocol, with its core function centered on turn policy management. We provide an example implementation of turn policy management through
DiscourseManager
inknowledge_storm/collaborative_storm/engine.py
. It can be customized and further improved.
Datasets
To facilitate the study of automatic knowledge curation and complex information seeking, our project releases the following datasets:
FreshWiki
The FreshWiki Dataset is a collection of 100 high-quality Wikipedia articles focusing on the most-edited pages from February 2022 to September 2023. See Section 2.1 in STORM paper for more details.
You can download the dataset from huggingface directly. To ease the data contamination issue, we archive the source code for the data construction pipeline that can be repeated at future dates.
WildSeek
To study usersโ interests in complex information seeking tasks in the wild, we utilized data collected from the web research preview to create the WildSeek dataset. We downsampled the data to ensure the diversity of the topics and the quality of the data. Each data point is a pair comprising a topic and the userโs goal for conducting deep search on the topic. For more details, please refer to Section 2.2 and Appendix A of Co-STORM paper.
The WildSeek dataset is available here.
Replicate STORM & Co-STORM paper result
For STORM paper experiments, please switch to the branch NAACL-2024-code-backup
here.
For Co-STORM paper experiments, please switch to the branch EMNLP-2024-code-backup
(placeholder for now, will be updated soon).
Roadmap & Contributions
Our team is actively working on:
- Human-in-the-Loop Functionalities: Supporting user participation in the knowledge curation process.
- Information Abstraction: Developing abstractions for curated information to support presentation formats beyond the Wikipedia-style report.
If you have any questions or suggestions, please feel free to open an issue or pull request. We welcome contributions to improve the system and the codebase!
Contact person: Yijia Shao and Yucheng Jiang
Acknowledgement
We would like to thank Wikipedia for its excellent open-source content. The FreshWiki dataset is sourced from Wikipedia, licensed under the Creative Commons Attribution-ShareAlike (CC BY-SA) license.
We are very grateful to Michelle Lam for designing the logo for this project and Dekun Ma for leading the UI development.
Thanks to Vercel for their support of open-source software
Citation
Please cite our paper if you use this code or part of it in your work:
@inproceedings{jiang-etal-2024-unknown,
title = "Into the Unknown Unknowns: Engaged Human Learning through Participation in Language Model Agent Conversations",
author = "Jiang, Yucheng and
Shao, Yijia and
Ma, Dekun and
Semnani, Sina and
Lam, Monica",
editor = "Al-Onaizan, Yaser and
Bansal, Mohit and
Chen, Yun-Nung",
booktitle = "Proceedings of the 2024 Conference on Empirical Methods in Natural Language Processing",
month = nov,
year = "2024",
address = "Miami, Florida, USA",
publisher = "Association for Computational Linguistics",
url = "https://aclanthology.org/2024.emnlp-main.554/",
doi = "10.18653/v1/2024.emnlp-main.554",
pages = "9917--9955",
}
@inproceedings{shao-etal-2024-assisting,
title = "Assisting in Writing {W}ikipedia-like Articles From Scratch with Large Language Models",
author = "Shao, Yijia and
Jiang, Yucheng and
Kanell, Theodore and
Xu, Peter and
Khattab, Omar and
Lam, Monica",
editor = "Duh, Kevin and
Gomez, Helena and
Bethard, Steven",
booktitle = "Proceedings of the 2024 Conference of the North American Chapter of the Association for Computational Linguistics: Human Language Technologies (Volume 1: Long Papers)",
month = jun,
year = "2024",
address = "Mexico City, Mexico",
publisher = "Association for Computational Linguistics",
url = "https://aclanthology.org/2024.naacl-long.347/",
doi = "10.18653/v1/2024.naacl-long.347",
pages = "6252--6278",
}
API for Whatsapp Web Multi Device Version, Support UI, Webhook & MCP
WhatsApp API Multi Device Version
If you're using this tools to generate income, consider supporting its development by becoming a Patreon member!
Your support helps ensure the library stays maintained and receives regular updates!
Support for ARM
& AMD
Architecture along with MCP
Support
Download:
Support n8n package (n8n.io)
- n8n package
- Go to Settings -> Community Nodes -> Input
@aldinokemal2104/n8n-nodes-gowa
-> Install
Breaking Changes
v6
- For REST mode, you need to run
<binary> rest
instead of<binary>
- for example:
./whatsapp rest
instead of./whatsapp
- for example:
- For MCP mode, you need to run
<binary> mcp
- for example:
./whatsapp mcp
- for example:
- For REST mode, you need to run
Feature
-
Send WhatsApp message via http API, docs/openapi.yml for more details
-
MCP (Model Context Protocol) Server Support - Integrate with AI agents and tools using standardized protocol
-
Mention someone
@phoneNumber
- example:
Hello @628974812XXXX, @628974812XXXX
-
Post Whatsapp Status
-
Compress image before send
-
Compress video before send
-
Change OS name become your app (it's the device name when connect via mobile)
--os=Chrome
or--os=MyApplication
-
Basic Auth (able to add multi credentials)
--basic-auth=kemal:secret,toni:password,userName:secretPassword
, or you can simplify-b=kemal:secret,toni:password,userName:secretPassword
-
Customizable port and debug mode
--port 8000
--debug true
-
Auto reply message
--autoreply="Don't reply this message"
-
Webhook for received message
--webhook="http://yourwebhook.site/handler"
, or you can simplify-w="http://yourwebhook.site/handler"
-
Webhook Secret Our webhook will be sent to you with an HMAC header and a sha256 default key
secret
.You may modify this by using the option below:
--webhook-secret="secret"
Configuration
You can configure the application using either command-line flags (shown above) or environment variables. Configuration can be set in three ways (in order of priority):
- Command-line flags (highest priority)
- Environment variables
.env
file (lowest priority)
Environment Variables
You can configure the application using environment variables. Configuration can be set in three ways (in order of priority):
- Command-line flags (highest priority)
- Environment variables
.env
file (lowest priority)
To use environment variables:
- Copy
.env.example
to.env
in your project root (cp src/.env.example src/.env
) - Modify the values in
.env
according to your needs - Or set the same variables as system environment variables
Available Environment Variables
Variable | Description | Default | Example |
---|---|---|---|
APP_PORT |
Application port | 3000 |
APP_PORT=8080 |
APP_DEBUG |
Enable debug logging | false |
APP_DEBUG=true |
APP_OS |
OS name (device name in WhatsApp) | Chrome |
APP_OS=MyApp |
APP_BASIC_AUTH |
Basic authentication credentials | - | APP_BASIC_AUTH=user1:pass1,user2:pass2 |
APP_CHAT_FLUSH_INTERVAL |
Chat flush interval in days | 7 |
APP_CHAT_FLUSH_INTERVAL=30 |
DB_URI |
Database connection URI | file:storages/whatsapp.db?_foreign_keys=on |
DB_URI=postgres://user:pass@host/db |
WHATSAPP_AUTO_REPLY |
Auto-reply message | - | WHATSAPP_AUTO_REPLY="Auto reply message" |
WHATSAPP_WEBHOOK |
Webhook URL(s) for events (comma-separated) | - | WHATSAPP_WEBHOOK=https://webhook.site/xxx |
WHATSAPP_WEBHOOK_SECRET |
Webhook secret for validation | secret |
WHATSAPP_WEBHOOK_SECRET=super-secret-key |
WHATSAPP_ACCOUNT_VALIDATION |
Enable account validation | true |
WHATSAPP_ACCOUNT_VALIDATION=false |
WHATSAPP_CHAT_STORAGE |
Enable chat storage | true |
WHATSAPP_CHAT_STORAGE=false |
Note: Command-line flags will override any values set in environment variables or .env
file.
- For more command
./whatsapp --help
Requirements
System Requirements
- Go 1.24.0 or higher (for building from source)
- FFmpeg (for media processing)
Platform Support
- Linux (x86_64, ARM64)
- macOS (Intel, Apple Silicon)
- Windows (x86_64) - WSL recommended
Dependencies (without docker)
- Mac OS:
brew install ffmpeg
export CGO_CFLAGS_ALLOW="-Xpreprocessor"
- Linux:
sudo apt update
sudo apt install ffmpeg
- Windows (not recomended, prefer using WSL):
- install ffmpeg, download here
- add to ffmpeg to environment variable
How to use
Basic
- Clone this repo:
git clone https://github.com/aldinokemal/go-whatsapp-web-multidevice
- Open the folder that was cloned via cmd/terminal.
- run
cd src
- run
go run . rest
(for REST API mode) - Open
http://localhost:3000
Docker (you don't need to install in required)
- Clone this repo:
git clone https://github.com/aldinokemal/go-whatsapp-web-multidevice
- Open the folder that was cloned via cmd/terminal.
- run
docker-compose up -d --build
- open
http://localhost:3000
Build your own binary
- Clone this repo
git clone https://github.com/aldinokemal/go-whatsapp-web-multidevice
- Open the folder that was cloned via cmd/terminal.
- run
cd src
- run
- Linux & MacOS:
go build -o whatsapp
- Windows (CMD / PowerShell):
go build -o whatsapp.exe
- Linux & MacOS:
- run
- Linux & MacOS:
./whatsapp rest
(for REST API mode)- run
./whatsapp --help
for more detail flags
- run
- Windows:
.\whatsapp.exe rest
(for REST API mode)- run
.\whatsapp.exe --help
for more detail flags
- run
- Linux & MacOS:
- open
http://localhost:3000
in browser
MCP Server (Model Context Protocol)
This application can also run as an MCP server, allowing AI agents and tools to interact with WhatsApp through a standardized protocol.
- Clone this repo
git clone https://github.com/aldinokemal/go-whatsapp-web-multidevice
- Open the folder that was cloned via cmd/terminal.
- run
cd src
- run
go run . mcp
or build the binary and run./whatsapp mcp
- The MCP server will start on
http://localhost:8080
by default
MCP Server Options
--host localhost
- Set the host for MCP server (default: localhost)--port 8080
- Set the port for MCP server (default: 8080)
Available MCP Tools
whatsapp_send_text
- Send text messageswhatsapp_send_contact
- Send contact cardswhatsapp_send_link
- Send links with captionswhatsapp_send_location
- Send location coordinates
MCP Endpoints
- SSE endpoint:
http://localhost:8080/sse
- Message endpoint:
http://localhost:8080/message
MCP Configuration
Make sure you have the MCP server running: ./whatsapp mcp
For AI tools that support MCP with SSE (like Cursor), add this configuration:
{
"mcpServers": {
"whatsapp": {
"url": "http://localhost:8080/sse"
}
}
}
Production Mode REST (docker)
docker run --detach --publish=3000:3000 --name=whatsapp --restart=always --volume=$(docker volume create --name=whatsapp):/app/storages aldinokemal2104/go-whatsapp-web-multidevice rest --autoreply="Dont't reply this message please"
Production Mode REST (docker compose)
create docker-compose.yml
file with the following configuration:
services:
whatsapp:
image: aldinokemal2104/go-whatsapp-web-multidevice
container_name: whatsapp
restart: always
ports:
- "3000:3000"
volumes:
- whatsapp:/app/storages
command:
- rest
- --basic-auth=admin:admin
- --port=3000
- --debug=true
- --os=Chrome
- --account-validation=false
volumes:
whatsapp:
or with env file
services:
whatsapp:
image: aldinokemal2104/go-whatsapp-web-multidevice
container_name: whatsapp
restart: always
ports:
- "3000:3000"
volumes:
- whatsapp:/app/storages
environment:
- APP_BASIC_AUTH=admin:admin
- APP_PORT=3000
- APP_DEBUG=true
- APP_OS=Chrome
- APP_ACCOUNT_VALIDATION=false
volumes:
whatsapp:
Production Mode (binary)
- download binary from release
You can fork or edit this source code !
Current API
MCP (Model Context Protocol) API
- MCP server provides standardized tools for AI agents to interact with WhatsApp
- Supports Server-Sent Events (SSE) transport
- Available tools:
whatsapp_send_text
,whatsapp_send_contact
,whatsapp_send_link
,whatsapp_send_location
- Compatible with MCP-enabled AI tools and agents
HTTP REST API
- API Specification Document.
- Check docs/openapi.yml for detailed API specifications.
- Use SwaggerEditor to visualize the API.
- Generate HTTP clients using openapi-generator.
Feature | Menu | Method | URL |
---|---|---|---|
โ | Login with Scan QR | GET | /app/login |
โ | Login With Pair Code | GET | /app/login-with-code |
โ | Logout | GET | /app/logout |
โ | Reconnect | GET | /app/reconnect |
โ | Devices | GET | /app/devices |
โ | User Info | GET | /user/info |
โ | User Avatar | GET | /user/avatar |
โ | User Change Avatar | POST | /user/avatar |
โ | User Change PushName | POST | /user/pushname |
โ | User My Groups | GET | /user/my/groups |
โ | User My Newsletter | GET | /user/my/newsletters |
โ | User My Privacy Setting | GET | /user/my/privacy |
โ | User My Contacts | GET | /user/my/contacts |
โ | Send Message | POST | /send/message |
โ | Send Image | POST | /send/image |
โ | Send Audio | POST | /send/audio |
โ | Send File | POST | /send/file |
โ | Send Video | POST | /send/video |
โ | Send Contact | POST | /send/contact |
โ | Send Link | POST | /send/link |
โ | Send Location | POST | /send/location |
โ | Send Poll / Vote | POST | /send/poll |
โ | Send Presence | POST | /send/presence |
โ | Revoke Message | POST | /message/:message_id/revoke |
โ | React Message | POST | /message/:message_id/reaction |
โ | Delete Message | POST | /message/:message_id/delete |
โ | Edit Message | POST | /message/:message_id/update |
โ | Read Message (DM) | POST | /message/:message_id/read |
โ | Star Message | POST | /message/:message_id/star |
โ | Unstar Message | POST | /message/:message_id/unstar |
โ | Join Group With Link | POST | /group/join-with-link |
โ | Leave Group | POST | /group/leave |
โ | Create Group | POST | /group |
โ | Add Participants in Group | POST | /group/participants |
โ | Remove Participant in Group | POST | /group/participants/remove |
โ | Promote Participant in Group | POST | /group/participants/promote |
โ | Demote Participant in Group | POST | /group/participants/demote |
โ | List Requested Participants in Group | GET | /group/participant-requests |
โ | Approve Requested Participant in Group | POST | /group/participant-requests/approve |
โ | Reject Requested Participant in Group | POST | /group/participant-requests/reject |
โ | Unfollow Newsletter | POST | /newsletter/unfollow |
โ
= Available
โ = Not Available Yet
User Interface
MCP UI
- Setup MCP (tested in cursor)
- Test MCP
- Successfully setup MCP
HTTP REST API UI
Description | Image |
---|---|
Homepage | ![]() |
Login | ![]() |
Login With Code | ![]() |
Send Message | ![]() |
Send Image | ![]() |
Send File | ![]() |
Send Video | ![]() |
Send Contact | ![]() |
Send Location | ![]() |
Send Audio | ![]() |
Send Poll | ![]() |
Send Presence | ![]() |
Revoke Message | ![]() |
Delete Message | ![]() |
Reaction Message | ![]() |
Edit Message | ![]() |
User Info | ![]() |
User Avatar | ![]() |
My Privacy | ![]() |
My Group | ![]() |
Create Group | ![]() |
Join Group with LInk | ![]() |
Auto Reply | ![]() |
Basic Auth Prompt | ![]() |
Manage Participant | ![]() |
My Newsletter | ![]() |
Mac OS NOTE
- Please do this if you have an error (invalid flag in pkg-config --cflags: -Xpreprocessor)
export CGO_CFLAGS_ALLOW="-Xpreprocessor"
Important
- This project is unofficial and not affiliated with WhatsApp.
- Please use official WhatsApp API to avoid any issues.
- We only able to run MCP or REST API, this is limitation from whatsmeow library. independent MCP will be available in the future.
Docker container for managing Nginx proxy hosts with a simple, powerful interface
This project comes as a pre-built docker image that enables you to easily forward to your websites running at home or otherwise, including free SSL, without having to know too much about Nginx or Letsencrypt.
Project Goal
I created this project to fill a personal need to provide users with an easy way to accomplish reverse proxying hosts with SSL termination and it had to be so easy that a monkey could do it. This goal hasn't changed. While there might be advanced options they are optional and the project should be as simple as possible so that the barrier for entry here is low.
Features
- Beautiful and Secure Admin Interface based on Tabler
- Easily create forwarding domains, redirections, streams and 404 hosts without knowing anything about Nginx
- Free SSL using Let's Encrypt or provide your own custom SSL certificates
- Access Lists and basic HTTP Authentication for your hosts
- Advanced Nginx configuration available for super users
- User management, permissions and audit log
Hosting your home network
I won't go in to too much detail here but here are the basics for someone new to this self-hosted world.
- Your home router will have a Port Forwarding section somewhere. Log in and find it
- Add port forwarding for port 80 and 443 to the server hosting this project
- Configure your domain name details to point to your home, either with a static ip or a service like DuckDNS or Amazon Route53
- Use the Nginx Proxy Manager as your gateway to forward to your other web based services
Quick Setup
- Install Docker and Docker-Compose
- Create a docker-compose.yml file similar to this:
services:
app:
image: 'docker.io/jc21/nginx-proxy-manager:latest'
restart: unless-stopped
ports:
- '80:80'
- '81:81'
- '443:443'
volumes:
- ./data:/data
- ./letsencrypt:/etc/letsencrypt
This is the bare minimum configuration required. See the documentation for more.
- Bring up your stack by running
docker-compose up -d
# If using docker-compose-plugin
docker compose up -d
- Log in to the Admin UI
When your docker container is running, connect to it on port 81
for the admin interface. Sometimes this can take a little bit because of the entropy of keys.
Default Admin User:
Email: [email protected]
Password: changeme
Immediately after logging in with this default user you will be asked to modify your details and change your password.
Contributing
All are welcome to create pull requests for this project, against the develop
branch. Official releases are created from the master
branch.
CI is used in this project. All PR's must pass before being considered. After passing, docker builds for PR's are available on dockerhub for manual verifications.
Documentation within the develop
branch is available for preview at https://develop.nginxproxymanager.com
Contributors
Special thanks to all of our contributors.
Getting Support
ๅฐ็บขไนฆ็ฌ่ฎฐ | ่ฏ่ฎบ็ฌ่ซใๆ้ณ่ง้ข | ่ฏ่ฎบ็ฌ่ซใๅฟซๆ่ง้ข | ่ฏ่ฎบ็ฌ่ซใB ็ซ่ง้ข ๏ฝ ่ฏ่ฎบ็ฌ่ซใๅพฎๅๅธๅญ ๏ฝ ่ฏ่ฎบ็ฌ่ซใ็พๅบฆ่ดดๅงๅธๅญ ๏ฝ ็พๅบฆ่ดดๅง่ฏ่ฎบๅๅค็ฌ่ซ | ็ฅไน้ฎ็ญๆ็ซ ๏ฝ่ฏ่ฎบ็ฌ่ซ
๐ฅ MediaCrawler - ่ชๅชไฝๅนณๅฐ็ฌ่ซ ๐ท๏ธ
ๅ ่ดฃๅฃฐๆ๏ผ
ๅคงๅฎถ่ฏทไปฅๅญฆไน ไธบ็ฎ็ไฝฟ็จๆฌไปๅบโ ๏ธโ ๏ธโ ๏ธโ ๏ธ๏ผ็ฌ่ซ่ฟๆณ่ฟ่ง็ๆกไปถ
ๆฌไปๅบ็ๆๆๅ ๅฎนไป ไพๅญฆไน ๅๅ่ไน็จ๏ผ็ฆๆญข็จไบๅไธ็จ้ใไปปไฝไบบๆ็ป็ปไธๅพๅฐๆฌไปๅบ็ๅ ๅฎน็จไบ้ๆณ็จ้ๆไพต็ฏไปไบบๅๆณๆ็ใๆฌไปๅบๆๆถๅ็็ฌ่ซๆๆฏไป ็จไบๅญฆไน ๅ็ ็ฉถ๏ผไธๅพ็จไบๅฏนๅ ถไปๅนณๅฐ่ฟ่กๅคง่งๆจก็ฌ่ซๆๅ ถไป้ๆณ่กไธบใๅฏนไบๅ ไฝฟ็จๆฌไปๅบๅ ๅฎน่ๅผ่ตท็ไปปไฝๆณๅพ่ดฃไปป๏ผๆฌไปๅบไธๆฟๆ ไปปไฝ่ดฃไปปใไฝฟ็จๆฌไปๅบ็ๅ ๅฎนๅณ่กจ็คบๆจๅๆๆฌๅ ่ดฃๅฃฐๆ็ๆๆๆกๆฌพๅๆกไปถใ
็นๅปๆฅ็ๆดไธบ่ฏฆ็ป็ๅ ่ดฃๅฃฐๆใ็นๅป่ทณ่ฝฌ
๐ ้กน็ฎ็ฎไป
ไธไธชๅ่ฝๅผบๅคง็ๅคๅนณๅฐ่ชๅชไฝๆฐๆฎ้้ๅทฅๅ ท๏ผๆฏๆๅฐ็บขไนฆใๆ้ณใๅฟซๆใB็ซใๅพฎๅใ่ดดๅงใ็ฅไน็ญไธปๆตๅนณๅฐ็ๅ ฌๅผไฟกๆฏๆๅใ
๐ง ๆๆฏๅ็
- ๆ ธๅฟๆๆฏ๏ผๅบไบ Playwright ๆต่งๅจ่ชๅจๅๆกๆถ็ปๅฝไฟๅญ็ปๅฝๆ
- ๆ ้JS้ๅ๏ผๅฉ็จไฟ็็ปๅฝๆ็ๆต่งๅจไธไธๆ็ฏๅข๏ผ้่ฟ JS ่กจ่พพๅผ่ทๅ็ญพๅๅๆฐ
- ไผๅฟ็น็น๏ผๆ ้้ๅๅคๆ็ๅ ๅฏ็ฎๆณ๏ผๅคงๅน ้ไฝๆๆฏ้จๆง
โจ ๅ่ฝ็นๆง
ๅนณๅฐ | ๅ ณ้ฎ่ฏๆ็ดข | ๆๅฎๅธๅญID็ฌๅ | ไบ็บง่ฏ่ฎบ | ๆๅฎๅไฝ่ ไธป้กต | ็ปๅฝๆ็ผๅญ | IPไปฃ็ๆฑ | ็ๆ่ฏ่ฎบ่ฏไบๅพ |
---|---|---|---|---|---|---|---|
ๅฐ็บขไนฆ | โ | โ | โ | โ | โ | โ | โ |
ๆ้ณ | โ | โ | โ | โ | โ | โ | โ |
ๅฟซๆ | โ | โ | โ | โ | โ | โ | โ |
B ็ซ | โ | โ | โ | โ | โ | โ | โ |
ๅพฎๅ | โ | โ | โ | โ | โ | โ | โ |
่ดดๅง | โ | โ | โ | โ | โ | โ | โ |
็ฅไน | โ | โ | โ | โ | โ | โ | โ |
๐ ๐ MediaCrawlerPro ้็ฃ ๅๅธ๏ผๆดๅค็ๅ่ฝ๏ผๆดๅฅฝ็ๆถๆ่ฎพ่ฎก๏ผ
๐ MediaCrawlerPro ้็ฃ ๅๅธ๏ผ
ไธๆณจไบๅญฆไน ๆ็้กน็ฎ็ๆถๆ่ฎพ่ฎก๏ผไธไป ไป ๆฏ็ฌ่ซๆๆฏ๏ผPro ็ๆฌ็ไปฃ็ ่ฎพ่ฎกๆ่ทฏๅๆ ทๅผๅพๆทฑๅ ฅๅญฆไน ๏ผ
MediaCrawlerPro ็ธ่พไบๅผๆบ็ๆฌ็ๆ ธๅฟไผๅฟ๏ผ
๐ฏ ๆ ธๅฟๅ่ฝๅ็บง
- โ ๆญ็น็ปญ็ฌๅ่ฝ๏ผ้็น็นๆง๏ผ
- โ ๅค่ดฆๅท + IPไปฃ็ๆฑ ๆฏๆ๏ผ้็น็นๆง๏ผ
- โ ๅป้ค Playwright ไพ่ต๏ผไฝฟ็จๆด็ฎๅ
- โ ๅฎๆด Linux ็ฏๅขๆฏๆ
๐๏ธ ๆถๆ่ฎพ่ฎกไผๅ
- โ ไปฃ็ ้ๆไผๅ๏ผๆดๆ่ฏปๆ็ปดๆค๏ผ่งฃ่ฆ JS ็ญพๅ้ป่พ๏ผ
- โ ไผไธ็บงไปฃ็ ่ดจ้๏ผ้ๅๆๅปบๅคงๅ็ฌ่ซ้กน็ฎ
- โ ๅฎ็พๆถๆ่ฎพ่ฎก๏ผ้ซๆฉๅฑๆง๏ผๆบ็ ๅญฆไน ไปทๅผๆดๅคง
๐ ้ขๅคๅ่ฝ
- โ ่ชๅชไฝ่ง้ขไธ่ฝฝๅจๆก้ข็ซฏ๏ผ้ๅๅญฆไน ๅ จๆ ๅผๅ๏ผ
- โ ๅคๅนณๅฐ้ฆ้กตไฟกๆฏๆตๆจ่๏ผHomeFeed๏ผ
- ๅบไบ่ชๅชไฝๅนณๅฐ็AI Agentๆญฃๅจๅผๅไธญ ๐๐
็นๅปๆฅ็๏ผMediaCrawlerPro ้กน็ฎไธป้กต ๆดๅคไป็ป
๐ ๅฟซ้ๅผๅง
๐ก ๅผๆบไธๆ๏ผๅฆๆ่ฟไธช้กน็ฎๅฏนๆจๆๅธฎๅฉ๏ผ่ฏท็ปไธช โญ Star ๆฏๆไธไธ๏ผ
๐ ๅ็ฝฎไพ่ต
๐ uv ๅฎ่ฃ ๏ผๆจ่๏ผ
ๅจ่ฟ่กไธไธๆญฅๆไฝไนๅ๏ผ่ฏท็กฎไฟ็ต่ไธๅทฒ็ปๅฎ่ฃ ไบ uv๏ผ
- ๅฎ่ฃ ๅฐๅ๏ผuv ๅฎๆนๅฎ่ฃ ๆๅ
- ้ช่ฏๅฎ่ฃ
๏ผ็ป็ซฏ่พๅ
ฅๅฝไปค
uv --version
๏ผๅฆๆๆญฃๅธธๆพ็คบ็ๆฌๅท๏ผ่ฏๆๅทฒ็ปๅฎ่ฃ ๆๅ - ๆจ่็็ฑ๏ผuv ๆฏ็ฎๅๆๅผบ็ Python ๅ ็ฎก็ๅทฅๅ ท๏ผ้ๅบฆๅฟซใไพ่ต่งฃๆๅ็กฎ
๐ข Node.js ๅฎ่ฃ
้กน็ฎไพ่ต Node.js๏ผ่ฏทๅๅพๅฎ็ฝไธ่ฝฝๅฎ่ฃ ๏ผ
- ไธ่ฝฝๅฐๅ๏ผhttps://nodejs.org/en/download/
- ็ๆฌ่ฆๆฑ๏ผ>= 16.0.0
๐ฆ Python ๅ ๅฎ่ฃ
# ่ฟๅ
ฅ้กน็ฎ็ฎๅฝ
cd MediaCrawler
# ไฝฟ็จ uv sync ๅฝไปคๆฅไฟ่ฏ python ็ๆฌๅ็ธๅ
ณไพ่ตๅ
็ไธ่ดๆง
uv sync
๐ ๆต่งๅจ้ฉฑๅจๅฎ่ฃ
# ๅฎ่ฃ
ๆต่งๅจ้ฉฑๅจ
uv run playwright install
๐ก ๆ็คบ๏ผMediaCrawler ็ฎๅๅทฒ็ปๆฏๆไฝฟ็จ playwright ่ฟๆฅไฝ ๆฌๅฐ็ Chrome ๆต่งๅจไบ๏ผไธไบๅ ไธบ Webdriver ๅฏผ่ด็้ฎ้ข่ฟๅ่่งฃไบใ
็ฎๅๅผๆพไบ
xhs
ๅdy
่ฟไธคไธชไฝฟ็จ CDP ็ๆนๅผ่ฟๆฅๆฌๅฐๆต่งๅจ๏ผๅฆๆ้่ฆ๏ผๆฅ็config/base_config.py
ไธญ็้ ็ฝฎ้กนใ
๐ ่ฟ่ก็ฌ่ซ็จๅบ
# ้กน็ฎ้ป่ฎคๆฏๆฒกๆๅผๅฏ่ฏ่ฎบ็ฌๅๆจกๅผ๏ผๅฆ้่ฏ่ฎบ่ฏทๅจ config/base_config.py ไธญ็ ENABLE_GET_COMMENTS ๅ้ไฟฎๆน
# ไธไบๅ
ถไปๆฏๆ้กน๏ผไนๅฏไปฅๅจ config/base_config.py ๆฅ็ๅ่ฝ๏ผๅ็ๆไธญๆๆณจ้
# ไป้
็ฝฎๆไปถไธญ่ฏปๅๅ
ณ้ฎ่ฏๆ็ดข็ธๅ
ณ็ๅธๅญๅนถ็ฌๅๅธๅญไฟกๆฏไธ่ฏ่ฎบ
uv run main.py --platform xhs --lt qrcode --type search
# ไป้
็ฝฎๆไปถไธญ่ฏปๅๆๅฎ็ๅธๅญIDๅ่กจ่ทๅๆๅฎๅธๅญ็ไฟกๆฏไธ่ฏ่ฎบไฟกๆฏ
uv run main.py --platform xhs --lt qrcode --type detail
# ๆๅผๅฏนๅบAPPๆซไบ็ปด็ ็ปๅฝ
# ๅ
ถไปๅนณๅฐ็ฌ่ซไฝฟ็จ็คบไพ๏ผๆง่กไธ้ข็ๅฝไปคๆฅ็
uv run main.py --help
๐ ไฝฟ็จ Python ๅ็ venv ็ฎก็็ฏๅข๏ผไธๆจ่๏ผ
ๅๅปบๅนถๆฟๆดป Python ่ๆ็ฏๅข
ๅฆๆๆฏ็ฌๅๆ้ณๅ็ฅไน๏ผ้่ฆๆๅๅฎ่ฃ nodejs ็ฏๅข๏ผ็ๆฌๅคงไบ็ญไบ๏ผ
16
ๅณๅฏ
# ่ฟๅ
ฅ้กน็ฎๆ น็ฎๅฝ
cd MediaCrawler
# ๅๅปบ่ๆ็ฏๅข
# ๆ็ python ็ๆฌๆฏ๏ผ3.9.6๏ผrequirements.txt ไธญ็ๅบๆฏๅบไบ่ฟไธช็ๆฌ็
# ๅฆๆๆฏๅ
ถไป python ็ๆฌ๏ผๅฏ่ฝ requirements.txt ไธญ็ๅบไธๅ
ผๅฎน๏ผ้่ช่ก่งฃๅณ
python -m venv venv
# macOS & Linux ๆฟๆดป่ๆ็ฏๅข
source venv/bin/activate
# Windows ๆฟๆดป่ๆ็ฏๅข
venv\Scripts\activate
ๅฎ่ฃ ไพ่ตๅบ
pip install -r requirements.txt
ๅฎ่ฃ playwright ๆต่งๅจ้ฉฑๅจ
playwright install
่ฟ่ก็ฌ่ซ็จๅบ๏ผๅ็็ฏๅข๏ผ
# ้กน็ฎ้ป่ฎคๆฏๆฒกๆๅผๅฏ่ฏ่ฎบ็ฌๅๆจกๅผ๏ผๅฆ้่ฏ่ฎบ่ฏทๅจ config/base_config.py ไธญ็ ENABLE_GET_COMMENTS ๅ้ไฟฎๆน
# ไธไบๅ
ถไปๆฏๆ้กน๏ผไนๅฏไปฅๅจ config/base_config.py ๆฅ็ๅ่ฝ๏ผๅ็ๆไธญๆๆณจ้
# ไป้
็ฝฎๆไปถไธญ่ฏปๅๅ
ณ้ฎ่ฏๆ็ดข็ธๅ
ณ็ๅธๅญๅนถ็ฌๅๅธๅญไฟกๆฏไธ่ฏ่ฎบ
python main.py --platform xhs --lt qrcode --type search
# ไป้
็ฝฎๆไปถไธญ่ฏปๅๆๅฎ็ๅธๅญIDๅ่กจ่ทๅๆๅฎๅธๅญ็ไฟกๆฏไธ่ฏ่ฎบไฟกๆฏ
python main.py --platform xhs --lt qrcode --type detail
# ๆๅผๅฏนๅบAPPๆซไบ็ปด็ ็ปๅฝ
# ๅ
ถไปๅนณๅฐ็ฌ่ซไฝฟ็จ็คบไพ๏ผๆง่กไธ้ข็ๅฝไปคๆฅ็
python main.py --help
๐พ ๆฐๆฎไฟๅญ
ๆฏๆๅค็งๆฐๆฎๅญๅจๆนๅผ๏ผ
- MySQL ๆฐๆฎๅบ๏ผๆฏๆๅ
ณ็ณปๅๆฐๆฎๅบ MySQL ไธญไฟๅญ๏ผ้่ฆๆๅๅๅปบๆฐๆฎๅบ๏ผ
- ๆง่ก
python db.py
ๅๅงๅๆฐๆฎๅบ่กจ็ปๆ๏ผๅชๅจ้ฆๆฌกๆง่ก๏ผ
- ๆง่ก
- CSV ๆไปถ๏ผๆฏๆไฟๅญๅฐ CSV ไธญ๏ผ
data/
็ฎๅฝไธ๏ผ - JSON ๆไปถ๏ผๆฏๆไฟๅญๅฐ JSON ไธญ๏ผ
data/
็ฎๅฝไธ๏ผ
๐ MediaCrawlerPro ้็ฃ ๅๅธ ๐๏ผๆดๅค็ๅ่ฝ๏ผๆดๅฅฝ็ๆถๆ่ฎพ่ฎก๏ผ
๐ค ็คพๅบไธๆฏๆ
๐ฌ ไบคๆต็พค็ป
- ๅพฎไฟกไบคๆต็พค๏ผ็นๅปๅ ๅ ฅ
๐ ๆๆกฃไธๆ็จ
- ๅจ็บฟๆๆกฃ๏ผMediaCrawler ๅฎๆดๆๆกฃ
- ็ฌ่ซๆ็จ๏ผCrawlerTutorial ๅ ่ดนๆ็จ
ๅ ถไปๅธธ่ง้ฎ้ขๅฏไปฅๆฅ็ๅจ็บฟๆๆกฃ
ๅจ็บฟๆๆกฃๅ ๅซไฝฟ็จๆนๆณใๅธธ่ง้ฎ้ขใๅ ๅ ฅ้กน็ฎไบคๆต็พค็ญใ MediaCrawlerๅจ็บฟๆๆกฃ
ไฝ่ ๆไพ็็ฅ่ฏๆๅก
ๅฆๆๆณๅฟซ้ๅ ฅ้จๅๅญฆไน ่ฏฅ้กน็ฎ็ไฝฟ็จใๆบ็ ๆถๆ่ฎพ่ฎก็ญใๅญฆไน ็ผ็จๆๆฏใไบฆๆ่ ๆณไบ่งฃMediaCrawlerPro็ๆบไปฃ็ ่ฎพ่ฎกๅฏไปฅ็ไธๆ็็ฅ่ฏไป่ดนๆ ็ฎใ
ไฝ่ ็็ฅ่ฏไป่ดนๆ ็ฎไป็ป
โญ Star ่ถๅฟๅพ
ๅฆๆ่ฟไธช้กน็ฎๅฏนๆจๆๅธฎๅฉ๏ผ่ฏท็ปไธช โญ Star ๆฏๆไธไธ๏ผ่ฎฉๆดๅค็ไบบ็ๅฐ MediaCrawler๏ผ
๐ฐ ่ตๅฉๅๅฑ็คบ

**Swiftproxy** - 90M+ ๅ จ็้ซ่ดจ้็บฏๅไฝๅฎ IP๏ผๆณจๅๅฏ้ขๅ ่ดน 500MB ๆต่ฏๆต้๏ผๅจๆๆต้ไธ่ฟๆ๏ผ > ไธๅฑๆๆฃ็ ๏ผ**GHB5** ็ซไบซไนๆไผๆ ๏ผ
Sider - ๅ จ็ฝๆ็ซ็ ChatGPT ๆไปถ๏ผไฝ้ชๆๆปก๏ผ
๐ค ๆไธบ่ตๅฉ่
ๆไธบ่ตๅฉ่ ๏ผๅฏไปฅๅฐๆจ็ไบงๅๅฑ็คบๅจ่ฟ้๏ผๆฏๅคฉ่ทๅพๅคง้ๆๅ ๏ผ
่็ณปๆนๅผ๏ผ
- ๅพฎไฟก๏ผ
yzglan
- ้ฎ็ฎฑ๏ผ
[email protected]
๐ ๅ่
- ๅฐ็บขไนฆๅฎขๆท็ซฏ๏ผReaJason ็ xhs ไปๅบ
- ็ญไฟก่ฝฌๅ๏ผSmsForwarder ๅ่ไปๅบ
- ๅ ็ฝ็ฉฟ้ๅทฅๅ ท๏ผngrok ๅฎๆนๆๆกฃ
ๅ ่ดฃๅฃฐๆ
1. ้กน็ฎ็ฎ็ไธๆง่ดจ
ๆฌ้กน็ฎ๏ผไปฅไธ็ฎ็งฐโๆฌ้กน็ฎโ๏ผๆฏไฝไธบไธไธชๆๆฏ็ ็ฉถไธๅญฆไน ๅทฅๅ ท่ๅๅปบ็๏ผๆจๅจๆข็ดขๅๅญฆไน ็ฝ็ปๆฐๆฎ้้ๆๆฏใๆฌ้กน็ฎไธๆณจไบ่ชๅชไฝๅนณๅฐ็ๆฐๆฎ็ฌๅๆๆฏ็ ็ฉถ๏ผๆจๅจๆไพ็ปๅญฆไน ่ ๅ็ ็ฉถ่ ไฝไธบๆๆฏไบคๆตไน็จใ
2. ๆณๅพๅ่งๆงๅฃฐๆ
ๆฌ้กน็ฎๅผๅ่ ๏ผไปฅไธ็ฎ็งฐโๅผๅ่ โ๏ผ้้ๆ้็จๆทๅจไธ่ฝฝใๅฎ่ฃ ๅไฝฟ็จๆฌ้กน็ฎๆถ๏ผไธฅๆ ผ้ตๅฎไธญๅไบบๆฐๅ ฑๅๅฝ็ธๅ ณๆณๅพๆณ่ง๏ผๅ ๆฌไฝไธ้ไบใไธญๅไบบๆฐๅ ฑๅๅฝ็ฝ็ปๅฎๅ จๆณใใใไธญๅไบบๆฐๅ ฑๅๅฝๅ้ด่ฐๆณใ็ญๆๆ้็จ็ๅฝๅฎถๆณๅพๅๆฟ็ญใ็จๆทๅบ่ช่กๆฟๆ ไธๅๅ ไฝฟ็จๆฌ้กน็ฎ่ๅฏ่ฝๅผ่ตท็ๆณๅพ่ดฃไปปใ
3. ไฝฟ็จ็ฎ็้ๅถ
ๆฌ้กน็ฎไธฅ็ฆ็จไบไปปไฝ้ๆณ็ฎ็ๆ้ๅญฆไน ใ้็ ็ฉถ็ๅไธ่กไธบใๆฌ้กน็ฎไธๅพ็จไบไปปไฝๅฝขๅผ็้ๆณไพตๅ ฅไปไบบ่ฎก็ฎๆบ็ณป็ป๏ผไธๅพ็จไบไปปไฝไพต็ฏไปไบบ็ฅ่ฏไบงๆๆๅ ถไปๅๆณๆ็็่กไธบใ็จๆทๅบไฟ่ฏๅ ถไฝฟ็จๆฌ้กน็ฎ็็ฎ็็บฏๅฑไธชไบบๅญฆไน ๅๆๆฏ็ ็ฉถ๏ผไธๅพ็จไบไปปไฝๅฝขๅผ็้ๆณๆดปๅจใ
4. ๅ ่ดฃๅฃฐๆ
ๅผๅ่ ๅทฒๅฐฝๆๅคงๅชๅ็กฎไฟๆฌ้กน็ฎ็ๆญฃๅฝๆงๅๅฎๅ จๆง๏ผไฝไธๅฏน็จๆทไฝฟ็จๆฌ้กน็ฎๅฏ่ฝๅผ่ตท็ไปปไฝๅฝขๅผ็็ดๆฅๆ้ดๆฅๆๅคฑๆฟๆ ่ดฃไปปใๅ ๆฌไฝไธ้ไบ็ฑไบไฝฟ็จๆฌ้กน็ฎ่ๅฏผ่ด็ไปปไฝๆฐๆฎไธขๅคฑใ่ฎพๅคๆๅใๆณๅพ่ฏ่ฎผ็ญใ
5. ็ฅ่ฏไบงๆๅฃฐๆ
ๆฌ้กน็ฎ็็ฅ่ฏไบงๆๅฝๅผๅ่ ๆๆใๆฌ้กน็ฎๅๅฐ่ไฝๆๆณๅๅฝ้ ่ไฝๆๆก็บฆไปฅๅๅ ถไป็ฅ่ฏไบงๆๆณๅพๅๆก็บฆ็ไฟๆคใ็จๆทๅจ้ตๅฎๆฌๅฃฐๆๅ็ธๅ ณๆณๅพๆณ่ง็ๅๆไธ๏ผๅฏไปฅไธ่ฝฝๅไฝฟ็จๆฌ้กน็ฎใ
6. ๆ็ป่งฃ้ๆ
ๅ ณไบๆฌ้กน็ฎ็ๆ็ป่งฃ้ๆๅฝๅผๅ่ ๆๆใๅผๅ่ ไฟ็้ๆถๆดๆนๆๆดๆฐๆฌๅ ่ดฃๅฃฐๆ็ๆๅฉ๏ผๆไธๅฆ่ก้็ฅใ
๐ ่ด่ฐข
JetBrains ๅผๆบ่ฎธๅฏ่ฏๆฏๆ
ๆ่ฐข JetBrains ไธบๆฌ้กน็ฎๆไพๅ ่ดน็ๅผๆบ่ฎธๅฏ่ฏๆฏๆ๏ผ
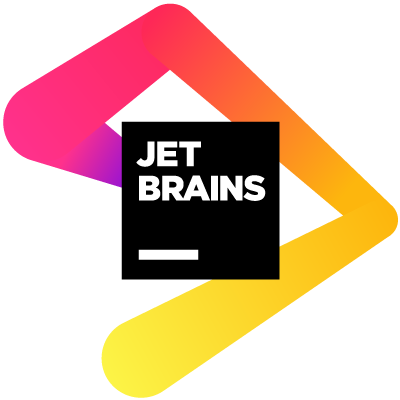
12 weeks, 26 lessons, 52 quizzes, classic Machine Learning for all
Machine Learning for Beginners - A Curriculum
๐ Travel around the world as we explore Machine Learning by means of world cultures ๐
Cloud Advocates at Microsoft are pleased to offer a 12-week, 26-lesson curriculum all about Machine Learning. In this curriculum, you will learn about what is sometimes called classic machine learning, using primarily Scikit-learn as a library and avoiding deep learning, which is covered in our AI for Beginners' curriculum. Pair these lessons with our 'Data Science for Beginners' curriculum, as well!
Travel with us around the world as we apply these classic techniques to data from many areas of the world. Each lesson includes pre- and post-lesson quizzes, written instructions to complete the lesson, a solution, an assignment, and more. Our project-based pedagogy allows you to learn while building, a proven way for new skills to 'stick'.
โ๏ธ Hearty thanks to our authors Jen Looper, Stephen Howell, Francesca Lazzeri, Tomomi Imura, Cassie Breviu, Dmitry Soshnikov, Chris Noring, Anirban Mukherjee, Ornella Altunyan, Ruth Yakubu and Amy Boyd
๐จ Thanks as well to our illustrators Tomomi Imura, Dasani Madipalli, and Jen Looper
๐ Special thanks ๐ to our Microsoft Student Ambassador authors, reviewers, and content contributors, notably Rishit Dagli, Muhammad Sakib Khan Inan, Rohan Raj, Alexandru Petrescu, Abhishek Jaiswal, Nawrin Tabassum, Ioan Samuila, and Snigdha Agarwal
๐คฉ Extra gratitude to Microsoft Student Ambassadors Eric Wanjau, Jasleen Sondhi, and Vidushi Gupta for our R lessons!
Getting Started
Follow these steps:
- Fork the Repository: Click on the "Fork" button at the top-right corner of this page.
- Clone the Repository:
git clone https://github.com/microsoft/ML-For-Beginners.git
find all additional resources for this course in our Microsoft Learn collection
Students, to use this curriculum, fork the entire repo to your own GitHub account and complete the exercises on your own or with a group:
- Start with a pre-lecture quiz.
- Read the lecture and complete the activities, pausing and reflecting at each knowledge check.
- Try to create the projects by comprehending the lessons rather than running the solution code; however that code is available in the
/solution
folders in each project-oriented lesson. - Take the post-lecture quiz.
- Complete the challenge.
- Complete the assignment.
- After completing a lesson group, visit the Discussion Board and "learn out loud" by filling out the appropriate PAT rubric. A 'PAT' is a Progress Assessment Tool that is a rubric you fill out to further your learning. You can also react to other PATs so we can learn together.
For further study, we recommend following these Microsoft Learn modules and learning paths.
Teachers, we have included some suggestions on how to use this curriculum.
Video walkthroughs
Some of the lessons are available as short form video. You can find all these in-line in the lessons, or on the ML for Beginners playlist on the Microsoft Developer YouTube channel by clicking the image below.
Meet the Team
Gif by Mohit Jaisal
๐ฅ Click the image above for a video about the project and the folks who created it!
Pedagogy
We have chosen two pedagogical tenets while building this curriculum: ensuring that it is hands-on project-based and that it includes frequent quizzes. In addition, this curriculum has a common theme to give it cohesion.
By ensuring that the content aligns with projects, the process is made more engaging for students and retention of concepts will be augmented. In addition, a low-stakes quiz before a class sets the intention of the student towards learning a topic, while a second quiz after class ensures further retention. This curriculum was designed to be flexible and fun and can be taken in whole or in part. The projects start small and become increasingly complex by the end of the 12-week cycle. This curriculum also includes a postscript on real-world applications of ML, which can be used as extra credit or as a basis for discussion.
Find our Code of Conduct, Contributing, and Translation guidelines. We welcome your constructive feedback!
Each lesson includes
- optional sketchnote
- optional supplemental video
- video walkthrough (some lessons only)
- pre-lecture warmup quiz
- written lesson
- for project-based lessons, step-by-step guides on how to build the project
- knowledge checks
- a challenge
- supplemental reading
- assignment
- post-lecture quiz
A note about languages: These lessons are primarily written in Python, but many are also available in R. To complete an R lesson, go to the
/solution
folder and look for R lessons. They include an .rmd extension that represents an R Markdown file which can be simply defined as an embedding ofcode chunks
(of R or other languages) and aYAML header
(that guides how to format outputs such as PDF) in aMarkdown document
. As such, it serves as an exemplary authoring framework for data science since it allows you to combine your code, its output, and your thoughts by allowing you to write them down in Markdown. Moreover, R Markdown documents can be rendered to output formats such as PDF, HTML, or Word.
A note about quizzes: All quizzes are contained in Quiz App folder, for 52 total quizzes of three questions each. They are linked from within the lessons but the quiz app can be run locally; follow the instruction in the
quiz-app
folder to locally host or deploy to Azure.
Lesson Number | Topic | Lesson Grouping | Learning Objectives | Linked Lesson | Author |
---|---|---|---|---|---|
01 | Introduction to machine learning | Introduction | Learn the basic concepts behind machine learning | Lesson | Muhammad |
02 | The History of machine learning | Introduction | Learn the history underlying this field | Lesson | Jen and Amy |
03 | Fairness and machine learning | Introduction | What are the important philosophical issues around fairness that students should consider when building and applying ML models? | Lesson | Tomomi |
04 | Techniques for machine learning | Introduction | What techniques do ML researchers use to build ML models? | Lesson | Chris and Jen |
05 | Introduction to regression | Regression | Get started with Python and Scikit-learn for regression models |
|
|
06 | North American pumpkin prices ๐ | Regression | Visualize and clean data in preparation for ML |
|
|
07 | North American pumpkin prices ๐ | Regression | Build linear and polynomial regression models |
|
|
08 | North American pumpkin prices ๐ | Regression | Build a logistic regression model |
|
|
09 | A Web App ๐ | Web App | Build a web app to use your trained model | Python | Jen |
10 | Introduction to classification | Classification | Clean, prep, and visualize your data; introduction to classification |
|
|
11 | Delicious Asian and Indian cuisines ๐ | Classification | Introduction to classifiers |
|
|
12 | Delicious Asian and Indian cuisines ๐ | Classification | More classifiers |
|
|
13 | Delicious Asian and Indian cuisines ๐ | Classification | Build a recommender web app using your model | Python | Jen |
14 | Introduction to clustering | Clustering | Clean, prep, and visualize your data; Introduction to clustering |
|
|
15 | Exploring Nigerian Musical Tastes ๐ง | Clustering | Explore the K-Means clustering method |
|
|
16 | Introduction to natural language processing โ๏ธ | Natural language processing | Learn the basics about NLP by building a simple bot | Python | Stephen |
17 | Common NLP Tasks โ๏ธ | Natural language processing | Deepen your NLP knowledge by understanding common tasks required when dealing with language structures | Python | Stephen |
18 | Translation and sentiment analysis โฅ๏ธ | Natural language processing | Translation and sentiment analysis with Jane Austen | Python | Stephen |
19 | Romantic hotels of Europe โฅ๏ธ | Natural language processing | Sentiment analysis with hotel reviews 1 | Python | Stephen |
20 | Romantic hotels of Europe โฅ๏ธ | Natural language processing | Sentiment analysis with hotel reviews 2 | Python | Stephen |
21 | Introduction to time series forecasting | Time series | Introduction to time series forecasting | Python | Francesca |
22 | โก๏ธ World Power Usage โก๏ธ - time series forecasting with ARIMA | Time series | Time series forecasting with ARIMA | Python | Francesca |
23 | โก๏ธ World Power Usage โก๏ธ - time series forecasting with SVR | Time series | Time series forecasting with Support Vector Regressor | Python | Anirban |
24 | Introduction to reinforcement learning | Reinforcement learning | Introduction to reinforcement learning with Q-Learning | Python | Dmitry |
25 | Help Peter avoid the wolf! ๐บ | Reinforcement learning | Reinforcement learning Gym | Python | Dmitry |
Postscript | Real-World ML scenarios and applications | ML in the Wild | Interesting and revealing real-world applications of classical ML | Lesson | Team |
Postscript | Model Debugging in ML using RAI dashboard | ML in the Wild | Model Debugging in Machine Learning using Responsible AI dashboard components | Lesson | Ruth Yakubu |
find all additional resources for this course in our Microsoft Learn collection
Offline access
You can run this documentation offline by using Docsify. Fork this repo, install Docsify on your local machine, and then in the root folder of this repo, type docsify serve
. The website will be served on port 3000 on your localhost: localhost:3000
.
PDFs
Find a pdf of the curriculum with links here.
Help Wanted
Would you like to contribute a translation? Please read our translation guidelines and add a templated issue to manage the workload here.
๐ Other Courses
Our team produces other courses! Check out:
- Generative AI for Beginners
- Generative AI for Beginners .NET
- Generative AI with JavaScript
- AI for Beginners
- Data Science for Beginners
- ML for Beginners
- Cybersecurity for Beginners
- Web Dev for Beginners
- IoT for Beginners
- XR Development for Beginners
- Mastering GitHub Copilot for Paired Programming
- Mastering GitHub Copilot for C#/.NET Developers
- Choose Your Own Copilot Adventure
All the open source AI Agents hosted on the oTTomator Live Agent Studio platform!
What is the Live Agent Studio?
The Live Agent Studio is a community-driven platform developed by oTTomator for you to explore cutting-edge AI agents and learn how to implement them for yourself or your business! All agents on this platform are open source and, over time, will cover a very large variety of use cases.
The goal with the studio is to build an educational platform for you to learn how to do incredible things with AI, while still providing practical value so that youโll want to use the agents just for the sake of what they can do for you!
This platform is still in beta โ expect longer response times under load, a rapidly growing agent library over the coming months, and a lot more content on this platform soon on Cole Medinโs YouTube channel!
What is this Repository for?
This repository contains the source code/workflow JSON for all the agents on the Live Agent Studio! Every agent being added to the platform is currently be open sourced here so we can not only create a curated collection of cutting-edge agents together as a community, but also learn from one another!
Tokens
Most agents on the Live Agent Studio cost tokens to use, which are purchasable on the platform. However, when you first sign in you are given some tokens to start so you can use the agents free of charge! The biggest reason agents cost tokens is that we pay for the LLM usage since we host all the agents developed by you and the rest of the community!
Future Plans
As the Live Agent Studio develops, it will become the go-to place to stay on top of what is possible with AI agents! Anytime there is a new AI technology, groundbreaking agent research, or a new tool/library to build agents with, itโll be featured through agents on the platform. Itโs a tall order, but we have big plans for the oTTomator community, and weโre confident we can grow to accomplish this!
FAQ
I want to build an agent to showcase in the Live Agent Studio! How do I do that?
Head on over here to learn how to build an agent for the platform:
Also check out the sample n8n agent for a starting point of building an n8n agent for the Live Agent Studio, and the sample Python agent for Python.
How many tokens does it cost to use an agent?
Each agent will charge tokens per prompt. The number of tokens depends on the agent, as some agents use larger LLMs, some call LLMs multiple times, and some use paid APIs.
Where can I go to talk about all these agents and get help implementing them myself?
Head on over to our Think Tank community and feel free to make a post!
ยฉ 2024 Live Agent Studio. All rights reserved.
Created by oTTomator
Master programming by recreating your favorite technologies from scratch.
Build your own <insert-technology-here>
This repository is a compilation of well-written, step-by-step guides for re-creating our favorite technologies from scratch.
What I cannot create, I do not understand โ Richard Feynman.
It's a great way to learn.
- 3D Renderer
- Augmented Reality
- BitTorrent Client
- Blockchain / Cryptocurrency
- Bot
- Command-Line Tool
- Database
- Docker
- Emulator / Virtual Machine
- Front-end Framework / Library
- Game
- Git
- Network Stack
- Neural Network
- Operating System
- Physics Engine
- Programming Language
- Regex Engine
- Search Engine
- Shell
- Template Engine
- Text Editor
- Visual Recognition System
- Voxel Engine
- Web Browser
- Web Server
- Uncategorized
Tutorials
Build your own 3D Renderer
- C++: Introduction to Ray Tracing: a Simple Method for Creating 3D Images
- C++: How OpenGL works: software rendering in 500 lines of code
- C++: Raycasting engine of Wolfenstein 3D
- C++: Physically Based Rendering:From Theory To Implementation
- C++: Ray Tracing in One Weekend
- C++: Rasterization: a Practical Implementation
- C# / TypeScript / JavaScript: Learning how to write a 3D soft engine from scratch in C#, TypeScript or JavaScript
- Java / JavaScript: Build your own 3D renderer
- Java: How to create your own simple 3D render engine in pure Java
- JavaScript / Pseudocode: Computer Graphics from scratch
- Python: A 3D Modeller
Build your own Augmented Reality
- C#: How To: Augmented Reality App Tutorial for Beginners with Vuforia and Unity 3D [video]
- C#: How To Unity ARCore [video]
- C#: AR Portal Tutorial with Unity [video]
- C#: How to create a Dragon in Augmented Reality in Unity ARCore [video]
- C#: How to Augmented Reality AR Tutorial: ARKit Portal to the Upside Down [video]
- Python: Augmented Reality with Python and OpenCV
Build your own BitTorrent Client
- C#: Building a BitTorrent client from scratch in C#
- Go: Building a BitTorrent client from the ground up in Go
- Nim: Writing a Bencode Parser
- Node.js: Write your own bittorrent client
- Python: A BitTorrent client in Python 3.5
Build your own Blockchain / Cryptocurrency
- ATS: Functional Blockchain
- C#: Programming The Blockchain in C#
- Crystal: Write your own blockchain and PoW algorithm using Crystal
- Go: Building Blockchain in Go
- Go: Code your own blockchain in less than 200 lines of Go
- Java: Creating Your First Blockchain with Java
- JavaScript: A cryptocurrency implementation in less than 1500 lines of code
- JavaScript: Build your own Blockchain in JavaScript
- JavaScript: Learn & Build a JavaScript Blockchain
- JavaScript: Creating a blockchain with JavaScript
- JavaScript: How To Launch Your Own Production-Ready Cryptocurrency
- JavaScript: Writing a Blockchain in Node.js
- Kotlin: Letโs implement a cryptocurrency in Kotlin
- Python: Learn Blockchains by Building One
- Python: Build your own blockchain: a Python tutorial
- Python: A Practical Introduction to Blockchain with Python
- Python: Letโs Build the Tiniest Blockchain
- Ruby: Programming Blockchains Step-by-Step (Manuscripts Book Edition)
- Scala: How to build a simple actor-based blockchain
- TypeScript: Naivecoin: a tutorial for building a cryptocurrency
- TypeScript: NaivecoinStake: a tutorial for building a cryptocurrency with the Proof of Stake consensus
- Rust: Building A Blockchain in Rust & Substrate
Build your own Bot
- Haskell: Roll your own IRC bot
- Node.js: Creating a Simple Facebook Messenger AI Bot with API.ai in Node.js
- Node.js: How to make a responsive telegram bot
- Node.js: Create a Discord bot
- Node.js: gifbot - Building a GitHub App
- Node.js: Building A Simple AI Chatbot With Web Speech API And Node.js
- Python: How to Build Your First Slack Bot with Python
- Python: How to build a Slack Bot with Python using Slack Events API & Django under 20 minute
- Python: Build a Reddit Bot
- Python: How To Make A Reddit Bot [video]
- Python: How To Create a Telegram Bot Using Python
- Python: Create a Twitter Bot in Python Using Tweepy
- Python: Creating Reddit Bot with Python & PRAW [video]
- R: Build A Cryptocurrency Trading Bot with R
- Rust: A bot for Starcraft in Rust, C or any other language
Build your own Command-Line Tool
- Go: Visualize your local git contributions with Go
- Go: Build a command line app with Go: lolcat
- Go: Building a cli command with Go: cowsay
- Go: Go CLI tutorial: fortune clone
- Nim: Writing a stow alternative to manage dotfiles
- Node.js: Create a CLI tool in Javascript
- Rust: Command line apps in Rust
- Rust: Writing a Command Line Tool in Rust
- Zig: Build Your Own CLI App in Zig from Scratch
Build your own Database
- C: Let's Build a Simple Database
- C++: Build Your Own Redis from Scratch
- C#: Build Your Own Database
- Clojure: An Archaeology-Inspired Database
- Crystal: Why you should build your own NoSQL Database
- Go: Build Your Own Database from Scratch: Persistence, Indexing, Concurrency
- Go: Build Your Own Redis from Scratch
- JavaScript: Dagoba: an in-memory graph database
- Python: DBDB: Dog Bed Database
- Python: Write your own miniature Redis with Python
- Ruby: Build your own fast, persistent KV store in Ruby
- Rust: Build your own Redis client and server
Build your own Docker
- C: Linux containers in 500 lines of code
- Go: Build Your Own Container Using Less than 100 Lines of Go
- Go: Building a container from scratch in Go [video]
- Python: A workshop on Linux containers: Rebuild Docker from Scratch
- Python: A proof-of-concept imitation of Docker, written in 100% Python
- Shell: Docker implemented in around 100 lines of bash
Build your own Emulator / Virtual Machine
- C: Home-grown bytecode interpreters
- C: Virtual machine in C
- C: Write your Own Virtual Machine
- C: Writing a Game Boy emulator, Cinoop
- C++: How to write an emulator (CHIP-8 interpreter)
- C++: Emulation tutorial (CHIP-8 interpreter)
- C++: Emulation tutorial (GameBoy emulator)
- C++: Emulation tutorial (Master System emulator)
- C++: NES Emulator From Scratch [video]
- Common Lisp: CHIP-8 in Common Lisp
- JavaScript: GameBoy Emulation in JavaScript
- Python: Emulation Basics: Write your own Chip 8 Emulator/Interpreter
- Rust: 0dmg: Learning Rust by building a partial Game Boy emulator
Build your own Front-end Framework / Library
- JavaScript: WTF is JSX (Let's Build a JSX Renderer)
- JavaScript: A DIY guide to build your own React
- JavaScript: Building React From Scratch [video]
- JavaScript: Gooact: React in 160 lines of JavaScript
- JavaScript: Learn how React Reconciler package works by building your own lightweight React DOM
- JavaScript: Build Yourself a Redux
- JavaScript: Letโs Write Redux!
- JavaScript: Redux: Implementing Store from Scratch [video]
- JavaScript: Build Your own Simplified AngularJS in 200 Lines of JavaScript
- JavaScript: Make Your Own AngularJS
- JavaScript: How to write your own Virtual DOM
- JavaScript: Building a frontend framework, from scratch, with components (templating, state, VDOM)
- JavaScript: Build your own React
- JavaScript: Building a Custom React Renderer [video]
Build your own Game
- C: Handmade Hero
- C: How to Program an NES game in C
- C: Chess Engine In C [video]
- C: Let's Make: Dangerous Dave [video]
- C: Learn Video Game Programming in C [video]
- C: Coding A Sudoku Solver in C [video]
- C: Coding a Rogue/Nethack RPG in C [video]
- C: On Tetris and Reimplementation
- C++: Breakout
- C++: Beginning Game Programming v2.0
- C++: Tetris tutorial in C++ platform independent focused in game logic for beginners
- C++: Remaking Cavestory in C++ [video]
- C++: Reconstructing Cave Story [video]
- C++: Space Invaders from Scratch
- C#: Learn C# by Building a Simple RPG
- C#: Creating a Roguelike Game in C#
- C#: Build a C#/WPF RPG
- Go: Games With Go [video]
- Java: Code a 2D Game Engine using Java - Full Course for Beginners [video]
- Java: 3D Game Development with LWJGL 3
- JavaScript: 2D breakout game using Phaser
- JavaScript: How to Make Flappy Bird in HTML5 With Phaser
- JavaScript: Developing Games with React, Redux, and SVG
- JavaScript: Build your own 8-Ball Pool game from scratch [video]
- JavaScript: How to Make Your First Roguelike
- JavaScript: Think like a programmer: How to build Snake using only JavaScript, HTML & CSS
- Lua: BYTEPATH
- Python: Developing Games With PyGame
- Python: Making Games with Python & Pygame [pdf]
- Python: Roguelike Tutorial Revised
- Ruby: Developing Games With Ruby
- Ruby: Ruby Snake
- Rust: Adventures in Rust: A Basic 2D Game
- Rust: Roguelike Tutorial in Rust + tcod
Build your own Git
- Haskell: Reimplementing โgit cloneโ in Haskell from the bottom up
- JavaScript: Gitlet
- JavaScript: Build GIT - Learn GIT
- Python: Just enough of a Git client to create a repo, commit, and push itself to GitHub
- Python: Write yourself a Git!
- Python: ugit: Learn Git Internals by Building Git Yourself
- Ruby: Rebuilding Git in Ruby
Build your own Network Stack
- C: Beej's Guide to Network Programming
- C: Let's code a TCP/IP stack
- C / Python: Build your own VPN/Virtual Switch
- Ruby: How to build a network stack in Ruby
Build your own Neural Network
- C#: Neural Network OCR
- F#: Building Neural Networks in F#
- Go: Build a multilayer perceptron with Golang
- Go: How to build a simple artificial neural network with Go
- Go: Building a Neural Net from Scratch in Go
- JavaScript / Java: Neural Networks - The Nature of Code [video]
- JavaScript: Neural networks from scratch for JavaScript linguists (Part1โโโThe Perceptron)
- Python: A Neural Network in 11 lines of Python
- Python: Implement a Neural Network from Scratch
- Python: Optical Character Recognition (OCR)
- Python: Traffic signs classification with a convolutional network
- Python: Generate Music using LSTM Neural Network in Keras
- Python: An Introduction to Convolutional Neural Networks
- Python: Neural Networks: Zero to Hero
Build your own Operating System
- Assembly: Writing a Tiny x86 Bootloader
- Assembly: Baking Pi โ Operating Systems Development
- C: Building a software and hardware stack for a simple computer from scratch [video]
- C: Operating Systems: From 0 to 1
- C: The little book about OS development
- C: Roll your own toy UNIX-clone OS
- C: Kernel 101 โ Letโs write a Kernel
- C: Kernel 201 โ Letโs write a Kernel with keyboard and screen support
- C: Build a minimal multi-tasking kernel for ARM from scratch
- C: How to create an OS from scratch
- C: Malloc tutorial
- C: Hack the virtual memory
- C: Learning operating system development using Linux kernel and Raspberry Pi
- C: Operating systems development for Dummies
- C++: Write your own Operating System [video]
- C++: Writing a Bootloader
- Rust: Writing an OS in Rust
- Rust: Add RISC-V Rust Operating System Tutorial
- (any): Linux from scratch
Build your own Physics Engine
- C: Video Game Physics Tutorial
- C++: Game physics series by Allen Chou
- C++: How to Create a Custom Physics Engine
- C++: 3D Physics Engine Tutorial [video]
- JavaScript: How Physics Engines Work
- JavaScript: Broad Phase Collision Detection Using Spatial Partitioning
- JavaScript: Build a simple 2D physics engine for JavaScript games
Build your own Programming Language
- (any): mal - Make a Lisp
- Assembly: Jonesforth
- C: Baby's First Garbage Collector
- C: Build Your Own Lisp: Learn C and build your own programming language in 1000 lines of code
- C: Writing a Simple Garbage Collector in C
- C: C interpreter that interprets itself.
- C: A C & x86 version of the "Let's Build a Compiler" by Jack Crenshaw
- C: A journey explaining how to build a compiler from scratch
- C++: Writing Your Own Toy Compiler Using Flex
- C++: How to Create a Compiler [video]
- C++: Kaleidoscope: Implementing a Language with LLVM
- F#: Understanding Parser Combinators
- Elixir: Demystifying compilers by writing your own [video]
- Go: The Super Tiny Compiler
- Go: Lexical Scanning in Go [video]
- Haskell: Let's Build a Compiler
- Haskell: Write You a Haskell
- Haskell: Write Yourself a Scheme in 48 Hours
- Haskell: Write You A Scheme
- Java: Crafting interpreters: A handbook for making programming languages
- Java: Creating JVM Language
- JavaScript: The Super Tiny Compiler
- JavaScript: The Super Tiny Interpreter
- JavaScript: Little Lisp interpreter
- JavaScript: How to implement a programming language in JavaScript
- JavaScript: Letโs go write a Lisp
- OCaml: Writing a C Compiler
- OCaml: Writing a Lisp, the series
- Pascal: Let's Build a Compiler
- Python: A Python Interpreter Written in Python
- Python: lisp.py: Make your own Lisp interpreter
- Python: How to Write a Lisp Interpreter in Python
- Python: Letโs Build A Simple Interpreter
- Python: Make Your Own Simple Interpreted Programming Language [video]
- Python: From Source Code To Machine Code: Build Your Own Compiler From Scratch
- Racket: Beautiful Racket: How to make your own programming languages with Racket
- Ruby: A Compiler From Scratch
- Ruby: Markdown compiler from scratch in Ruby
- Rust: So You Want to Build a Language VM
- Rust: Learning Parser Combinators With Rust
- Swift: Building a LISP from scratch with Swift
- TypeScript: Build your own WebAssembly Compiler
Build your own Regex Engine
- C: A Regular Expression Matcher
- C: Regular Expression Matching Can Be Simple And Fast
- Go: How to build a regex engine from scratch
- JavaScript: Build a Regex Engine in Less than 40 Lines of Code
- JavaScript: How to implement regular expressions in functional javascript using derivatives
- JavaScript: Implementing a Regular Expression Engine
- Perl: How Regexes Work
- Python: Build Your Own Regular Expression Engines: Backtracking, NFA, DFA
- Scala: No Magic: Regular Expressions
Build your own Search Engine
- CSS: A search engine in CSS
- Python: Building a search engine using Redis and redis-py
- Python: Building a Vector Space Indexing Engine in Python
- Python: Building A Python-Based Search Engine [video]
- Python: Making text search learn from feedback
- Python: Finding Important Words in Text Using TF-IDF
Build your own Shell
- C: Tutorial - Write a Shell in C
- C: Let's build a shell!
- C: Writing a UNIX Shell
- C: Build Your Own Shell
- C: Write a shell in C
- Go: Writing a simple shell in Go
- Rust: Build Your Own Shell using Rust
Build your own Template Engine
- JavaScript: JavaScript template engine in just 20 lines
- JavaScript: Understanding JavaScript Micro-Templating
- Python: Approach: Building a toy template engine in Python
- Python: A Template Engine
- Ruby: How to write a template engine in less than 30 lines of code
Build your own Text Editor
- C: Build Your Own Text Editor
- C++: Designing a Simple Text Editor
- Python: Python Tutorial: Make Your Own Text Editor [video]
- Python: Create a Simple Python Text Editor!
- Ruby: Build a Collaborative Text Editor Using Rails
- Rust: Hecto: Build your own text editor in Rust
Build your own Visual Recognition System
- Python: Developing a License Plate Recognition System with Machine Learning in Python
- Python: Building a Facial Recognition Pipeline with Deep Learning in Tensorflow
Build your own Voxel Engine
Build your own Web Browser
Build your own Web Server
- C#: Writing a Web Server from Scratch
- Node.js: Build Your Own Web Server From Scratch In JavaScript
- Node.js: Let's code a web server from scratch with NodeJS Streams
- Node.js: lets-build-express
- PHP: Writing a webserver in pure PHP
- Python: A Simple Web Server
- Python: Letโs Build A Web Server.
- Python: Web application from scratch
- Python: Building a basic HTTP Server from scratch in Python
- Python: Implementing a RESTful Web API with Python & Flask
- Ruby: Building a simple websockets server from scratch in Ruby
Uncategorized
- (any): From NAND to Tetris: Building a Modern Computer From First Principles
- Alloy: The Same-Origin Policy
- C: How to Write a Video Player in Less Than 1000 Lines
- C: Learn how to write a hash table in C
- C: The very basics of a terminal emulator
- C: Write a System Call
- C: Sol - An MQTT broker from scratch
- C++: Build your own VR headset for $200
- C++: How X Window Managers work and how to write one
- C++: Writing a Linux Debugger
- C++: How a 64k intro is made
- C++: Make your own Game Engine
- C#: C# Networking: Create a TCP chater server, TCP games, UDP Pong and more
- C#: Loading and rendering 3D skeletal animations from scratch in C# and GLSL
- Clojure: Building a spell-checker
- Go: Build A Simple Terminal Emulator In 100 Lines of Golang
- Go: Let's Create a Simple Load Balancer
- Go: Video Encoding from Scratch
- Java: How to Build an Android Reddit App [video]
- JavaScript: Build Your Own Module Bundler - Minipack
- JavaScript: Learn JavaScript Promises by Building a Promise from Scratch
- JavaScript: Implementing promises from scratch (TDD way)
- JavaScript: Implement your ownโโโcall(), apply() and bind() method in JavaScript
- JavaScript: JavaScript Algorithms and Data Structures
- JavaScript: Build a ride hailing app with React Native
- JavaScript: Build Your Own AdBlocker in (Literally) 10 Minutes
- Kotlin: Build Your Own Cache
- Lua: Building a CDN from Scratch to Learn about CDN
- Nim: Writing a Redis Protocol Parser
- Nim: Writing a Build system
- Nim: Writing a MiniTest Framework
- Nim: Writing a DMIDecode Parser
- Nim: Writing a INI Parser
- Nim: Writing a Link Checker
- Nim: Writing a URL Shortening Service
- Node.js: Build a static site generator in 40 lines with Node.js
- Node.js: Building A Simple Single Sign On(SSO) Server And Solution From Scratch In Node.js.
- Node.js: How to create a real-world Node CLI app with Node
- Node.js: Build a DNS Server in Node.js
- PHP: Write your own MVC from scratch in PHP
- PHP: Make your own blog
- PHP: Modern PHP Without a Framework
- PHP: Code a Web Search Engine in PHP
- Python: Build a Deep Learning Library [video]
- Python: How to Build a Kick-Ass Mobile Document Scanner in Just 5 Minutes
- Python: Continuous Integration System
- Python: Recommender Systems in Python: Beginner Tutorial
- Python: Write SMS-spam detector with Scikit-learn
- Python: A Simple Content-Based Recommendation Engine in Python
- Python: Stock Market Predictions with LSTM in Python
- Python: Building a simple Generative Adversarial Network (GAN) using Tensorflow
- Python: Learn ML Algorithms by coding: Decision Trees
- Python: JSON Decoding Algorithm
- Python: Build your own Git plugin with python
- Ruby: A Pedometer in the Real World
- Ruby: Creating a Linux Desktop application with Ruby
- Rust: Building a DNS server in Rust
- Rust: Writing Scalable Chat Service from Scratch
- Rust: WebGL + Rust: Basic Water Tutorial
- TypeScript: Tiny Package Manager: Learns how npm or Yarn works
Contribute
- Submissions welcome, just send a PR, or create an issue
- Help us review pending submissions by leaving comments and "reactions"
Origins & License
This repository is the work of many contributors. It was started by Daniel Stefanovic, and is now maintained by CodeCrafters, Inc. To the extent possible under law, CodeCrafters, Inc. has waived all copyright and related or neighboring rights to this work.
A list of useful payloads and bypass for Web Application Security and Pentest/CTF
Payloads All The Things
A list of useful payloads and bypasses for Web Application Security. Feel free to improve with your payloads and techniques ! I โค๏ธ pull requests :)
You can also contribute with a ๐ป IRL, or using the sponsor button
An alternative display version is available at PayloadsAllTheThingsWeb.
๐ Documentation
Every section contains the following files, you can use the _template_vuln
folder to create a new chapter:
- README.md - vulnerability description and how to exploit it, including several payloads
- Intruder - a set of files to give to Burp Intruder
- Images - pictures for the README.md
- Files - some files referenced in the README.md
You might also like the other projects from the AllTheThings family :
- InternalAllTheThings - Active Directory and Internal Pentest Cheatsheets
- HardwareAllTheThings - Hardware/IOT Pentesting Wiki
You want more ? Check the Books and Youtube channel selections.
๐ง๐ป Contributions
Be sure to read CONTRIBUTING.md
Thanks again for your contribution! โค๏ธ
๐ป Sponsors
This project is proudly sponsored by these companies:
12 Weeks, 24 Lessons, AI for All!
Artificial Intelligence for Beginners - A Curriculum
![]() |
---|
AI For Beginners - Sketchnote by @girlie_mac |
Explore the world of Artificial Intelligence (AI) with our 12-week, 24-lesson curriculum! It includes practical lessons, quizzes, and labs. The curriculum is beginner-friendly and covers tools like TensorFlow and PyTorch, as well as ethics in AI
What you will learn
In this curriculum, you will learn:
- Different approaches to Artificial Intelligence, including the "good old" symbolic approach with Knowledge Representation and reasoning (GOFAI).
- Neural Networks and Deep Learning, which are at the core of modern AI. We will illustrate the concepts behind these important topics using code in two of the most popular frameworks - TensorFlow and PyTorch.
- Neural Architectures for working with images and text. We will cover recent models but may be a bit lacking in the state-of-the-art.
- Less popular AI approaches, such as Genetic Algorithms and Multi-Agent Systems.
What we will not cover in this curriculum:
Find all additional resources for this course in our Microsoft Learn collection
- Business cases of using AI in Business. Consider taking Introduction to AI for business users learning path on Microsoft Learn, or AI Business School, developed in cooperation with INSEAD.
- Classic Machine Learning, which is well described in our Machine Learning for Beginners Curriculum.
- Practical AI applications built using Cognitive Services. For this, we recommend that you start with modules Microsoft Learn for vision, natural language processing, Generative AI with Azure OpenAI Service and others.
- Specific ML Cloud Frameworks, such as Azure Machine Learning, Microsoft Fabric, or Azure Databricks. Consider using Build and operate machine learning solutions with Azure Machine Learning and Build and Operate Machine Learning Solutions with Azure Databricks learning paths.
- Conversational AI and Chat Bots. There is a separate Create conversational AI solutions learning path, and you can also refer to this blog post for more detail.
- Deep Mathematics behind deep learning. For this, we would recommend Deep Learning by Ian Goodfellow, Yoshua Bengio and Aaron Courville, which is also available online at https://www.deeplearningbook.org/.
For a gentle introduction to AI in the Cloud topics you may consider taking the Get started with artificial intelligence on Azure Learning Path.
Content
Each lesson contains
- Pre-reading material
- Executable Jupyter Notebooks, which are often specific to the framework (PyTorch or TensorFlow). The executable notebook also contains a lot of theoretical material, so to understand the topic you need to go through at least one version of the notebook (either PyTorch or TensorFlow).
- Labs available for some topics, which give you an opportunity to try applying the material you have learned to a specific problem.
- Some sections contain links to MS Learn modules that cover related topics.
Getting Started
- We have created a setup lesson to help you with setting up your development environment. - For Educators, we have created a curricula setup lesson for you too!
- How to Run the code in a VSCode or a Codepace
Follow these steps:
Fork the Repository: Click on the "Fork" button at the top-right corner of this page.
Clone the Repository: git clone https://github.com/microsoft/AI-For-Beginners.git
Don't forget to star (๐) this repo to find it easier later.
Meet other Learners
Join our official AI Discord server to meet and network with other learners taking this course and get support.
If you have product feedback or questions whilst building visit our Azure AI Foundry Developer Forum
Quizzes
A note about quizzes: All quizzes are contained in the Quiz-app folder in etc\quiz-app, They are linked from within the lessons the quiz app can be run locally or deployed to Azure; follow the instruction in the
quiz-app
folder. They are gradually being localized.
Help Wanted
Do you have suggestions or found spelling or code errors? Raise an issue or create a pull request.
Special Thanks
- โ๏ธ Primary Author: Dmitry Soshnikov, PhD
- ๐ฅ Editor: Jen Looper, PhD
- ๐จ Sketchnote illustrator: Tomomi Imura
- โ Quiz Creator: Lateefah Bello, MLSA
- ๐ Core Contributors: Evgenii Pishchik
Other Curricula
Our team produces other curricula! Check out:
- Generative AI for Beginners
- Generative AI for Beginners .NET
- Generative AI with JavaScript
- AI for Beginners
- Data Science for Beginners
- ML for Beginners
- Cybersecurity for Beginners
- Web Dev for Beginners
- IoT for Beginners
- XR Development for Beginners
- Mastering GitHub Copilot for Paired Programming
- Mastering GitHub Copilot for C#/.NET Developers
- Choose Your Own Copilot Adventure